Cataloging Biodiversity with Artificial Intelligence
The International Union for Conservation of Nature (IUCN) has assessed more than 163,000 species to identify those that are threatened with extinction. Of that list, roughly 22,000 species are considered “data deficient” — which means that we simply do not know enough about them to discern whether they are in decline. To complicate matters, the IUCN list does not include every species on Earth because scientists have yet to catalog them all.
Unfortunately, time is of the essence for many organisms. Climate change, pollution, loss of habitat, and other human-imposed pressures have already led to widespread extinctions. Without knowing the number and population stability of certain species, we are missing vital information about the planet’s overall health.
“If you look at biodiversity studies, there is a huge bias in where they are [conducted], who they’re done by, and what species we study,” computer scientist Tanya Berger-Wolf of The Ohio State University said. “This bias translates into a lack of understanding of drivers of biodiversity loss and whether policies work or don’t work. And we’re running out of time.” Given the situation’s severity, Berger-Wolf is collaborating with data scientists, ecologists, and biologists to develop new machine learning (ML) methods to fill these knowledge gaps.
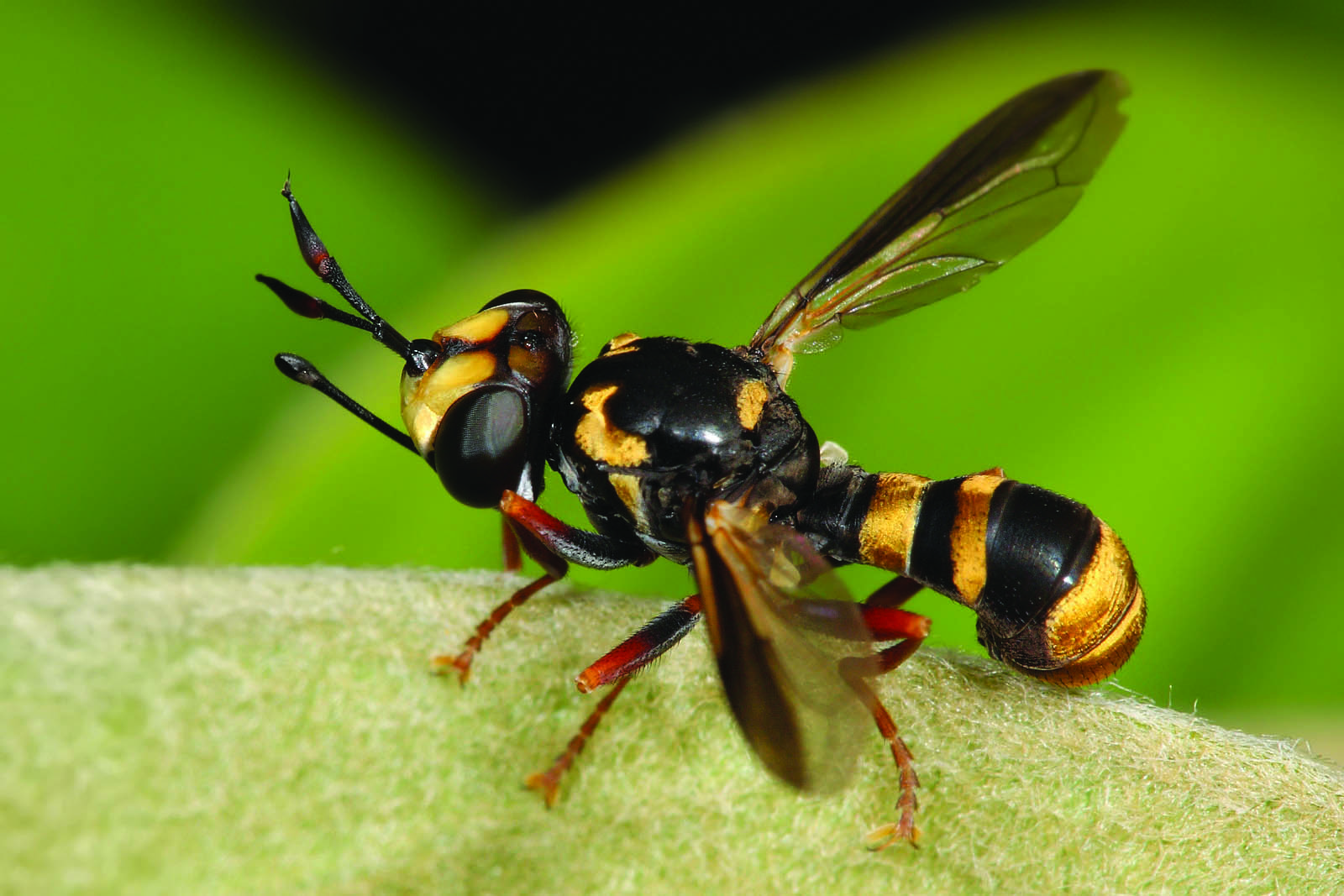
However, the associated computer and data science challenges are immense. The identification of known species from visual or audio data is often difficult even for experts, particularly when species are closely related or bear a strong resemblance due to mimicry or simple coincidence (see Figure 1). Furthermore, many organisms—especially insects and plants—change their appearances significantly throughout their life cycles. “I’m not a field biologist, despite having just returned from the tropical rainforest in Panama,” Berger-Wolf said. “I don’t like bugs and all of that! So what can a computer scientist do with what is clearly an urgent need and a huge challenge in every possible way?”
Computers can only help fill the gaps in biodiversity knowledge if they are well programmed, which in turn requires large quantities of reliable data beyond current data sources. “That means including the millions of images, videos, sounds, and observations on social media and dedicated apps for nature,” Berger-Wolf said. She added that these resources might contain vital information beyond the apps’ intended use; for instance, a photo of a bird may also depict relevant plants in the background. “Data sources have exploded, but we need to develop methods that extract information from high-throughput observations,” Berger-Wolf continued. “That’s a very different approach than what we’ve done before.”
