Computational Attorneys: Opportunities and Challenges
The idea of computers that serve as attorneys once seemed like a distant, futuristic concept. However, the advent of large language models means that this seemingly far-off notion might actually be on the horizon. For many years, the legal domain has been an important application field for various data mining and machine learning technologies. While some progress has occurred in the automation of legal compliance management and legal information processing, most advances have focused on specific, well-defined tasks (e.g., the classification or clustering of legal text, legal entity extraction, and legal information retrieval).
We coin the term computational attorney as an intelligent software agent that embodies the future capabilities of these systems and can assist human lawyers with a wide range of complex, high-level tasks, such as drafting legal briefs for prosecution or defense. Here, we reexamine the current research agenda on legal artificial intelligence (AI) by asking a critical question: What does it take to make a computational attorney?
Past and Present
Research on legal AI began in the 1990s when scientists started to utilize data mining and machine learning to address basic legal tasks, like the computer-assisted classification of legal abstracts. Over the years, researchers have harnessed these techniques to automate other minor and repetitive legal projects, including similar case matching, litigation analytics, and information extraction from legal documents [1, 5, 9, 10].
The next big step in the evolution of legal AI was the creation of foundation models for a variety of legal tasks; LEGAL-BERT is one such example [3]. In our opinion, the pinnacle of this line of research is a Large Legal Language Model (L3M) that is pre-trained on an extensive legal corpus. The collection of a massive amount of quality text data in the legal domain, which helps to train and tune such a model, is key to its success.
In addition to handling extremely high labeling costs for demanding legal tasks via zero-shot or in-context learning, L3Ms can also accommodate possible ambiguities and idiosyncrasies to meet the challenges of thoroughness and specialized knowledge in the legal domain. The continued maturation of L3Ms will achieve new heights in legal natural language processing. When an L3M reaches a certain scale, for example, it may start to exhibit “emergent abilities” — one of which is legal reasoning [11]. L3M-based legal prompt engineering has demonstrated impressive performance and potential on legal text entailment and can even answer questions from bar exams [6, 12].
