Accurate and Reliable Measurement of Thoracic Aortic Aneurysm Growth
A thoracic aortic aneurysm (TAA) is a common ailment that is characterized by dilation of the thoracic aorta, which is the largest artery in the body. TAA is increasing in prevalence worldwide; in fact, approximately three percent of patients older than 50 years have a dilated thoracic aorta. Current methods to determine risk of complication, track disease progression (i.e., dilation), and ascertain the need for surgical repair are based on measurements of the maximal aortic diameter. Medical imaging techniques—typically computed tomography angiography (CTA)—can obtain such measurements. However, a major problem with this approach is that aortic growth is slow (often less than one millimeter per year). Additionally, the degree of variability in aortic diameter measurements often prevents confident assessment of disease progression. The multiple sources of measurement variability between CTA studies include differences in location, angulation, and manual caliper placement by physicians or technicians. Furthermore, diameter measurements are inherently one dimensional (1D) and are performed at fixed anatomic locations; they are thus unable to capture the three-dimensional (3D) nature of TAA growth.
Our group developed an image registration-based method called vascular deformation mapping (VDM) to measure the 3D growth of a thoracic aorta [4]. VDM enables comprehensive quantification of aortic growth at all points on the aortic wall, avoiding many of the limitations that are imposed by manual definition of measurement planes. We have tested and validated VDM on both a synthetic and realistic clinical cohort, and it has shown superior measurement accuracy when compared to human raters [1, 3].
The Process of Vascular Deformation Mapping
The core of VDM is image registration, which automates the measurement process and provides a meaningful geometric deformation field that quantifies the voxel-wise displacement between two CT scans. The Jacobian matrix also delivers a powerful description of the deformation field’s local properties, which can facilitate TAA growth analysis [2].
Figure 1 illustrates VDM’s overall pipeline, with registration as its core component. The registration process involves two images: the moving image \(m\) and the fixed image \(f\). Both images are 3D, and each is defined on its own spatial domain: \(\Omega _F \subset \mathbb{R}^3\) and \(\Omega _M \subset \mathbb{R}^3\). The problem of registration then involves finding a transformation function \(\phi\) that spatially aligns \(m(\phi(\textbf{p}))\) with \(f(\textbf{p})\), where \(\textbf{p} \in \Omega_F\). We can identify the transformation \(\phi\) through the optimization problem
\[\phi = \textrm{argmin} \; \mathcal{L} (f, m, \boldsymbol{\phi}).\]
In VDM, the energy function \(\mathcal{L} (f, m, \boldsymbol{\phi})\) contains three terms:
\[\mathcal{L} (f, m, \boldsymbol{\phi}) = \mathcal{L}_{\textrm{sim}} (f,\phi(m)) + \lambda \mathcal{L}_{\textrm{reg}} (\boldsymbol{\phi}) + \gamma \mathcal{L}_{\textrm{seg}} (f,\phi(m)),\]
where \(\mathcal{L}_{\textrm{sim}}\) penalizes differences in appearance, \(\mathcal{L}_{\textrm{reg}}\) imposes regularization on \(\phi\), and \(\mathcal{L}_{\textrm{seg}}\) penalizes the difference between the segmentation of moving and fixed images. \(\lambda\) and \(\gamma\) are hyperparameters that we choose empirically. For \(\mathcal{L}_{\textrm{sim}}\), we use mutual information; for \(\mathcal{L}_{\textrm{reg}}\), we use bending energy [6] and rigidity penalty [7] to regularize the deformation field (i.e., \(\phi\)) as smooth and diffeomorphic. And for \(\mathcal{L}_{\textrm{seg}}\), we choose normalized cross correlation.