The magnitude of this undertaking is similar to the creation of generative artificial intelligence (AI) models that draw training data from the entire internet (although the ethics of this practice are admittedly questionable) [1]. However, the model output is very different and requires a type of synthetic analysis to recognize organisms across a wide variety of contexts and potentially identify previously undescribed species. This type of work extends far beyond the current capabilities of AI systems.
A Fondness for Beetles
Data in the realm of modern population biology and ecology stems from a wide range of sources: field researchers, remote sensing, robotic drones, trail cameras, microphones, devices that attach directly to animals, and so forth. Regardless of provenance, the most prevalent information comes from photos and (to a lesser degree) videos. Visual data is often provided by non-scientists, as snapping a photo of a bird, bug, or flower does not require special equipment.
Some data sources are meant for sharing and identification. For example, the birdwatching community has hugely benefitted from the internet era; given the wide availability of cameras, apps, and online groups, the hobby is accessible to more people than ever. Other apps and citizen science organizations such as iNaturalist are designed for broader species identification. Yet despite the growing popularity of nature apps, most users live in North America or Western Europe; in contrast, high-profile conservation work often focuses on “charismatic” animal species in more exotic areas — the lions, tigers, and bears of the world. Nothing is intrinsically wrong with these circumstances, but they do yield a glut of data for a relatively small subset of global biodiversity.
“We know a lot about very little,” Berger-Wolf said, noting that scientific descriptions might not yet exist for the majority of species in multiple taxonomic groups. Although scientists estimate that beetles alone comprise roughly a quarter of all animal species, only about 400,000 beetle species have been categorized to date. As biologist J.B.S. Haldane was apparently fond of saying in one form or another, “God has an inordinate fondness for stars and beetles” [2].
Limiting one’s efforts solely to animal species still leaves large blank spaces in the map of scientific knowledge. The catalogs of plants and fungi are even worse and invite additional challenges due to changes over time and the fact that these species spend large portions of their lives hidden from human eyes. For instance, a fungal colony can cover a huge area underground or inside of a tree, but people generally only notice when it sprouts mushrooms. Since many plants and fungi strongly resemble each other, teaching a computer to tell them apart is no small matter.
When Biology Gives You Images, Make Imageomics
Berger-Wolf and her collaborators utilize a framework that was established by the Global Partnership on Artificial Intelligence under the auspices of the Organisation for Economic Co-operation and Development: an international think tank that pursues policies to combat climate change and environmental issues, among other causes. The ethical use of AI is part of their purview, including the application of ML to tackle biodiversity loss.
