2025 February Prize Spotlight
Congratulations to the following SIAM prize recipients who will be recognized at the 2025 SIAM Conference on Computational Science and Engineering (CSE25), taking place March 3-7, 2025 in Fort Worth, Texas.
- Daniel Arndt, et al.—SIAM/ACM Prize in Computational Science and Engineering
- Haim Avron, Lior Horesh, Misha Kilmer, and Elizabeth Newman—SIAM Activity Group on Computational Science and Engineering Best Paper Prize
- Omar Ghattas—Ivo & Renata Babuška Prize
- Jiequn Han—SIAM Activity Group on Computational Science and Engineering Early Career Prize
- Erin Carson—Wilkinson Prize in Numerical Analysis and Scientific Computing
Daniel Arndt, et al.
The Principal Authors of the deal.II project are the recipients of the 2025 SIAM/ACM Prize in Computational Science and Engineering. The team received the award for their “highly impactful library supporting finite element calculations. The software achieved a very high standard of software quality and modularity by providing packaged algorithms and data structures in a comprehensive and well-documented manner that enables and elevates whole communities to achieve more computationally advanced models than they could possibly otherwise.” The team includes: Daniel Arndt, Oak Ridge National Laboratory; Wolfgang Bangerth, Colorado State University; Bruno Blais, Polytechnique Montréal; Marc Fehling, Charles University; Rene Gassmoeller, GEOMAR Helmholtz Centre for Ocean Research Kiel; Timo Heister, Clemson University, Luca Heltai, University of Pisa; Guido Kanschat, Heidelberg University; Martin Kronbichler, Ruhr University Bochum; Matthias Maier, Texas A&M University; Peter Munch, Technical University of Berlin; Jean-Paul Pelteret, independent researcher; Bruno Turcksin, Oak Ridge National Laboratory; and David Wells, University of North Carolina, Chapel Hill.
Dr. Wolfgang Bangerth will deliver the prize lecture on behalf of the team at the 2025 SIAM Conference on Computational Science and Engineering (CSE25) on Monday, March 3 at 5:35 p.m. CST.
The SIAM/ACM Prize in Computational Science and Engineering is awarded every two years by SIAM and the Association for Computing Machinery (ACM) in the area of computational science to one individual or a group of individuals in recognition of outstanding contributions to the development and use of mathematical and computational tools and methods for the solution of science and engineering problems.
Dr. Daniel Arndt is a computational scientist at Oak Ridge National Laboratory. He previously held postdoctoral research positions at Oak Ridge National Laboratory and Heidelberg University, Germany. He earned his Ph.D. in mathematics from the University of Göttingen, Germany (2016) and became a deal.II principal developer the same year. Dr. Arndt's research revolves around performance portability.
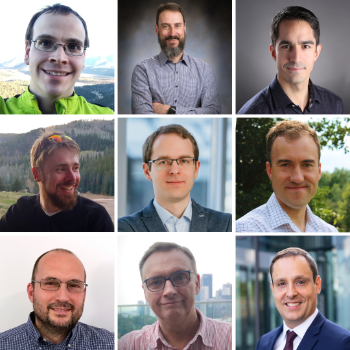
He is a key developer of the Kokkos library, which enables algorithms with a single source code to be mapped to diverse target architectures, applying architecture-specific optimizations for optimal performance. He also contributes to various other projects based on Kokkos using finite element simulations. Learn more about Dr. Arndt.
Dr. Wolfgang Bangerth is a professor of mathematics and, by courtesy, geosciences at Colorado State University. He was previously a professor at Texas A&M University (2005-2016), and a postdoc at the University of Texas at Austin. He received his Ph.D. in mathematics from Heidelberg University, Germany (2002). His research is on the development of widely usable finite element methods, their algorithmic implementation in open-source software, and applications of computational methods throughout the sciences and engineering.
Driven by a passion for applying mathematical and computational techniques beyond mathematics, Dr. Bangerth has collaborated with scientists across diverse disciplines, from astrophysics to zoology. His work has been published in a wide range of journals, and he has received his university’s 2024 Interdisciplinary Scholarship Award. A key example of his commitment to making computational tools widely accessible is the deal.II project, an open-source software library for finite element solutions of partial differential equations, which he co-founded and continues to serve as a principal developer. For his contributions to deal.ll, he received the 2007 James H. Wilkinson Prize for Numerical Software. Learn more about Dr. Bangerth.
Dr. Bruno Blais is an associate professor of chemical engineering at Polytechnique Montréal, Canada. He was previously a research officer at the National Research Council Canada (2016-18). He received his Ph.D. in chemical engineering from Polytechnique Montréal (2016). His research is on the development, verification and validation of high-performance open-source simulation software to simulate multiphase flows encountered in chemical and manufacturing processes.
Dr. Blais aims at playing a key role in facilitating the energy transition of the chemical industry by accelerating the development of intensified chemical processes that leverage electricity. This is achieved in his research by development of open-source finite-element based simulation models that accurately reproduce the complex reacting multiphase flows that occur in chemical reactors. He is one of the principal developers of the deal.II project and a staunch advocate of open-source software and open science. Learn more about Dr. Blais.
