Research Projects in 2025
Mentors named for the 2025 SIAM-Simons Undergraduate Summer Research Program
Congratulations to the following five members of the SIAM community who will be mentors for the 2025 SIAM-Simons Undergraduate Summer Research Program.
- Joshua Hiller, Adelphi University
- Veronica Ciocanel, Duke University
- Iván Ojeda-Ruiz, Texas State University
- Anastasiiia Minenkova, University of Hartford
- David Elzinga, University of Wisconsin – La Crosse
Additional information about each mentor and the research project they proposed can be found below.
Each year, program establishes five sites across the United States for a program of research and learning in applied mathematics and computational science. One faculty mentor and two students at each site will work together as participants learn how to conduct scientific research, effectively communicate mathematics and computational science principles, and gain an improved understanding of how they can pursue a career in applied mathematics and computational science. Students and mentors from the five sites will come together via video conference to present their work, participate in professional development activities, and engage in community-building initiatives to bring all participants together and foster a strong sense of belonging.
The application for undergraduate participants is open. If you are interested in becoming a mentor for the summer 2026 cohort, the application will open in summer 2025.
This program targets U.S. students from groups underrepresented in applied mathematics and computational mathematics in the U.S., specifically ethnic minorities (African American/Black, Hispanic, Native American/Indigenous Peoples, Native Alaskan, Native Hawaiian/Other Pacific Islander). The program is intended to broaden participation in mathematics by students who are underrepresented and historically marginalized in our discipline.
Adelphi University, Garden City, New York
Effectiveness of Market-Based Deforestation Prevention Strategies: Modeling the Effect of Fluctuating Commodity Prices and Industrial
The emergence of tools that help decision-makers face deforestation pressures lags behind the rate at which natural forests are lost to industrial agriculture. Critical ecosystems such as the South American Cerrado and Chaco forests or peat swamp forests in South-East Asia are being lost at alarming rates to industrial production of soybean, pasture for cattle, and oil palm, despite unprecedented attempts from local and international organizations to temper forest loss. In this project we will investigate a simple two-state Markov model of deforestation that explicitly tests potential market-based strategies to halt deforestation in a context of fluctuating commodity prices of industrial agriculture. The project will draw on concepts from probability, statistics, economics, and data analysis. We will validate our model using historical data from the Gran Chaco region of Argentina in order to examine the effectiveness of Argentina’s Law of the Forest (one of the largest payment for ecosystems services programs in the world). In this project students will interact both with their mentor at Adelphi and with industry partners via weekly meetings with the Gran Chaco Lead at The Nature Conservancy.
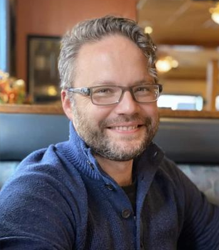
Mentor: Joshua Hiller is Associate Professor of Mathematics and Computer Science at Adelphi University. His research straddles the line between theoretical computer science and applied mathematics, and uses tools from probability, graph theory, algebra, and enumerative combinatorics. He is passionate about the use of undergraduate research experiences as a retention tool for students from under-represented communities. He received his PhD from the
Duke University, Durham, North Carolina
Inference and Mathematical Modeling of mRNA Transport in Neuronal Cells
How the brain is assembled during embryonic development is a key question that would help us understand many neurodevelopmental disorders. Experiments have shown that the movement of proteins inside neurons has a large impact on brain development and function. In particular, a specific type of protein (called messenger RNA or mRNA) is needed in various locations within neuronal cells, and its transport to these locations is very impactful in development. This is often difficult to fully understand experimentally, so we partner with a medical lab to quantify and mathematically model the protein movement. In this project, we will extract information about mRNA transport from experimental videos using computational and machine learning techniques. We will then use these measurements to build and predict mathematical models of the messenger RNA dynamics in neural cells using techniques drawing from Bayesian analysis, stochastic processes, and differential equations.
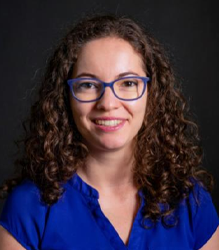
Mentor: Veronica Ciocanel received her B.S. in Mathematics from Duke University and her M.S. and PhD in Applied Mathematics from Brown University. She completed her postdoctoral training at the Mathematical Biosciences Institute at The Ohio State University, where she held a President's Postdoctoral Scholar fellowship. Dr. Ciocanel is currently an Assistant Professor of Mathematics, with a joint appointment in Biology, at Duke University. She has served as the Chair of the Cell and Developmental Biology subgroup of the Society of Mathematical Biology (SMB). She currently co-chairs the DEI team of Duke Mathematics. Dr. Ciocanel’s group studies questions in cell and developmental biology using dynamical systems, stochastic processes, and numerical simulations.
