AI in Education: A Progressive, Practical Proposal
Teaching with AI: A Practical Guide to a New Era of Human Learning. By José Antonio Bowen and C. Edward Watson. Johns Hopkins University Press, Baltimore, MD, April 2024. 280 pages, $24.95.
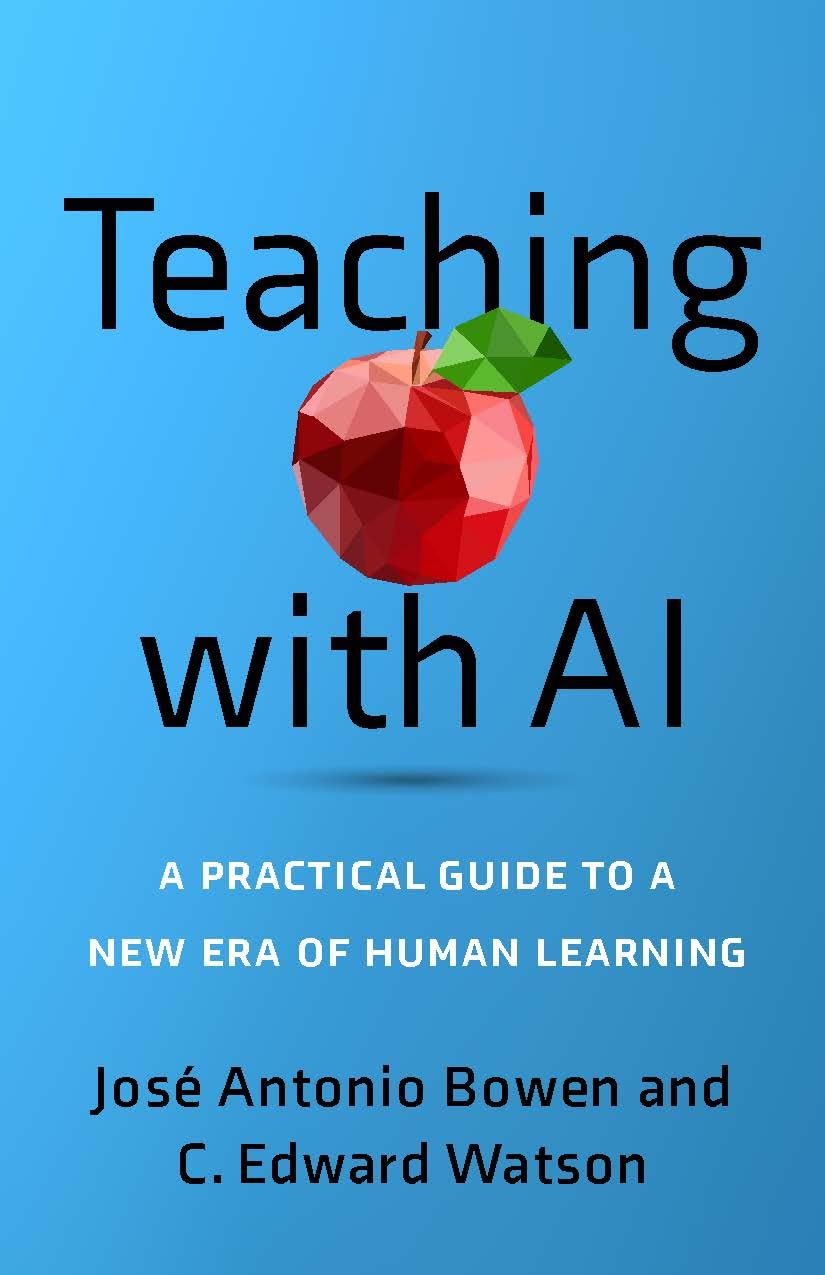
As artificial intelligence (AI) continues to revolutionize mathematics [3], increasingly more students are utilizing the new generation of AI tools for their coursework. In response, educators must adopt, adapt, and implement AI-based mathematical education research that affects their pedagogy. Teaching with AI: A Practical Guide to a New Era of Human Learning by José Antonio Bowen and C. Edward Watson is the best, most balanced, and most insightful guide on this topic that I have found to date. Perhaps I shouldn’t be surprised, as Bowen—a former president of Goucher College—is the author of several popular books on teaching and Watson is Vice President for Digital Innovation at the American Association of Colleges and Universities. The two previously collaborated on a 2017 book titled Teaching Naked Techniques: A Practical Guide to Designing Better Classes [2].