Dr. Marc Fehling has been a postdoctoral fellow in the mathematical analysis department of Charles University, Czech Republic since 2024. Previously, he was a postdoctoral fellow in the mathematics department at Colorado State University (2020-24). He received his Ph.D. in civil engineering from the University of Wuppertal, Germany in collaboration with the Jülich Research Centre, Germany (2020).
Dr. Fehling is an applied mathematician and a computational scientist. He became a principal developer of the deal.II library in 2021 after significant contributions to the parallelization of the hp-adaptive finite element implementation and the efficient solution of the resulting systems of equations. Learn more about Dr. Fehling.
Dr. Rene Gassmoeller has been a staff scientist at the GEOMAR Helmholtz Centre for Ocean Research Kiel, Germany, since 2024. He was previously a research scientist at the University of Florida, a project scientist at University of California, Davis, and a postdoctoral researcher at Colorado State University and Texas A&M University. He received his Ph.D. in geophysics from the University of Potsdam, Germany, in collaboration with the GFZ Helmholtz Centre for Geosciences (2015).
Dr. Gassmoeller’s interests lie in applying modern numerical methods to solve geoscientific research problems and establishing and disseminating better scientific software standards in the geosciences. As a result of this ongoing effort, he was awarded the Better Scientific Software Fellowship by the IDEAS project, part of the Department of Energy-funded Exascale Computing Project (2019). He also acted as technical lead for the Computational Infrastructure in Geodynamics, a community-driven organization that provides infrastructure for the development and dissemination of software for geophysics and related fields. Besides his work as a principal developer for the deal.II library, he is also one of the maintainers of the deal.II-based geodynamic modeling software ASPECT, which he uses to investigate the interactions between Earth’s mantle and the surface, and the long-term dynamic behavior of the Earth’s mantle. Learn more about Dr. Gassmoeller.
Dr. Timo Heister is a professor in the School of Mathematical and Statistical Sciences at Clemson University. He received his Ph.D. in mathematics from the University of Göttingen, Germany and joined Texas A&M University as a visiting assistant professor (2011-13). He then became an assistant professor at Clemson University (2013). He is an applied mathematician and a computational scientist. His research centers around numerical analysis and the numerical solution of partial differential equations using the finite element method focusing on efficient solvers that scale to massively parallel supercomputers.
As one of the principal developers of the open source deal.II library that supports the creation of finite element codes, Dr. Heister’s work is used to develop new numerical methods and solve problems across a wide range of scientific disciplines. Dr. Heister is involved in various other open-source projects used in geosciences (e.g., the ASPECT geodynamic modeling code) and in solid mechanics. Learn more about Dr. Heister.
Dr. Luca Heltai has been a professor of numerical analysis in the mathematics department of the University of Pisa, Italy, since 2023. He was previously a professor at the International School for Advanced Studies, Trieste, Italy, and a research associate at Penn State College of Earth and Mineral Sciences, State College. He obtained his Ph.D. in mathematics and scientific computing from the University of Pavia (2006). He was a Fulbright scholar at the Courant Institute of Mathematical Sciences in New York, and a visiting faculty member at the Ecole Polytechnique de Paris, Universitat Polytechnica de Catalunia, Weierstrass Institute for Applied Analysis and Stochastics, and the King Abdullah University of Science and Technology.
Previously, Dr. Heltai served as the director and co-founder of the joint SISSA-ICTP Master's program in high performance computing (2014-22) and contributed to the design and establishment of the master’s degree in data science and scientific computing offered jointly by SISSA, ICTP, University of Trieste, and the University of Udine. Dr. Heltai is an expert in the development of numerical methods and algorithms for the solution of biological, physiological, and industrial problems modeled by partial differential equations. He also is one of the principal developers of the open source library deal.II for the high performance computing solution of partial differential equations. Learn more about Dr. Heltai.
Dr. Guido Kanschat received his Ph.D. and habilitation from the Heidelberg University in 1996 and 2004, respectively. He also was a faculty member at Texas A&M University (2006-2012). Since then, he has been professor at Heidelberg University, and recently became the founding Dean of the Faculty of Engineering Sciences (2020).
In his research, he studies the analysis and implementation of finite element methods. In 1992, he co-founded the DEAL project leading to the deal.II finite element library, which he co-maintained for more than 20 years since 1998. Mathematically, his research focuses on discontinuous Galerkin methods with applications to coupled flow problems and radiative transfer as well as compatible finite elements for cochain complexes. He also works on the efficient implementation of multigrid methods on modern hardware.
Dr. Martin Kronbichler is a professor of applied numerics at Ruhr University Bochum, Germany. He was previously a professor at the University of Augsburg, Germany, an associate professor at Uppsala University, Sweden, as well as a post-doctoral researcher and senior researcher at Technical University of Munich, Germany. He obtained his Ph.D. in scientific computing with specialization in numerical analysis from Uppsala University (2012).
Dr. Kronbichler’s main research interests are high-performance computing aspects of finite element algorithms and their use in sophisticated applications in computational mechanics. He has been contributing to a wide range of topics to the widely used open-source library deal.II, including MPI parallelization, SIMD vectorization, and GPU algorithms for higher-order methods with matrix-free methods, multigrid methods, and unfitted finite element methods. With his research, Dr. Kronbichler has been able to make huge leaps in the application performance of modern mathematical schemes, such as mesh adaptivity, conforming and non-conforming finite element methods, delivering costs per unknown near classical low-order finite difference methods, at much higher flexibility and accuracy. Learn more about Dr. Kronbichler.