![<strong>Figure 1.</strong> Simplified schematic overview of the vascular deformation mapping (VDM) analysis pipeline. Computed tomography (CT) data undergo aortic segmentation (orange box), followed by rigid and deformable registration (blue box). Researchers use the displacement field \(\phi\), which is calculated from the deformable registration, to translate the mesh vertices of the baseline model (blue surface) to the aortic geometry at follow up (red mesh). The ratio of change in the surface area of each mesh element (area ratio) is plotted on the aortic surface via a colorized scale. Figure courtesy of [3].](/media/g3nblqdm/figure1.jpg)
Our registration workflow consists of three main steps: (i) Rigid registration, (ii) aortic centerline alignment, and (iii) deformable registration (see the blue box in Figure 1). We set up different \(\lambda\), \(\gamma\), and image types (mask or CT) for each step according to our purpose. The first step is rigid registration, which only utilizes aorta segmentation to rigidly align the images by minimizing just the \(\mathcal{L}_{\textrm{seg}}\) term. The next step, aortic centerline alignment, is highly regularized by bending energy and aortic rigidity penalties. These penalties implicitly register the aortic centerlines by allowing non-rigid movement of the tissues that are adjacent to the aorta, but only rigid movement of the aorta itself. Most importantly, the third and final step—deformable registration—captures the growth/expansion of the aorta. Our group’s recent publication provides further details about the registration method [1].
In brief, the overall registration pipeline is designed around two principles: (i) A multi-step registration approach that reduces the need for a large capture range, and (ii) quantification of aortic growth via measurement of wall deformation. Our workflow is implemented in an open-source registration toolbox called elastix [5].
Validation of VDM in Synthetic Cases
Given the impossibility of obtaining a ground-truth deformation field directly from images, we evaluated the VDM pipeline via a novel and systematic evaluation process that generates synthetic CTA growth phantoms with variable degrees and locations of aortic wall deformation. To compare the performance of VDM aortic growth measurements with expert analysts, we used these synthetic phantoms and assessed differences in measurement error. We found that VDM measurements of the magnitude and location of maximal diameter change have significantly higher accuracy and lower variability when compared to two expert manual raters (\(p<0.03\) across all comparisons). These results highlight several important advantages over diameter measurements and support validation of the VDM technique for accurate quantification of aortic growth in patients. Figure 2 summarizes our experimental workflow, and Figure 2d depicts the resulting performance comparison between human raters (blue and orange) and VDM (gray).
![<strong>Figure 2.</strong> Validation of three-dimensional vascular deformation mapping (VDM) growth measurements using synthetic phantoms. <strong>2a.</strong> We manually created deformations on three-dimensional meshes using three techniques: radial change, sculpting, and dragging. <strong>2b.</strong> We obtained ground-truth maximal diameter change \(\Delta \textrm{max}D\) and its location on the centerline. <strong>2c.</strong> Two human raters manually measured the maximal diameter change and recorded its location on the centerline. <strong>2d.</strong> We compared VDM-based measurements with manual measurements from the two expert raters. The results indicate that the VDM-based measurements have significantly less variability (i.e., are more precise) than those of the two manual raters. VDM was also considerably more accurate in localizing the area of maximal diameter change. Adapted from [1].](/media/xp0jjckj/figure2_edited.jpg)
Concluding Thoughts
Our experiments have demonstrated that VDM is a reproducible method for comprehensive 3D quantification of longitudinal aortic growth in a realistic cohort of patients with TAA. VDM analysis yielded reliable growth assessments in nearly all surveillance intervals and displayed excellent interrater reproducibility. Through a phantom study, we found that VDM significantly outperformed experienced manual raters in head-to-head measurements of the magnitude and location of aortic growth; such results suggest that this technique could significantly improve the accuracy and reliability of aortic measurements when compared to standard-of-care measurement techniques. Because the accurate assessment of aortic growth is central to the clinical management of patients with TAA, VDM seems likely to improve the care of aneurysm patients. In addition, the 3D nature of VDM may enable a more nuanced assessment of patient risk, disease phenotypes, and growth trajectories than the current 1D diameter metrics — potentially serving to better inform surveillance intervals, assist with treatment decisions, and predict outcomes in patients with TAA.