![<strong>Figure 2.</strong> Machine learning models that classify organisms must be trained on data that properly place them in scientific categories. The TreeOfLife-10M dataset allows users to file organisms in nesting categories from phyla down to species, shown here diagrammatically for arthropods: the group that contains insects, spiders, crabs, and other joint-legged invertebrates. Figure adapted from [3].](/media/chbcl5vi/figure2.jpg)
In a nod to genomics, Berger-Wolf and her colleagues proposed an umbrella category of study called imageomics. Just as geneticists can extract crucial information from an organism’s genome, imageomics integrates all available biologically relevant information from visual data to measure biodiversity; it even identifies new species and determines their relationships to other organisms [4].
To that end, the collaborators constructed a dataset called TreeOfLife-10M that comprises more than 10 million images of over 450,000 species from iNaturalist, the Encyclopedia of Life, and the Bioscan-1M catalog of insect photos in labs. They curated the dataset to amass scientifically reliable images that pre-train their ML system, which they call BioCLIP [3]. As its name implies, this model’s construction is based on the OpenAI image recognition project on Contrastive Language-Image Pre-training (CLIP). Using 10 classification tests on biological images and a subset of images for rare and threatened species, Berger-Wolf’s team compared BioCLIP’s results to those of other image recognition ML models. They found that BioCLIP offered a substantial improvement over general-purpose models, with 16 to 17 percent more correct species identifications.
From Cataloging to Synthesis
ML systems like BioCLIP use training data to output the most probable response based on an input; i.e., better-curated data and better-constructed goals have a higher likelihood of the response matching reality. Identifying known species in images—especially images that were taken precisely for this purpose—is a well-defined ML problem, even if it is not necessarily easy to solve.
Preliminary findings indicate that BioCLIP is able to sort images of organisms according to a broad hierarchy, separating them into scientific categories like phyla and families that document the relationships between species (see Figure 2). Given its narrow purpose, the model is also less energy intensive than large language models, which is another bonus for ecologically minded individuals.
Yet despite its success, BioCLIP is not the end goal for Berger-Wolf and her collaborators. The researchers wish to rethink how ML models work on a fundamental level so they can identify previously undescribed species and fit them within the tangled tree of life — the areas of biology known as taxonomy and cladistics. Doing so raises much broader, less-defined challenges than simple species identification. After all, human biologists are not always consistent in their own taxonomic efforts — particularly when species boundaries are blurry or organisms do not clearly fit into one genus or another.
“We definitely need something like the next generation of AI that can truly do synthesis,” Berger-Wolf said, suggesting that AI techniques will need to combine different sources of information into something new. “This bumps up against the very frontier of computer science and machine learning today.”
Tanya Berger-Wolf gave an invited presentation on this research at the 2024 SIAM International Conference on Data Mining, which took place in Houston, Texas, this past April.
References
[1] Francis, M.R. (2022, November 1). The ethics of artificial intelligence-generated art. SIAM News, 55(9), p. 11.
[2] Gould, S.J. (1993). A special fondness for beetles. Nat. Hist., 102(1), 4.
[3] Stevens, S., Wu, J., Thompson, M.J., Campolongo, E.G., Song, C.H., Carlyn, D.E., … Su, Y. (2024). BioCLIP: A vision foundation model for the tree of life. In Proceedings of the IEEE/CVF conference on computer vision and pattern recognition (pp. 19412-19424). Seattle, WA: Computer Vision Foundation.
[4] Tuia, D., Kellenberger, B., Beery, S., Costelloe, B.R., Zuffi, S., Risse, B., … Berger-Wolf, T. (2022). Perspectives in machine learning for wildlife conservation. Nat. Commun., 13(1), 792.
About the Author
Matthew R. Francis
Science writer
Matthew R. Francis is a physicist, science writer, public speaker, educator, and frequent wearer of jaunty hats. His website is bsky.app/profile/bowlerhatscience.org.
Stay Up-to-Date with Email Alerts
Sign up for our monthly newsletter and emails about other topics of your choosing.