Bowen and Watson assert that educators should not perceive current AI as a threat, but rather welcome AI tools as catalysts for progressive change that enhance learning, develop students’ creative potential, and “transform cheating into innovation.” While the authors do make generic statements like any other pundits—e.g., “Previous AI helped curate your world ... but GPT AI will allow you to create your world”—they explicitly identify the impactful aspects of AI that will inspire users to generate ideas, ask better questions, evaluate assumptions, seek contradictory evidence, investigate additional sources, anticipate problems, clarify concepts, find information, create visualizations, write drafts, offer feedback, analyze data, and so forth.
A number of sources have generated updates of Benjamin Bloom’s classic taxonomy that incorporate AI; for instance, Figure 1 displays an Oregon State University revision of Bloom’s taxonomy that is referenced in the book. One could similarly modify my pyramid of a quantitative reasoning perspective to reflect the significance of AI (see Figure 2). Furthermore, several recent discussions have focused on the movement from Ed 3.0 (one size fits all) to Ed 4.0 (dynamic and responsive) and even Ed 5.0 (adaptive learning) [8]. These levels represent three paradigms: (i) directed education, wherein the student is a recipient; (ii) supported education, wherein the student is a co-learner; and (iii) empowered education, wherein the student is a collaborator [7]. For adult learners, this shift marks a transition from pedagogy to andragogy to heutagogy [1].
While most SIAM News readers can likely skip Bowen and Watson’s introductory chapter about AI and the shift from early rule-based systems to contemporary machine learning and large language models (LLMs), I still found helpful tidbits in their narrative of different AI applications that can survey the literature and possibly verify information. The authors list 12 LLMs (seven of which they describe in terms of ability and limitation) and 20 software intermediaries for specific functions such as writing, research, and coding. This first chapter also contains a glossary of significant terms like LLM, application programming interface, generative AI, and artificial general intelligence.
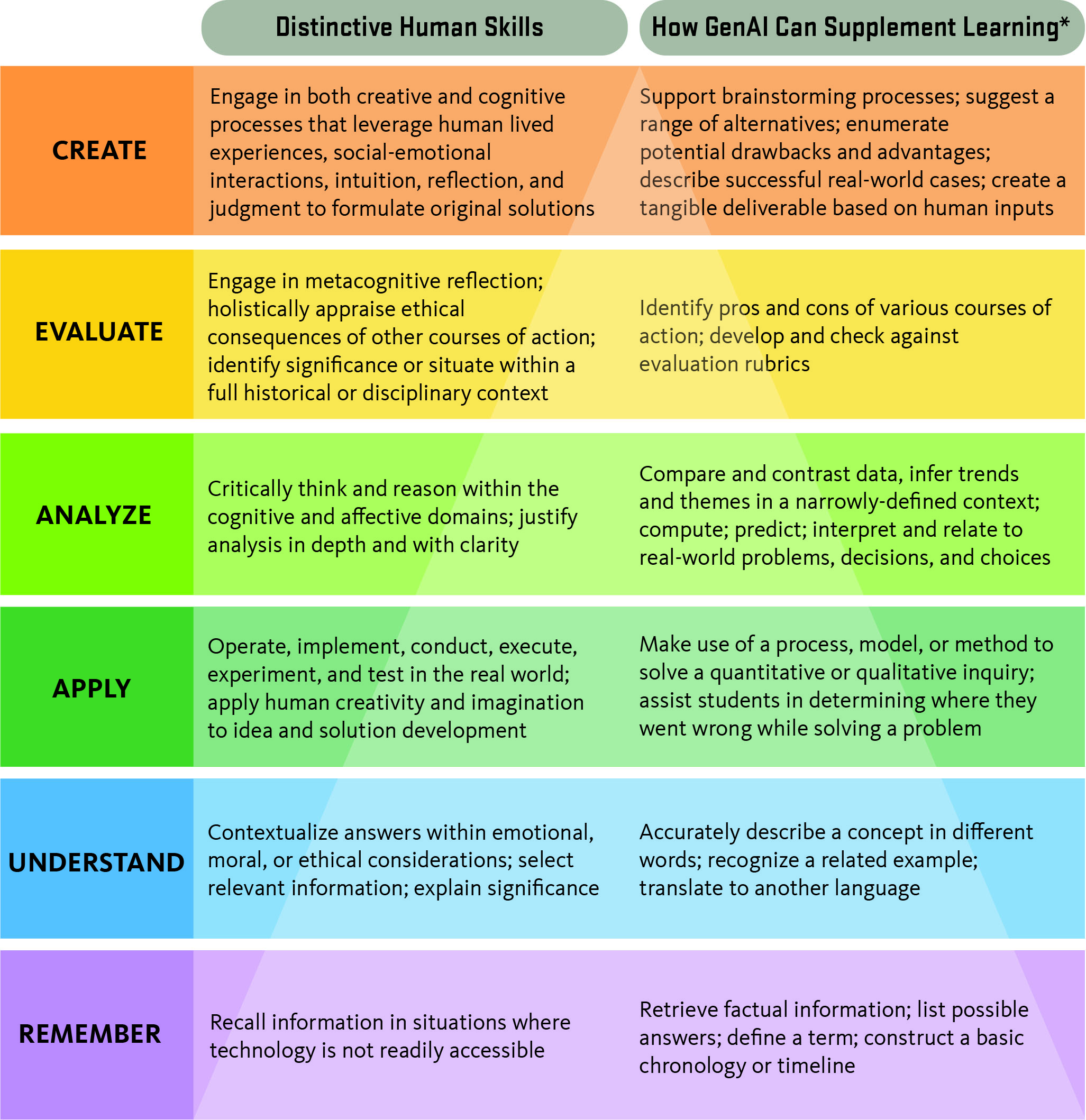