Looking to the Future
While ongoing advancements in L3Ms are encouraging, several challenges currently preclude them from becoming fully functional computational attorneys. We present these challenges as actual measurements for the required performance of a system that uses an L3M. However, it is important to note that future research may identify barriers that today’s transformer-based models cannot overcome.
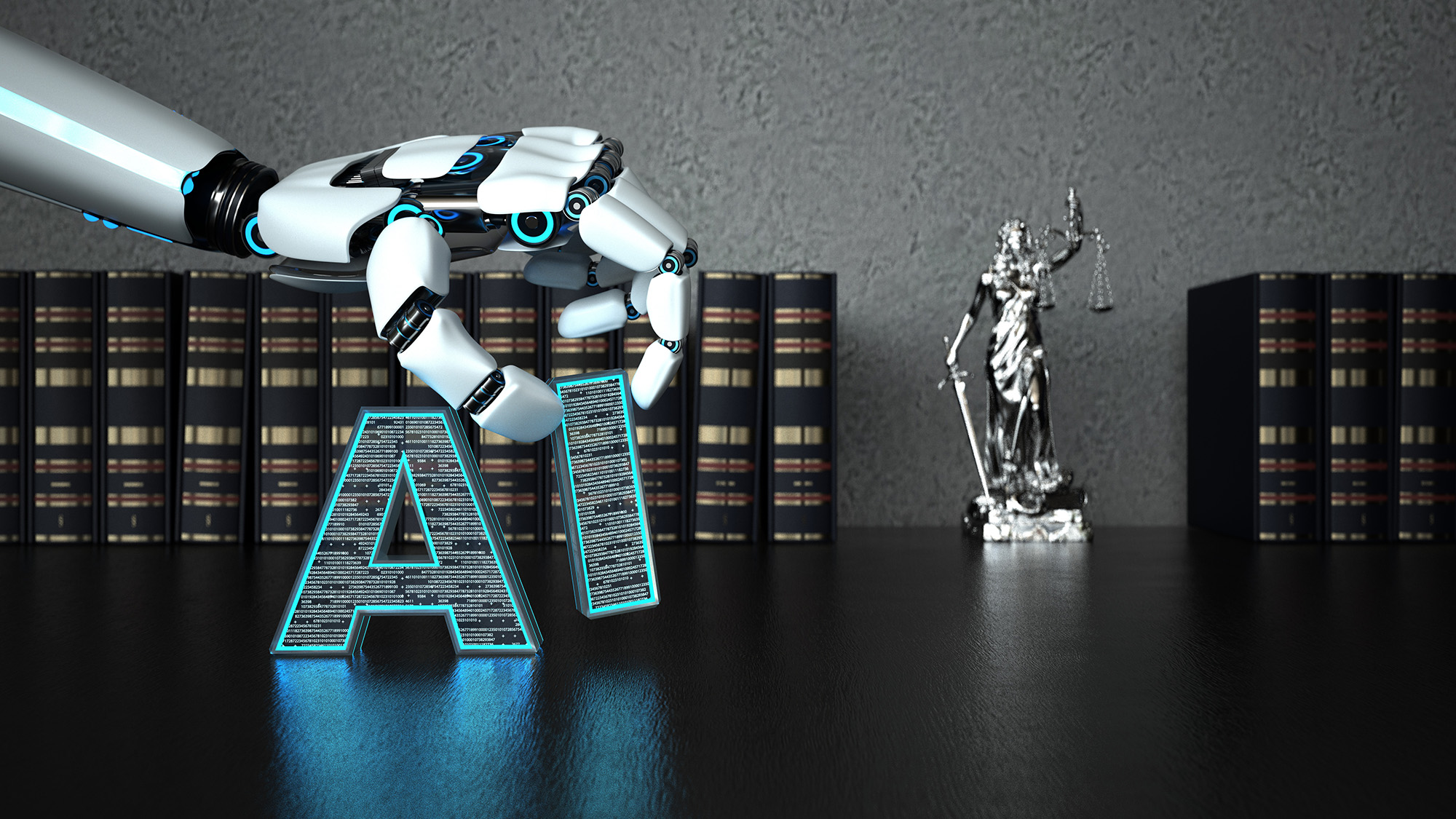
First, these models must be updatable to keep pace with changes in the legal domain. Timely updates with novel information can significantly enhance a model’s ability to deliver reliable solutions. New methods to revise a model in the post-deployment stage are preferable to retraining it from scratch, which is both expensive and time-consuming. Continual learning, machine unlearning, and data stream mining are examples of techniques that can facilitate this process.
Second, these models must be stable. They should be able to reason within the bounds of existing legal systems in the relevant jurisdiction. At the same time, scientists must take appropriate measures to prevent the models from inventing seemingly plausible but nonexistent responses (i.e., hallucinations or confabulations). Retrieval-augmented generation [7]—as well as uncertainty quantification techniques like evidential deep learning—can help ensure stability and avoid confabulations.
Third, these models must be provable. In other words, their legal opinions or judgments need to stem from relevant laws and rules. Provability is a higher requirement than interpretability or explainability, as the models have to justify the correctness and fairness of each step of their reasoning process. Achieving this objective is crucial in the legal context and may involve techniques from abductive reasoning and neuro-symbolic inferencing.
Fourth, these models must be communicable. They should be capable of capturing subtle details and nuances in the instructions from fellow lawyers or legal clients. They should also be teachable, in that they keep learning from human demonstrations and feedback [2, 4]. The creation of an advanced natural language interface is essential for this specific purpose; such an interface must facilitate bidirectional communication, thereby allowing L3Ms to learn from their interactions with humans and integrate new knowledge accordingly.
