Adaptive Feedback Control Finds Applications in Pest Management
Pest management is crucial for arable farming, but mathematical models of pest species—both flora and fauna—are often highly uncertain. Despite the widespread use of pesticides, significant losses in crop yield occur annually throughout the world. Given these losses, researchers continue to explore various forms of control theory to effectively apply pesticides, implement other forms of biological control, and otherwise manage weeds and animal pests. Though uncommon, the use of adaptive control might prove especially effective in this realm. During a minisymposium presentation at the 2023 SIAM Conference on Control and Its Applications, which took place earlier this week in Philadelphia, Pa., Richard Rebarber of the University of Nebraska utilized an adaptive feedback scheme to generate theoretical methods of pest management. He specifically sought a control method that is both independent of the initial population distribution and robust to model uncertainty, measurement errors, and disturbances.
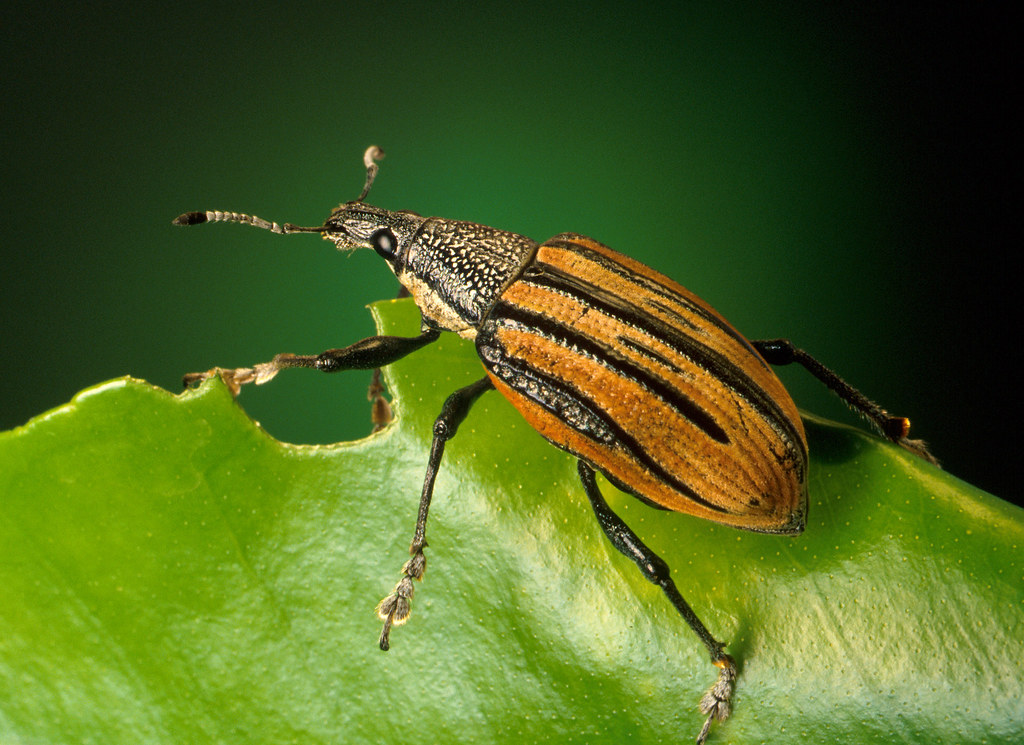
Rebarber began with a brief discussion of open- versus closed-loop control. “In open-loop control, the control is determined at the initial time based on the model,” he said. “In closed-loop control, the control is a feedback of an observation of the system.” Open-loop control is quite effective when the system’s model is very accurate; however, it has no guaranteed robustness and is thus less useful in the presence of model uncertainty or drift. “Most ecological models are uncertain and subject to disturbances and observation error, so open-loop control might be ineffective,” Rebarber said. Certain closed-loop/feedback techniques—including adaptive control—are common in engineering but less so in population management.
Rebarber explained that feedback control is a form of active adaptive management and requires frequent monitoring of at least part of the system. Though it does not necessarily minimize a cost, it is typically robust in the presence of model uncertainties — even without precise knowledge of system parameters. “The more you know about the system, the better performance you can get,” he said.
Rebarber considered stage-structured population projection models for the purpose of this study, which partition individuals into demographically different stage classes (i.e., insect populations are divided into egg, larva, pupa, and adult stages). The stages of many plants, however, are better described as a continuous function (i.e., by stem diameter or another indicator of size).
Rebarber then presented a controlled, observed system in the form of a basic discrete time model. The observation/output for population dynamics may correspond to census or survey data that specifies which stage or stages are monitored; here, a functional selects the stages or weighted sum of stages. Rebarber added a one-dimensional control to the system and noted that in the case of invasive species, the control/input might correspond to the amount of pesticide or biological control that is applied to part of the population.