Consistent with existing math education literature on problem posing, Bowen and Watson elaborate on the axiom that “asking the right question requires first questioning the problem.” They illustrate a Double Diamond innovation process that involves both divergent and convergent thinking about discoveries and definitions in problem posing as well as ideas, prototypes, and tests in problem solving. The authors write that “the more we can prepare students to question assumptions, analyze problems more deeply, tolerate the discomfort of ambiguity, find subproblems, and clearly reframe problems…the better prepared they will be not only for the first wave of AI-inspired jobs, but for the subsequent waves that are still unknown.”
While Bowen and Watson explicitly address concerns about cheating and plagiarism, they do so after a chapter titled “Reimagining Creativity” because they believe that educators must first appreciate that AI is iterative. “The goal is not to have AI do the thinking,” they write, “but to have a dialogue that helps you think.” After detailing AlphaFold’s success in the 2020 Critical Assessment of Structure Prediction competition and its subsequent prediction of a three-dimensional structure with more than 200 million proteins, they surmise that AI will significantly impact numerous scientific problems — including star mapping, climate modeling, the new generation of antibodies, and hydrogen fusion research. The authors thus view AI as a partnership, stating that “it is in the iteration, the reflection, the back and forth, and the refined questions that thinking and creativity happen.”
A subsequent chapter about “AI Cheating and Detection” reveals that 22 percent of college students have utilized AI for schoolwork; 49 percent used AI tools on a daily, weekly, or monthly basis in fall 2023; and 89 percent use ChatGPT in some capacity. Given this current state of affairs, the text details a number of AI detectors with varying rates of false positives. Since many of these tools are unreliable (even if they exceed most faculty’s ability to detect AI writing), Bowen and Watson assert that the alternative is better course design. Their proposed strategies include discussing academic integrity, giving “integrity quizzes,” allowing students to withdraw submissions, demonstrating AI detection tools, and normalizing homework assistance. They conclude the chapter by stating that “increasing transparency, relevancy, belonging, and motivation will all reduce cheating, but none will eliminate it.”