The development of functional computational attorneys is an exciting prospect that will likely play a central role in the future of the legal industry. It will enhance the efficiency of various legal services (such as conducting research, reviewing documents, preparing for depositions, drafting briefs, and creating contracts) and broaden access to legal assistance by dramatically reducing the associated costs. L3Ms will comprise the foundation of this paradigm shift and serve as partners to human attorneys, providing expert support while the human attorneys retain overall control and responsibility (see Figure 1).
Nevertheless, the use of computational attorneys is not without its potential challenges and unintended consequences. The aforementioned requirements are fairly demanding; if current L3Ms do not meet them, additional safeguarding techniques will be necessary. We also need to ensure that L3Ms function as tools that assist—but do not replace—human attorneys. Instead of jeopardizing employment in the legal workforce, the automation of simple and complex legal tasks should enable legal professionals to serve a much wider customer base. Moreover, information from computational attorneys cannot compromise a lawyer’s legal and ethical responsibilities to provide accurate legal advice.
The legal jurisdictional systems in several regions have already begun to adapt to the transformations that result from generative AI technologies. In the U.S., judges have underscored an attorney’s duty to ensure the accuracy of their legal statements and the robustness of their legal reasoning in their briefs [8]. Meanwhile, the European Bar Association has published guidelines for best practices of lawyers in the era of ChatGPT. While ongoing research aims to establish the accountability of L3Ms, the ultimate responsibility lies with human attorneys to meticulously review and rigorously verify the outputs of computational attorneys.
All in all, the future of legal AI promises to advance the legal profession in a multitude of dimensions. With further research and development, we can anticipate the rise of computational attorneys that not only execute mundane, low-level legal tasks with superhuman performance, but also take on complex, high-level legal challenges. This outcome would revolutionize the legal industry, provide more efficient legal services, and democratize access to justice.
References
[1] Bench-Capon, T., Araszkiewicz, M., Ashley, K., Atkinson, K., Bex, F., Borges, F., … Wyner, A.Z. (2012). A history of AI and law in 50 papers: 25 years of the international conference on AI and law. Artif. Intell. Law, 20, 215-319.
[2] Casper, S., Davies, X., Shi, C., Gilbert, T.K., Scheurer, J., Rando, J., … Hadfield-Menell, D. (2023). Open problems and fundamental limitations of reinforcement learning from human feedback. Preprint, arXiv:2307.15217.
[3] Chalkidis, I., Fergadiotis, M., Mala-kasiotis, P., Aletras, N., & Androutsopoulos, I. (2020). LEGAL-BERT: The Muppets straight out of law school. In Findings of the association for computational linguistics: EMNLP 2020 (pp. 2898-2904). Association for Computational Linguistics.
[4] Christiano, P.F., Leike, J., Brown, T.B., Martic, M., Legg, S., & Amodei, D. (2017). Deep reinforcement learning from human preferences. In Advances in neural information processing systems 30 (NeurIPS 2017). Long Beach, CA: Curran Associates, Inc.
[5] Governatori, G., Bench-Capon, T., Verheij, B., Araszkiewicz, M., Francesconi, E., & Grabmair, M. (2022). Thirty years of artificial intelligence and law: The first decade. Artif. Intell. Law, 30, 481-519.
[6] Katz, D.M., Bommarito, M.J., Gao, S., & Arredondo, P. (2023). GPT-4 passes the Bar exam. Preprint, Social Science Research Network.
[7] Lewis, P., Perez, E., Piktus, A., Petroni, F., Karpukhin, V., Goyal, N., … Kiela, D. (2020). Retrieval-augmented generation for knowledge-intensive NLP tasks. In Advances in neural information processing systems 33 (NeurIPS 2020) (pp. 9459-9474). Curran Associates, Inc.