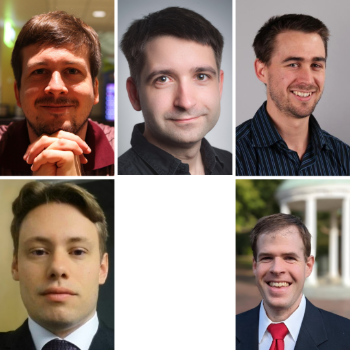
Dr. Matthias Maier is an associate professor in the mathematics department of Texas A&M University. He obtained his master's degrees in mathematics and physics from Heidelberg University, Germany, as well as his doctorate in mathematics (2015). He was a Dunham Jackson Assistant Professor at the University of Minnesota (201-18). He joined the Texas A&M faculty in 2018 before being promoted to associate professor (2022).
Dr. Maier's research centers around scientific computation and analysis of numerical methods for optics and fluid dynamics with an emphasis on simulating multiscale phenomena. The nature of the problems he investigates makes his research collaborations highly interdisciplinary. They range from mathematical topics in applied and numerical analysis and scientific computing, to computer science and software engineering, to theoretical physics and engineering. Learn more about Dr. Maier.
Dr. Peter Munch is a postdoctoral fellow at the Technical University of Berlin, Germany (TU Berlin). He received his doctoral degree from the Institute of Mathematics of the University of Augsburg (2023) under the supervision of Professor Martin Kronbichler. Before joining TU Berlin, he was a postdoctoral researcher in the Division of Scientific Computing and the Uppsala Architecture Research Team at Uppsala University, Sweden.
Dr. Munch’s research interests lie in the fields of high-performance computing, scientific software development, and development of iterative solvers—with a particular focus on computational fluid mechanics, additive manufacturing, immersed and non-conforming methods, space-time finite element methods, and multigrid methods for locally refined meshes. His most significant contribution to deal.II is its simplex and mixed mesh support. Learn more about Dr. Munch.
Dr. Jean-Paul Pelteret received his Ph.D. in mechanical engineering from the University of Cape Town (2013), under the guidance of Professor Daya Reddy. Afterwards, he took on a post-doctoral research role at the Friedrich-Alexander-Universität Erlangen-Nürnberg. He has since moved into the commercial sector, currently contributing to the development of high-performance multi-physics software.
Dr. Pelteret’s primary research interests span nonlinear solid mechanics, biomechanics, electromagnetics, and coupled material modelling. He became a principal developer of the deal.II finite element library in 2016. Being interested in community development and making computational simulations more accessible and more easily verifiable, he developed generic frameworks to incorporate established automatic differentiation and symbolic algebra libraries into deal.II.
Dr. Bruno Turcksin is a computational scientist at Oak Ridge National Laboratory. He previously held postdoctoral research positions at Oak Ridge National Laboratory and Texas A&M University. He earned his Ph.D. in nuclear engineering from Texas A&M University (2012). His research focuses on performance portability and additive manufacturing. He is a key developer of the Kokkos library, which enables algorithms to be mapped to diverse target architectures, applying architecture-specific optimizations for optimal performance. His work also encompasses additive manufacturing and data assimilation, where he collaborates with a multidisciplinary team to advance the fabrication of complex metal parts. Learn more about Dr. Turcksin.
Dr. David Wells is a research scientist in the mathematics department of the University of North Carolina, Chapel Hill (UNC). He received his Ph.D. in applied mathematics from Virginia Tech (2015), while studying under Traian Iliescu. Before coming to UNC, he was an RTG Postdoctoral Fellow at Rensselaer Polytechnic Institute, where he worked with Fengyan Li and Jeff Banks. Dr. Wells’ primary research goal is to develop tools in applied mathematics and computational science to solve real-world problems and give practitioners actionable advice. Publishing high-quality open-source software is the primary means by which his research is made publicly accessible. As such, he is a principal developer of both deal.II and IBAMR, a fluid-structure interaction library. At UNC, Dr. Wells supports the technical infrastructure of the Cardiovascular Modeling and Simulation Laboratory led by Dr. Boyce Griffith. This research, conducted in collaboration with the McAllister Heart Institute and UNC’s hospital system, led to the development of the world’s first fluid-structure interaction model of the human heart, incorporating anatomically and physiologically detailed representations of all four cardiac valves.
The authors collaborated on their answers to our questions.
Q: Why are you all excited to receive the award?
A: Much of industrial and applied mathematics involves computing – computing solutions of linear systems, of differential or partial differential equations, optimization, etc. All of this requires software, and we all rely on that software every day. Yet, historically, writing this software was treated as something one also does, in addition to developing the underlying methods and writing the papers that document the results. Rarely was the act of writing the software considered equal to developing the mathematics, even if the algorithms used were absolutely non-trivial or if the resulting software package was professionally developed and widely used.
As a consequence, it is exciting for us to be recognized for the effort we have been putting into deal.II over the many years we have been working on this project. We are also honored to be in excellent company, seeing that the authors of PETSc and SUNDIALS – both also widely used packages that we hold in high regard – have received the SIAM/ACM Prize in Computational Science and Engineering before.