This commentary introduces a very interesting rubric with four assessment levels: Absent (0 percent), AI level (50 percent) \(=\) F, Good (80 percent) \(=\) B, and Great (100 percent) \(=\) A. When I tried this rubric on my own students, they only reacted negatively to the criteria for the harsh reality of an “F.” “AI has shrunk the distance between a C grade and an A grade, but it has made articulating the distance between them more important,” the authors write. “Combining high standards with high care, building trust and community, focusing on equity and inclusion, increasing motivations, and creating better, clearer, and more relevant assignments…can both increase learning and reduce cheating.”
![<strong>Figure 2.</strong> Bloom-like taxonomy pyramid of quantitative reasoning. Figure courtesy of [4].](/media/sfkfwp0c/figure2.jpg)
The culminating section of Teaching with AI focuses on “Learning with AI.” Bowen and Watson concentrate on the use of AI to provide instantaneous and individualized feedback; offer responsive tutoring; serve as a debate partner with specificity and contextual knowledge; act as a discussion leader to eliminate certain social pressures; and help students run scenarios, create visualizations, and forecast and test ideas. They note that “if we are to prepare students for a world where collaboration with AI is required rather than prevented, then helping students leverage AI to produce better work should become a signature pedagogy of higher education.”
As a faculty member, I was surprised by the many ways in which AI already influences my professional life. For instance, some journals and grant agencies are deploying AI in a variety contexts that extend beyond checking for plagiarism, quality, and adherence to guidelines even before they share a manuscript or grant application with reviewers. Unfortunately, Teaching with AI was already complete when Lisa Messeri and M.J. Crockett published their “taxonomy of productivity and objectivity” with four aspects of considerable concern about the use of AI for research as an (i) oracle, (ii) surrogate, (iii) quant, and (iv) arbiter [5]. Each of these AI collaborations engenders epistemic, ethical, social, and cultural susceptibilities to illusions of understanding. Messeri and Crockett argue that “the proliferation of AI tools in science risks introducing a phase of scientific enquiry in which we produce more but understand less.”
So what’s missing? Applied mathematics educators may feel that Bowen and Watson are simultaneously too generic and too focused on humanities and social science. With 20 pages of references, readers have plenty of opportunities to peruse further literature. In fact, many SIAM members are already exploring the issues and potentialities of Teaching with AI in their own classrooms. In SIAM News earlier this year, Alvaro Ortiz Lugo summarized a SIAM Education Committee session about AI and education at the 2024 Joint Mathematics Meetings [6]. The eight talks in the session addressed the use of AI in theorem proving; data analysis; modeling; coding and computation; visualization; parameterization; spatial reasoning; audio, video, and text processing; emotion detection and classification; lesson planning; report writing; and more. As AI continues to advance in the coming months and years, increasingly more members of the SIAM community will undoubtedly witness and adapt to its effects in a variety of academic settings.
References
[1] Blaschke, L.M. (2012). Heutagogy and lifelong learning: A review of heutagogical practice and self-determined learning. Int. Rev. Res. Open Distrib. Learn., 13(1), 56-71.
[2] Bowen, J.A., & Watson, C.E. (2017). Teaching naked techniques: A practical guide to designing better classes. San Francisco, CA: Jossey-Bass.
[3] Fink, T. (2024). Why mathematics is set to be revolutionized by AI. Nature, 629, 505.
[4] Jungck, J.R. (2011). Mathematical biology education: Modeling makes meaning. Math. Model. Nat. Phenom., 6(6), 1-21.
[5] Messeri, L., & Crockett, M.J. (2024). Artificial intelligence and illusions of understanding in scientific research. Nature, 627(8002), 49-58.