[8] Merken, S. (2023, June 26). New York lawyers sanctioned for using fake ChatGPT cases in legal brief. Reuters Legal. Retrieved from https://www.reuters.com/legal/new-york-lawyers-sanctioned-using-fake-chatgpt-cases-legal-brief-2023-06-22.
[9] Sartor, G., Araszkiewicz, M., Atkinson, K., Bex, F., van Engers, T., Francesconi, E., … Bench-Capon, T. (2022). Thirty years of artificial intelligence and law: The second decade. Artif. Intell. Law, 30, 521-557.
[10] Surden, H. (2014). Machine learning and law. Wash. Law Rev., 89(1), 87.
[11] Wei, J., Tay, Y., Bommasani, R., Raffel, C., Zoph, B., Borgeaud, S., … Fedus, W. (2022). Emergent abilities of large language models. Trans. Mach. Learn. Res.
[12] Yu, F., Quartey, L., & Schilder, F. (2023). Exploring the effectiveness of prompt engineering for legal reasoning tasks. In Findings of the association for computational linguistics: ACL 2023 (pp. 13582-13596). Toronto, Canada: Association for Computational Linguistics.
About the Authors
Frank Schilder
Senior Research Director, Thomson Reuters Labs
Frank Schilder is a senior research director at Thomson Reuters Labs, where he leads a team of researchers and engineers who explore new machine learning and artificial intelligence techniques to create smart products for legal natural language processing problems. Prior to joining Thomson Reuters, Schilder was an assistant professor in the Department of Informatics at the University of Hamburg.
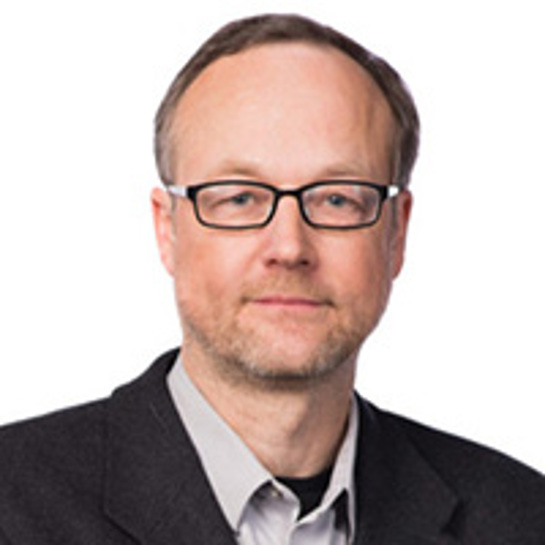
Dell Zhang
Research Lead, Thomson Reuters Labs
Dell Zhang currently leads the Applied Research team at Thomson Reuters Labs in London. He was formerly a tech lead manager at ByteDance AI Lab and TikTok UK; a staff research scientist at Blue Prism AI Labs; and a reader in computer science at Birkbeck, University of London.
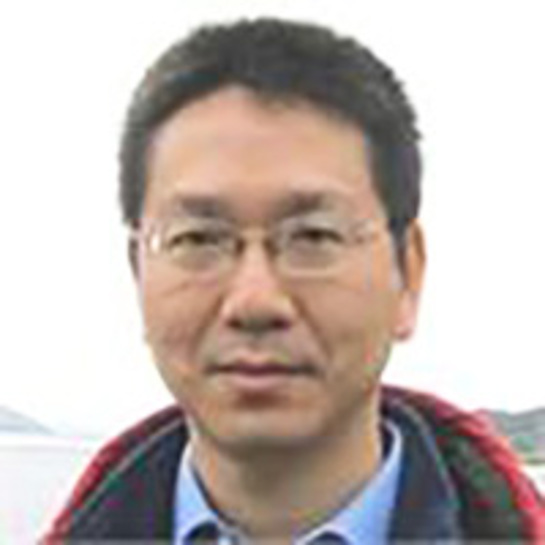
Stay Up-to-Date with Email Alerts
Sign up for our monthly newsletter and emails about other topics of your choosing.