Q: Could you tell us about the research that won your team the award?
A: Writing widely usable software such as deal.II has both a technical and a research component. The technical side involves extensive test suites, thousands of pages of documentation, deep thought, and much empirical observation on how one can teach a software package that by now has nearly a million lines of code and thousands of classes and functions available to users. But there is also a research component. Many of the data structures and algorithms used in deal.II – entirely hidden from view of a user – are quite complex. They are what sets deal.II apart from your typical graduate student software that does what it’s supposed to do, but is not efficient and probably does not scale to tens of thousands of MPI processes. Collectively, the dozens of publications that describe the algorithmic underpinning of deal.II run into many hundreds of pages. They describe how abstract mathematical ideas are translated into concrete algorithms that can be efficiently implemented.
Q: What does your team's work mean to the public?
A: With good reason, mathematicians think of and pride themselves as providing a language that transcends disciplines. At the same time, a perhaps underappreciated truth is that the methods we come up with are often so complex and sophisticated that practitioners in other fields cannot reasonably implement them. To name just a few examples, many commercial codes across the disciplines still use direct solvers instead of Krylov-space methods for linear systems; uniform mesh and low-order instead of adaptive mesh variable-order discretizations; or, fixed point instead of properly globalized Newton solvers. We know that these codes are not as efficient as they could be, but the more efficient algorithms developed over the past few decades are objectively not easy to implement.
Software packages like deal.II make the mathematical state of the art accessible to broad range scientific and engineering disciplines, and indeed, one can find papers from acoustics to zoology that use deal.II; the library is also used in a wide range of commercial projects. We think of deal.II as a technology transfer tool that brings mathematics to other areas, and in return, brings problems that we cannot currently solve efficiently from the applied sciences to the attention of mathematicians with the experience to analyze them.
Packages such as deal.II also democratize the sciences. It no longer takes a large research group to implement advanced solvers for complex problems. Indeed, deal.II is used around the world, with many users also in countries that have not traditionally had the resources to contribute significantly to the worldwide research enterprise.
Interested in submitting a nomination for the SIAM/ACM Prize in Computational Science and Engineering? The prize next opens for nominations on March 1, 2026.
Haim Avron, Lior Horesh, Misha E. Kilmer, and Elizabeth Newman
The team of Haim Avron, Lior Horesh, Misha E. Kilmer, and Elizabeth Newman are the recipients of the 2025 SIAM Activity Group on Computational Science and Engineering Best Paper Prize. The team received the award for their paper, “Tensor-tensor algebra for optimal representation and compression of multiway data." Proceedings of the National Academy of Sciences, 118 (28) pp. e2015851118 (2021). The paper was selected for “groundbreaking work in tensor-tensor algebra, advancing data compression, theory, and applications, enabling superior efficiency and broad impact across disciplines, including simulations and data science.”
Dr. Elizabeth Newman will present the paper at the 2025 SIAM Conference on Computational Science and Engineering (CSE25) on Monday, March 3 at 1:10 p.m. CST.
The SIAM Activity Group on Computational Science and Engineering awards the prize every two years to the author(s) of the best paper, as determined by the prize committee, on the development and use of mathematical and computational tools and methods for solving problems that may arise in broad areas of science, engineering, technology, and society. The prize recognizes a paper that makes an outstanding and potentially long-lasting contribution to the field. The selection criteria emphasize multidisciplinary work opening up new areas of research, and potential broad impact, in addition to novelty, creativity, and overall scientific advancement and quality.
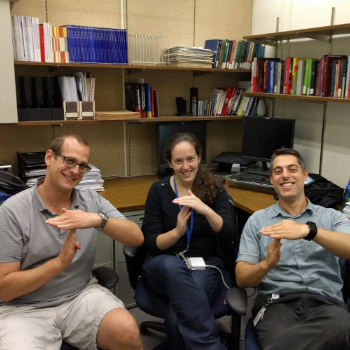
Dr. Haim Avron is a professor in the applied mathematics department at Tel Aviv University. His research focuses on numerical computing and high-performance computing, and their applications in scientific computing and machine learning. His interests and work range from mathematical and computational foundations to end-to-end implementation aspects. Dr. Avron completed his Ph.D. at the School of Computer Science at Tel Aviv University under the supervision of Professor Sivan Toledo. Afterward, he spent two years as a postdoctoral researcher in the Business Analytics and Mathematical Sciences department at the IBM T.J. Watson Research Center before becoming a research staff member in the Mathematical Sciences & Analytics department (2012-15). He then joined Tel Aviv University in 2015. Learn more about Dr. Avron.
Dr. Lior Horesh is a Principal Research Scientist, master inventor, and a Senior Manager of the Mathematics and Theoretical Computer Science department at IBM Research. His group’s mission is to approach some of the big challenges in science and AI, from a principled mathematical angle. This involves conceiving novel mathematical theories, efficient algorithms and insightful analysis tools. Additionally, Dr. Horesh holds an adjunct associate professor position at the computer science department of Columbia University where he teaches graduate level advanced machine Learning and quantum computing courses. Dr. Horesh received his Ph.D. from the University College London (2006) and then joined IBM Research (2009). Dr. Horesh's own research focuses on algorithmic and theoretical aspects of tensor algebra, numerical analysis, simulation of complex systems, inverse problems, non-linear optimization, experimental design, machine learning, quantum computing and the interplay between deductive logic derivation (first-principles modelling), and inductive statistical AI in the context of symbolic scientific discovery. Learn more about Dr. Horesh.