Next, Rebarber turned to a pillar of robust control theory: adaptive control. “The idea behind simple adaptive control is that the control effort is a dynamic variable that is governed by some prescribed set of equations that are tied to an output—a measured variable—of the to-be-controlled system,” Rebarber said. “The output need not be the whole state; in practice, it may just be a portion of the state.” He explained that the power of simple adaptive controllers comes from three points:
- Ease of computation and implementation
- Ability to achieve dynamic control objectives with a paucity of information, such as only a measured output
- Global robustness properties that ensure the controllers’ theoretical efficacy for all systems from a class that is prescribed by certain structural properties.
![<strong>Figure 2.</strong> Simulations of the adaptive control scheme for 30 projection matrices of the Diaprepes root weevil model. All three controls pull down to 0 at different rates. Figure courtesy of [2].](/media/xsijkjly/pest-management-figure2.jpg)
Rebarber introduced a 2010 stage-structured dispersal model [3] for the spatial spread of the Diaprepes root weevil, a generalist invasive herbivore in citrus-growing regions of the U.S. (see Figure 1). The model has six dimensions that represent the weevil’s six stages: eggs, two larval stages, a pupa stage, and two adult stages. He and his colleagues utilized this model in a 2016 study that published in the SIAM Journal on Applied Mathematics [2]. Their work employed nematodes—small worms that infect and kill their insect larva hosts—to manage pest populations. They assume that hosts are distributed according to a Poisson distribution and that nematode attacks are random and independent; doing so yields the probability that a host is not attacked by nematodes.
Rebarber and his collaborators applied the same principle to the use of insecticides, where the probability term instead measures the probability of a pest surviving a chemical application. The type of control (nematode or insecticide) determines the stages at which one must apply it, since pesticides might act on different stages than biological control measures. Because the control is more impactful in the presence of a higher number of weevils, its effect is proportional to the sum of the state. This suggests that the team should rely on high gain control, which only targets specific life stages of the invasive species. Rebarber assumes that the population will decline with a large enough control (either nematode or insecticide).
To demonstrate the adaptive control scheme’s robustness when modeling uncertainty, Rebarber conducted simulations of the weevil population model for 30 pseudo-randomly generated population projection matrices (see Figure 2). He utilized three distinct design parameters that reveal the speed of adaptation. All three controls pull down to 0 at different rates because they provide varied amounts of control. Nevertheless, the same control strategy achieves stabilization of the state in every case.
Rebarber concluded his talk with some brief comments about extensions of this model, including dead zone feedback and subsequent work on adaptive feedback control in the SIAM Journal on Control and Optimization [1].
References
[1] Edholm, C.J., Guiver, E.C., Rebarber, R., Tenhumberg, B., & Townley, S. (2022). Stabilization by adaptive feedback control for positive difference equations with applications in pest management. SIAM J. Control Optim., 60(4), 2214-2245.
[2] Guiver, C., Edholm, C., Jin, Y., Mueller, M., Powell, J., Rebarber, R., … Townley, S. (2016). Simple adaptive control for positive linear systems with applications to pest management. SIAM J. Appl. Math., 76(1), 238-275.
[3] Miller, T.E.X., & Tenhumberg, B. (2010). Contributions of demography and dispersal parameters to the spatial spread of a stage-structured insect invasion. Ecol. Appl., 20(3), 620-622.
About the Author
Lina Sorg
Managing editor, SIAM News
Lina Sorg is the managing editor of SIAM News.
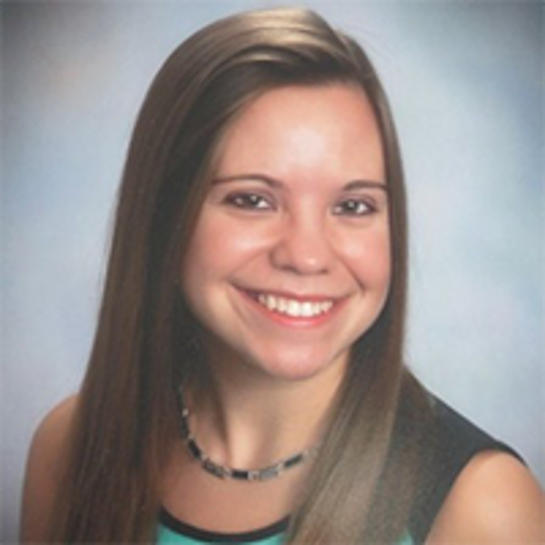
Stay Up-to-Date with Email Alerts
Sign up for our monthly newsletter and emails about other topics of your choosing.