References
[1] Bian, Z., Zhong, J., Dominic, J., Christensen, G.E., Hatt, C.R., & Burris, N.S. (2022). Validation of a robust method for quantification of three-dimensional growth of the thoracic aorta using deformable image registration. Med. Phys., 49(4), 2514-2530.
[2] Bian, Z., Zhong, J., Hatt, C.R., & Burris, N.S. (2021). A deformable image registration based method to assess directionality of thoracic aortic aneurysm growth. In I. Išgum & B.A. Landman (Eds.), Proceedings of SPIE medical imaging 2021: Image processing (Vol. 11596) (pp. 115962). International Society for Optics and Photonics.
[3] Burris, N.S., Bian, Z., Dominic, J., Zhong, J., Houben, I.B., van Bakel, T.M.J., … Hatt, C.R. (2022). Vascular deformation mapping for CT surveillance of thoracic aortic aneurysm growth. Radiol., 302(1), 218-225.
[4] Burris, N.S., Hoff, B.A., Patel, H.J., Kazerooni, E.A., & Ross, B.D. (2018). Three-dimensional growth analysis of thoracic aortic aneurysm with vascular deformation mapping. Circ. Cardiovasc. Imaging, 11(8), e008045.
[5] Klein, S., Staring, M., Murphy, K., Viergever, M.A., & Pluim, J.P.W. (2009). Elastix: A toolbox for intensity-based medical image registration. IEEE Trans. Med. Imaging, 29(1), 196-205.
[6] Rueckert, D., Sonoda, L.I., Hayes, C., Hill, D.L.G., Leach, M.O., & Hawkes, D.J. (1999). Nonrigid registration using free-form deformations: Application to breast MR images. IEEE Trans. Med. Imaging, 18(8), 712-721.
[7] Staring, M., Klein, S., & Pluim, J.P.W. (2007). A rigidity penalty term for nonrigid registration. Med. Phys., 34(11), 4098-4108.
About the Authors
Zhangxing Bian
Ph.D. Student, Johns Hopkins University
Zhangxing Bian is a Ph.D. student in computer science at Johns Hopkins University. He earned his master’s degree in electrical engineering and computer science from the University of Michigan. His research interests lie in medical image analysis and machine learning.

Charles R. Hatt
Director of Research and Senior Imaging Scientist, Imbio
Charles R. Hatt is the Director of Research and Senior Imaging Scientist at Imbio, Inc., and a research adjunct professor in radiology at the University of Michigan. His primary area of interest is computed tomography imaging biomarker development for lung and heart applications.
Nicholas S. Burris
Assistant Professor, University of Michigan
Nicholas S. Burris, M.D., is an assistant professor of cardiothoracic radiology at the University of Michigan. His clinical and research interests are primarily focused on the use of advanced techniques for medical image acquisition and analysis to better understand diseases of the heart and aorta.
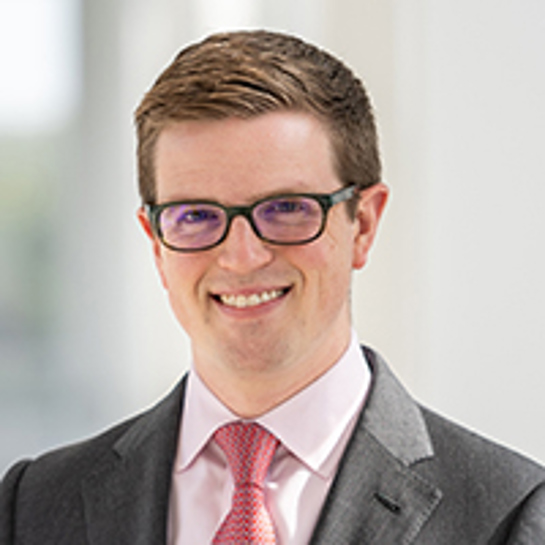
Stay Up-to-Date with Email Alerts
Sign up for our monthly newsletter and emails about other topics of your choosing.