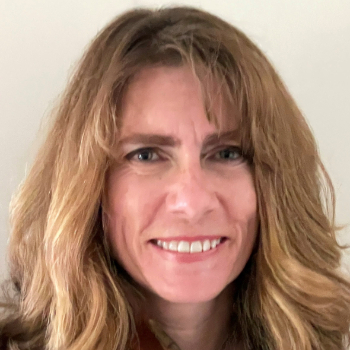
Dr. Misha E. Kilmer is the William Walker Professor of Mathematics at Tufts University and is concurrently serving as Deputy Director of the Institute for Computational and Experimental Research in Mathematics at Brown University. In 2019, Dr. Kilmer was named a SIAM Fellow for her fundamental contributions to numerical linear algebra and scientific computing, including ill-posed problems, tensor decompositions, and iterative methods. She served as a section editor for SIAM Review, SIAM’s flagship journal, for five years. Dr. Kilmer had the honor of being a Kirk Distinguished Visiting Fellow at the Isaac Newton Institute for Mathematical Sciences at Cambridge University in Spring 2023. She is an inaugural member of the Tufts Chapter of the National Academy of Inventors, the 2023 recipient of the Tufts Faculty Distinguished Scholar Award, and earned the unique distinction of being promoted directly from assistant to full professor at Tufts in 2005. Learn more about Dr. Kilmer.
Dr. Elizabeth Newman is an assistant professor in the department of mathematics at Emory University. Her research falls broadly into two categories of computational mathematics: extending numerical linear algebraic tools to multilinear algebra and developing efficient optimization methods for machine learning. Previously, she was a postdoctoral fellow at Emory University working with Dr. Lars Ruthotto (2019-22) and received her Ph.D. in mathematics from Tufts University (2019), advised by Dr. Misha Kilmer. She graduated with a Bachelor of Science in mathematics and statistics from Haverford College (2014). Learn more about Dr. Newman.
The authors collaborated on their answers to our questions.
Q: Why are you all excited to receive the award?
A: Our work began with a decades-long open question: can tensor-based approaches mirror their matrix-based counterparts. This motivated our development of a "matrix-mimetic" tensor algebraic framework that could be understood and utilized akin to matrix algebra. We are excited that the end result of this long-term academic and industry collaboration is the solution to this open problem. This award recognizes not only our past efforts but also energizes us to continue pushing the boundaries of what is possible in tensor processing.
Q: Could you tell us about the research that won your team the award?
A: Matrix algebra possesses many desirable properties, with the Eckart-Young theorem being particularly noteworthy, as it states that truncated Singular Value Decomposition (SVD) offers an optimal low-rank approximation to the original matrix. Our research makes a fundamental contribution to tensor computation by extending the Eckart-Young theorem to tensors.
Developing and working in a new matrix-mimetic tensor algebra enabled us to prove:
- Optimality: Truncated decompositions are provably optimal.
- Superiority: Unless the underlying tensor has no high-order correlations, the tensor decomposition offers better compression than the matrix one.
- Dual Role: Just like matrix algebra, where a matrix can be treated as either a data object or as an operator, tensors now also have this dual role property.
- Tensor Context: Our tensor-tensor decompositions have connections to other tensor decomposition methods.
By showing that truncated SVDs in tensor format can achieve superior compression compared to traditional matrix-based methods, the study has opened new avenues for data analysis and beyond. This work bridges theoretical advancements with practical algorithmic implementations, which hopefully will make a significant impact across various disciplines.
Q: What does your team's work mean to the public?
A: The world is intrinsically multidimensional. Our work provides accessible, computationally practical, and theoretically-grounded machinery to enable tensor-based approaches to be adopted across mathematical, computational, and scientific fields. The matrix-mimeticity of our framework enables seamless integration of tensor operators in classical numerical algorithms and offers rigorous theoretical guarantees. It is our hope that the work in our paper will find utility in new applications and disciplines, provide a basis for future theoretical discoveries, and inspire new, interdisciplinary collaborations.
Q: What does being a member of SIAM mean to your team?
A: We all have benefited from being part of the welcoming, supportive, and vibrant SIAM community. In fact, SIAM’s mission to bridge like-minded researchers from academia and industry reflects our cross-institutional collaboration on this work. Being a part of SIAM has fueled our passion for continual learning and innovation, facilitated lasting scientific collaborations, and provided stimulating opportunities to share our latest research; in short, SIAM is our intellectual home.
Interested in submitting a nomination for the SIAM Activity Group on Computational Science and Engineering Best Paper Prize? The prize next opens for nominations on March 1, 2026.