[6] Ortiz Lugo, A. (2024, May 1). SIAM session at JMM 2024 address artificial intelligence in the education sector. SIAM News, 57(4), 12.
[7] Ouyang, F., & Jiao, P. (2021). Artificial intelligence in education: The three paradigms. Comput. Educ. Artif. Intell., 2, 100020.
[8] Rane, N.L., Choudhary, S.P., & Rane, J. (2023). Education 4.0 and 5.0: Integrating artificial intelligence (AI) for personalized and adaptive learning. J. Artif. Intell. Robot., 1(1), 29-43.
About the Author
John R. Jungck
Professor, University of Delaware
John R. Jungck is a professor in the Department of Mathematical Sciences and Department of Biological Sciences at the University of Delaware. He has been senior editor of the Bulletin of Mathematical Biology’s Education Section since 1997.
Related Reading
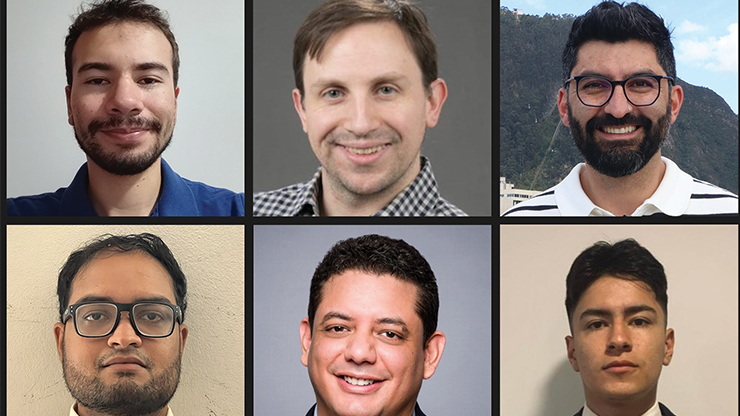
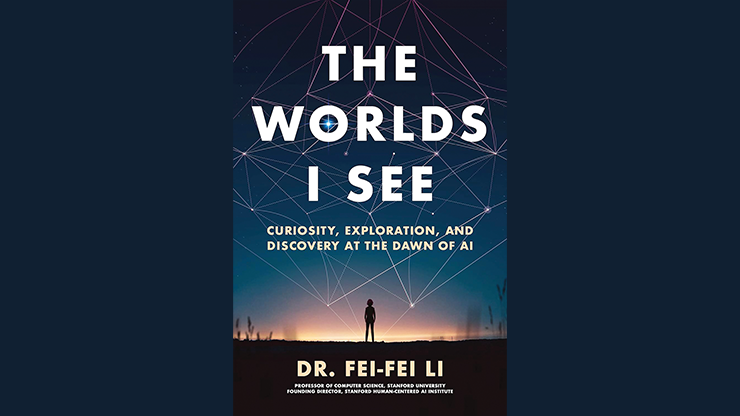
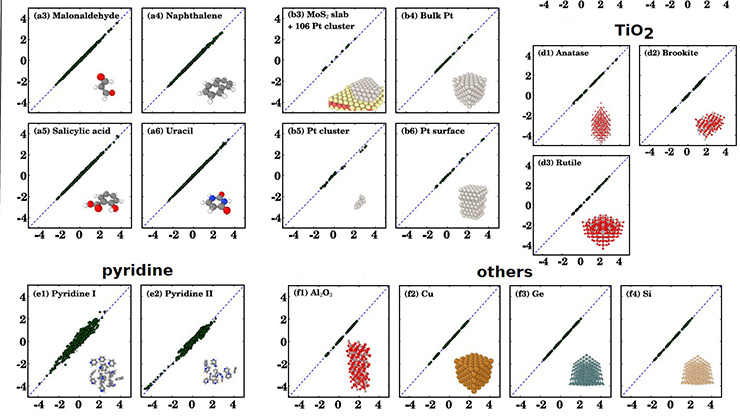
Stay Up-to-Date with Email Alerts
Sign up for our monthly newsletter and emails about other topics of your choosing.