Texas State University, San Marcos, Texas
Clustering Methods with Fairness
This project delves into the crucial intersection of fairness and mathematical models, particularly clustering algorithms. Early research established the concept of disparate impact and introduced quantitative fairness metrics, paving the way for integrating fairness into data analysis. Clustering, while powerful, can inadvertently amplify biases, necessitating the incorporation of fairness measures.
Our project builds upon this foundation by reformulating existing fairness metrics into a linearly optimizable form, facilitating their integration into clustering algorithms. We explore social fairness within the k-means algorithm and the role of fair Principal Component Analysis in ensuring equitable data analysis. Furthermore, inspired by our prior work linking the Algebraic Multigrid Method and Spectral Clustering, we are developing a novel approach to fair clustering by imposing fairness constraints on the optimization problems. This innovative method highlights the potential of interdisciplinary collaboration in promoting fairness in AI.
By combining theoretical advancements with practical applications, we aim to contribute to the development of more equitable and responsible AI systems. This project represents a significant step towards embedding fairness considerations into the core of mathematical models and algorithms, ultimately striving for a more just and equitable world.
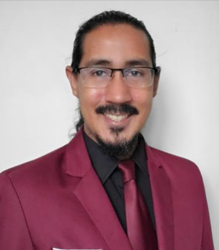
Mentor: Iván Ojeda-Ruiz is an associate professor of instruction in the Department of Mathematics at Texas State University. He earned a bachelor’s degree with a double major in mathematics and chemistry from the University of Puerto Rico, Rio Piedras, and completed his Ph.D. at the University of Texas at Arlington in 2017, where he served as president of the SIAM student chapter. His research interests include image segmentation, clustering methods, and machine learning fairness.
Dr. Ojeda-Ruiz has been an active research mentor for many undergraduate students, which led to the creation of a SIAM student chapter at Texas State University in the fall of 2023. He has been a dedicated SIAM member for 13 years and is currently an MGB-SIAM Early Career Fellow and a SIAM Science Policy Fellow.
University of Hartford, Hartford, Connecticut
Inverse Problems in Linear Algebra and the Perfect Quantum State
The goal of this project is to dive into the fascinating world of symmetries and explore how they help us understand different sets of graphs by means of linear algebra. Using information about eigenvalues and matrix structures, we will rebuild a class of graphs, representing the spin chains. Finally, we will explore what quantum computing is and how the structure of these matrices relates to achieving the perfect quantum state transfer. Join us on this journey to uncover the mysteries of quantum spin chains and their symmetries!
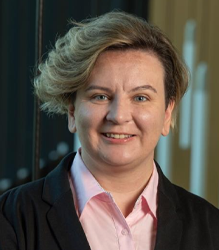
Mentor: Anastasiia Minenkova joined the Mount Holyoke College as a Visiting Lecturer in 2022 for a year and since Fall 2023, she is an assistant professor at the University of Hartford. She got her PhD from the University of Connecticut and now works in applied mathematics on the intersection of numerical analysis and perturbation theory. She is also interested in applications of numerical linear algebra to quantum computing. She has experience working with undergraduate students on research projects. She strives to promote equity and inclusion in math through her mentoring, teaching and service.
University of Wisconsin - La Crosse, La Crosse, Wisconsin
Using Dynamical Systems to Shape Management Decisions for Beavers and Cottonwood Trees in the Upper Mississippi River Region
Managing fragile ecosystems is a challenging task. The Upper Mississippi River (UMR) is a fragile ecosystem, whose modern management is critical to ecological health. Cottonwood trees serve as keystone species in the UMR by improving water quality, filtering air pollution, and providing nesting sites for birds. Recent declines in cottonwood tree reproduction are therefore of notable concern. Changes in the flooding patterns of the Mississippi River, the restoration of the American beaver, and habitat loss all put pressure on maintaining cottonwood trees. This project aims to develop a mathematical model that simulates the interactions between cottonwood trees, beavers, and the river. The model will be used both to forecast the future of cottonwood populations without intervention and to inform effective management strategies for sustaining these crucial trees.
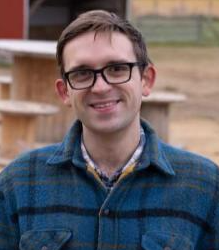
Mentor: David Elzinga is an Assistant Professor of Statistics at the University of Wisconsin-La Crosse. He holds a Ph.D. in Mathematical Biology and an M.S. in Statistics from the University of Tennessee. His research centers on using data-driven methods in mathematics, statistics, and data science to address natural resource management problems.