Omar Ghattas
Dr. Omar Ghattas, The University of Texas at Austin, is the recipient of the 2025 Ivo & Renata Babuška Prize. He received the award “for groundbreaking interdisciplinary contributions to the theory and algorithms of Bayesian inverse problems, and their high-impact application across the geosciences.” The Babuška Prize lecture will be given at the 2025 SIAM Conference on Computational Science and Engineering (CSE25) on Monday, March 3 at 4:35 p.m. CST.
Established in 2022 through a generous gift from Ivo and Renata Babuška, SIAM awards this prize every two years in recognition of high-quality interdisciplinary work that targets any aspect of modeling and numerical solution of a specific engineering or scientific application, including mathematical modeling, numerical analysis, algorithms, and validation. This will be the first time that the prize will be awarded.
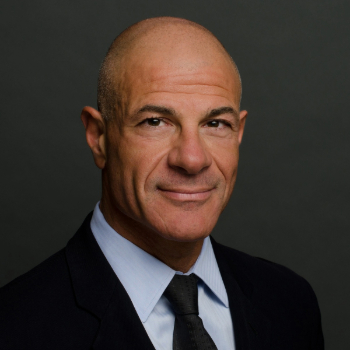
Dr. Ghattas is a professor of mechanical engineering at The University of Texas at Austin, where he holds the Fletcher Stuckey Pratt Chair in Engineering. He is also the Director of the OPTIMUS (Optimization, Inverse Problems, Machine Learning, and Uncertainty for Complex Systems) Center in the Oden Institute for Computational Engineering and Sciences. Additionally, he is a faculty member in the Computational Science, Engineering, and Mathematics interdisciplinary Ph.D. program within the Oden Institute and holds courtesy appointments in geological sciences, computer science, and biomedical engineering.
Dr. Ghattas earned his Bachelor of Science in Engineering in civil and environmental engineering, as well as his Master of Science and Ph.D. in computational mechanics, from Duke University. He spent 16 years as a faculty member at Carnegie Mellon University before joining the University of Texas at Austin in 2005.
His research contributions have been recognized with numerous accolades. With collaborators, he received the ACM Gordon Bell Prize for Special Achievement (2003) and again for scalability (2015), and was a finalist for the award in 2008, 2010, and 2012. He received the 2019 SIAM Activity Group on Computational Science and Engineering Best Paper Prize and the 2019 SIAM Activity Group on Geosciences Career Prize. He was named a 2014 SIAM Fellow and serves on the National Academies Committee on Applied and Theoretical Statistics.
Dr. Ghattas is also the director of the Mathematics of Machine Learning, Modeling, and Data for Digital Twins Center, a multi-institutional collaboration funded by the Department of Energy’s Advanced Scientific Computing Research program, which focuses on developing the mathematical foundations for digital twins. Additionally, he serves as Co-Principal Investigator and Chief Scientist for the Texas Advanced Computing Center’s Frontera high-performance computing system.
Dr. Ghattas’s research focuses on advancing mathematical, computational, and statistical theory and algorithms for large-scale inverse and optimization problems governed by models of complex engineered and natural systems. His group develops algorithms to address challenges in Bayesian inverse problems, Bayesian optimal experimental design, and stochastic optimal control and design for large-scale systems. Their work involves structure-exploiting methods for dimension reduction, surrogate modeling, and neural network approximation, integrated with high-performance computing techniques. These components are coupled to form frameworks for digital twins, with applications spanning geophysics and climate science—including ice sheet dynamics, ice-ocean interaction, seismology, subsurface flows, poroelasticity, and tsunamis—as well as advanced materials and manufacturing processes such as metamaterials, nanomaterials, and additive manufacturing. His research also extends to gravitational wave inference. Learn more about Dr. Ghattas.
Q: Why are you excited to receive the award?
A: It is a tremendous honor and thrill to have been selected for this inaugural SIAM prize recognizing interdisciplinary work in computational science and engineering. I give all the credit to the outstanding students, postdocs, and colleagues I have been fortunate enough to work with over the years. What makes this prize particularly special is that it bears the name of my cherished long-time colleague in the Oden Institute at UT Austin. Ivo Babuška was an intellectual giant in the field of computational science and engineering, continuing to publish papers into his mid 90's. To learn about his career, I recommend Doug Arnold's excellent remembrance of Ivo in SIAM News. Briefly, Ivo was one of the founders of the mathematical theory of finite element methods and made numerous fundamental contributions across a spectrum of topics involving error analysis, reliability, and robustness of FEMs. In his later years he championed the need for uncertainty quantification for complex models, and pioneered methods for random and stochastic PDEs as well as verification and validation of computer models.
I had the privilege of being Ivo's colleague for 16 years at the Oden Institute. He was a larger-than-life presence at the institute, where he spent the last 25 years of his career. He regularly held court in his office to a steady stream of students, postdocs, colleagues, and visitors, always generous with his time and eager to debate the central question that motivated his work: How can we quantify and increase our confidence in the predictions of complex numerical models? His training as both a civil engineer and a mathematician gave him a special appreciation for the field of computational science & engineering. In one of his final acts of generosity, he established the Ivo & Renata Babuška Prize to recognize interdisciplinary work on engineering or scientific problems that integrates perspectives from modeling, numerical analysis, algorithms, and validation. I can't think of a more meaningful recognition than to be the inaugural recipient of this prize.
Q: Could you tell us about the research that won you the award?
A: This prize recognizes collaborative research with several dozen of my current and former students, postdocs, and colleagues on Bayesian inverse problems (BIPs) and their application to geophysical systems. Mathematical models of physical systems are often characterized by uncertain parameters. In a BIP, we seek to update our knowledge of these parameters via a so-called posterior probability distribution that merges prior knowledge with observational data and the mathematical model mapping parameters to observables. Often these parameters represent (discretizations of) infinite-dimensional fields, such as initial conditions, boundary conditions, source terms, or heterogeneous coefficients of a PDE system. Solution of the BIP requires statistical characterization of the posterior; this is extremely challenging in high dimensions and for complex forward models, since each evaluation of the posterior entails solving the forward model, and millions of such evaluations may be required using black-box statistical methods. By building on recent advances in the theory of infinite dimensional Bayesian inverse problems, developing Markov chain Monte Carlo sampling algorithms that are tailored to the geometry of the posterior and scale to high dimensions, and exploiting new supercomputer architectures, we have been able to address a number of large-scale geoscience inverse problems over the past decade. These include Antarctic ice sheet flow, coupled subsurface flow and geomechanics, seismic wave propagation, earthquake source inversion, mantle convection, and tsunami early warning.
Q: What does your work mean to the public?
A: Inverse problems abound in all areas of science, engineering, medicine, and technology. Geophysical problems are among the richest sources of challenging inverse problems. For example, when you check the weather forecast, you've benefited from inverse problems that are solved with continuous streams of observations. Or, when energy and mineral companies search for or manage resources, they employ inverse methods. Earthquake sources are inferred from seismic and geodetic data, as is the structure of the Earth. Uncertain states and parameters of atmospheric, ocean, ice, and land components of climate models are inferred from historical and present-day climate observations and proxy data. And subsurface contaminants are inferred by solving inverse problems. Beyond the geosciences, inverse problems directly impact the public in numerous areas, from medical imaging to calibration of models of engineered systems and processes such as aerospace and automotive structures, chemical plants, and electronics for design and control purposes. Even the field of machine learning relies fundamentally on the solution of inverse problems, when machine learning models are trained on data.
Q: What does being a member of SIAM mean to you?
A: Everything! First class journals, conferences, books, and most importantly, community.
Interested in submitting a nomination for the Ivo & Renata Babuška Prize? The prize next opens for nominations on March 1, 2026.
Jiequn Han
Dr. Jiequn Han, Flatiron Institute, is the recipient of the 2025 SIAM Activity Group on Computational Science and Engineering Early Career Prize. He received the award for his “outstanding theoretical, algorithmic and computational software contributions to the fields of deep learning, stochastic control, stochastic differential equations, and molecular dynamics.” His lecture, titled "Solving High-Dimensional Partial Differential Equations using Deep Learning: Original Insights and Recent Progress," will be given at the 2025 SIAM Conference on Computational Science and Engineering (CSE25) on Monday, March 3 at 1:40 p.m. CST.
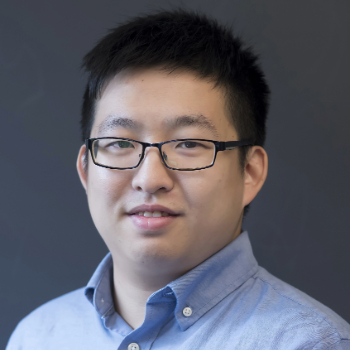
The SIAM Activity Group on Computational Science and Engineering awards the prize every two years to one post-Ph.D. early career researcher in the field of computational science and engineering for outstanding, influential, and potentially long-lasting contributions to the field within seven years of receiving the Ph.D. or equivalent degree as of January 1 of the award year. The contributions must be contained in a paper or papers published in English in peer-reviewed journals.
Dr. Han is a research scientist in the Center for Computational Mathematics, Flatiron Institute, Simons Foundation. He holds a Ph.D. in applied mathematics from Princeton University (2018), as well as a B.S. in computational mathematics and a B.A. in economics from Peking University (2013). He conducts research on machine learning for science, drawing inspiration from various scientific disciplines and focusing on solving high-dimensional problems in scientific computing, primarily those related to PDEs. Learn more about Dr. Han.
Q: Why are you excited to receive the award?
A: I am deeply honored to receive the SIAM Activity Group on Computational Science and Engineering Early Career Prize. The previous recipients have made outstanding contributions to computational science and engineering, making this recognition especially meaningful to me. This award acknowledges my contributions to scientific computing through deep learning techniques, a journey I have pursued since the earliest stages of the field. I have been fortunate to study and work at outstanding universities and research institutions, and I am immensely grateful for the support of my mentors, collaborators, and colleagues. Inspired by this recognition, I look forward to continuing interdisciplinary research in scientific machine learning.
Q: Could you tell us about the research that won you the award?
A: My research focuses on solving challenging high-dimensional computational problems by integrating deep learning with scientific computing. One of my earliest contributions, “Solving high-dimensional partial differential equations using deep learning,” demonstrated how deep learning could overcome the curse of dimensionality in solving partial differential equations (PDEs), a long-standing challenge in the field. This work, which predates well-known developments like physics-informed neural networks, has been widely adopted and has inspired numerous follow-up studies.
Beyond PDEs, I have worked on several interdisciplinary projects, including machine learning-based molecular dynamics simulations, multiscale modeling, stochastic control, game theory, and variational Monte Carlo methods. These contributions have advanced computational techniques across physics, engineering, and social science. A common theme across these works is the development of scientifically rigorous and computationally efficient methods that combine the strengths of machine learning with classical scientific computing.
Q: What does your work mean to the public?
A: Scientific computing plays a crucial role in solving real-world problems through simulation, often involving complex high-dimensional systems. However, solving these systems is often challenging for traditional numerical methods. My work integrates machine learning with established numerical techniques in a principled way to overcome these limitations, making complex simulations significantly faster, more efficient, and more accessible — sometimes by orders of magnitude. These advancements have broad applications in simulation, predictive modeling, and optimal control for physics, engineering, and social science. Examples include improving fluid dynamics models, enhancing molecular simulations for materials design, aiding medical imaging, and optimizing decision-making in economics. Ultimately, my goal is to harness machine learning to develop more accurate, reliable, and scalable scientific computing methods to address pressing challenges in science and technology.
Q: What does being a member of SIAM mean to you?
A: I appreciate SIAM for fostering a community of pure, applied, and industrial mathematicians, united by its mission to connect mathematics with science and technology. Being a SIAM member means engaging with a diverse community that shares a common mathematical language while exploring a broad range of real-world challenges that drive mathematical innovation. SIAM has been instrumental in shaping my research, inspiring me through its journals and conferences while also providing key platforms to share my own contributions. Beyond this, I am honored to serve as an associate editor for the new scientific machine learning section of SIAM Journal on Scientific Computing, where we aim to highlight outstanding contributions in this rapidly evolving field.
Interested in submitting a nomination for the SIAM Activity Group on Computational Science and Engineering Early Career Prize? The prize next opens for nominations on March 1, 2026.
Erin Carson
Dr. Erin Carson, Charles University, is the recipient of the 2025 James H. Wilkinson Prize in Numerical Analysis and Scientific Computing. She received the award “for her impactful contributions to the performance and stability of mixed-precision numerical linear algebra.” Her lecture, titled "Mixed Precision Numerical Linear Algebra." will be given at the 2025 SIAM Conference on Computational Science and Engineering (CSE25) on Monday, March 3 at 5:05 p.m. CST.
SIAM awards the prize every four years to one individual for research in, or other contributions to, numerical analysis and scientific computing during the six years preceding the award year. The purpose of the prize is to stimulate early career contributors and to help them in their careers.
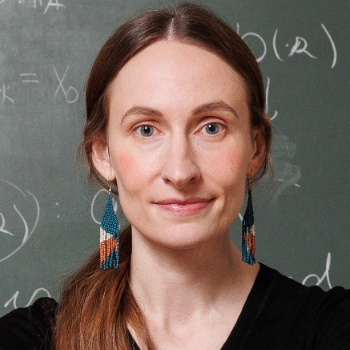
Dr. Carson is an assistant professor in the Faculty of Mathematics and Physics at Charles University. Her research involves the analysis of matrix computations and the development of parallel algorithms for large-scale problems, with a particular focus on their finite precision behavior on modern heterogeneous hardware. She obtained her Ph.D. at the University of California, Berkeley, and was formerly a postdoctoral researcher at the Courant Institute of Mathematical Sciences, New York University. She currently serves as secretary of the SIAM Activity Group on Supercomputing, the co-chair of the GAMM Activity Group on Applied and Numerical Linear Algebra, an associate editor of ACM Transactions on Parallel Computing, and a member of the EuroHPC Joint Undertaking Access Resource Committee. She is the principal investigator of the 2022 ERC Starting Grant inEXASCALE, and has previously been involved in the U.S. Exascale Computing Project. Learn more about Professor Carson.
Q: Why are you excited to receive the award?
A: It is a great pleasure for me to receive the 2025 James H. Wilkinson Prize in Numerical Analysis and Scientific Computing. So much of my research relies on the foundational work done by James H. Wilkinson in numerical linear algebra, and his contributions have been a great inspiration to me. I am honored that the community has chosen to recognize my work in this area.
Q: Could you tell us about the research that won you the award?
A: My work involves the development of mixed precision algorithms for matrix computations and numerical linear algebra. The main idea is to selectively use low precision in some parts of an algorithm and higher precision in others, such that the performance of an algorithm is improved while maintaining important numerical properties like stability and accuracy. This requires both finite precision analysis and an understanding of the cost of practical computations.
Q: What does your work mean to the public?
A: Matrix computations are at the core of nearly all applications in scientific computing, data science, and machine learning. By taking advantage of modern mixed precision computing hardware, we can potentially significantly improve the performance of matrix computations, and thus improve the performance of many critical applications.
Q: What does being a member of SIAM mean to you?
A: I consider SIAM to be my professional home. I am grateful for the community and connections it has provided me, and it has undoubtedly contributed to my professional growth.
Interested in submitting a nomination for the James H. Wilkinson Prize in Numerical Analysis and Scientific Computing? The prize next opens for nominations on March 1, 2028.
Stay Up-to-Date with Email Alerts
Sign up for our monthly newsletter and emails about other topics of your choosing.