Decoding Mobility Data in Disease Models: From Infection Dynamics to Health Economics
Human mobility on both short and long timescales greatly impacts disease dynamics and healthcare practices. Globalization and the growth of transportation networks have accelerated human mobility, leading to faster disease spread and a greater chance of major outbreaks. Patterns of movement and factors such as work schedules, seasonal employment, and the climate all affect healthcare spending, resource allocation, and the economic burden of public health crises.
While researchers developed numerous models to track viral spread during the COVID-19 pandemic, outstanding challenges that pertain to data reliability, standardization, and the integration of key metrics—especially human behaviors like population movement—prevent our complete understanding of disease dynamics. Our recent work systematically reviews these issues, summarizes mobility trends, and offers guidance on the selection of appropriate datasets and metrics for enhanced data-driven disease models [1].
Methods
We gathered several common metrics for the quantification of population movement based on three different sources: mobile phone data, community survey data, and transportation data. For example, the origin-destination metric from mobile phone data tracks local preferred regular movement within a city; community survey data reveals contact rates across age groups; and transportation data discloses intercity travel flux.
Our systematic literature review comprised five broad steps: (i) Evidence strategy, including protocol development that involved key search terms, search timeline, and database selection; (ii) the appraisal process, during which we designed predefined criteria for literature inclusion, exclusion, and quality assessment; (iii) real-world data synthesis, which involved the extraction and categorization of data from selected articles; (iv) evidence generation via the meta-analysis of synthesized data from multiple studies; and (v) the reporting of results according to internationally accepted Preferred Reporting Items for Systematic Reviews and Meta-Analyses guidelines [5]. Our literature search yielded 56 articles from Web of Science, PubMed, and Google Scholar that aligned with our predefined criteria in Figure 1.
![<strong>Figure 1.</strong> Criteria for the inclusion/exclusion of articles in our review [1]. Figure courtesy of the authors.](/media/u2hmfsbw/figure1.jpg)
Mobility Data Sources and Metrics
Human movement data from sources like public transit records, cell phones, and surveys provide insights into individual and population-wide mobility (see Figure 2). Researchers can filter this data based on their objectives to analyze changes in distinct mobility patterns over time; for instance, COVID-19 studies generally categorize movement data by generation method and intended use. Figure 3 outlines the creation of a mobility matrix based on different sources.
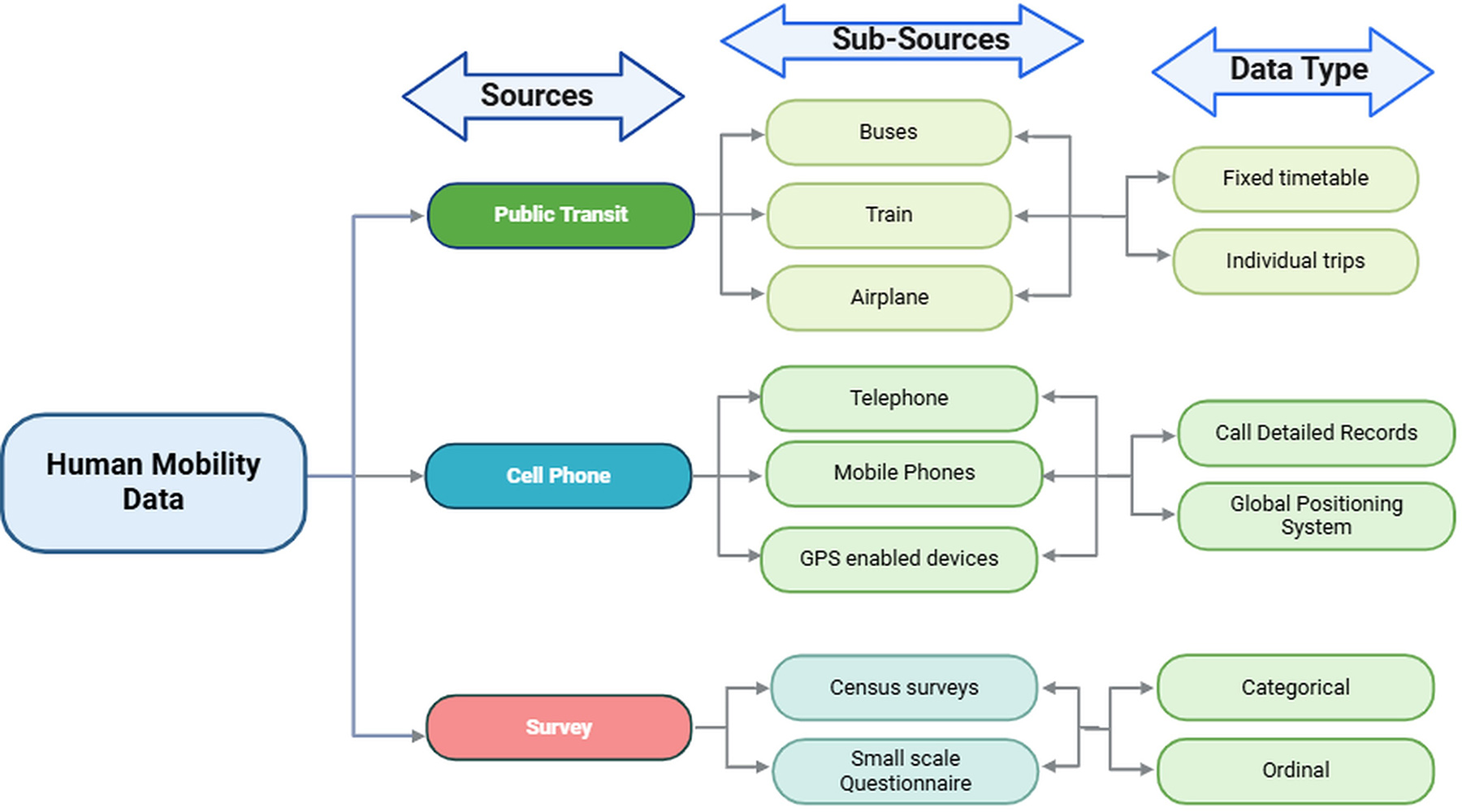
Factors That Influence Mobility
The COVID-19 pandemic significantly altered human mobility patterns on a global scale, as various direct and indirect factors shaped people’s movements within their environments.
Direct Factors: Health authorities imposed strict lockdowns and travel restrictions to limit the spread of COVID-19, initially causing significant reductions to human movement. Data from countries like Italy, Spain, and China revealed that mobility declined sharply in the beginning stages of the pandemic but quickly returned to pre-pandemic levels [8]. Early interventions included stay-at-home directives, border closures, school shutdowns, and travel bans. The severe social and economic impacts of these stringent measures led to the implementation of alternative strategies, such as evening curfews, weekend restrictions, and regulations for crowded public areas. The effectiveness of these strategies depended on individual behaviors. For instance, a 6 p.m. (rather than 9 p.m.) curfew in Greece did not significantly reduce essential activity mobility, but it did increase the time that citizens spent outside [9] — thus indicating that the limitation of certain behaviors can yield alternative actions. In Spain, the highest mobility fluctuations during the first lockdown occurred on Fridays, with the lowest fluctuations on Sundays [6]. Many countries imposed weekend curfews that resulted in minimal mobility fluctuations when compared to weekdays. Overall, human behavior—which is influenced by perceived risk—significantly impacted mobility during the COVID-19 pandemic. As such, we must always consider human behavior when implementing mobility restrictions and studying disease transmission.
Indirect Factors: Major indirect factors that affected movement during the pandemic include economic disparity, geographical disparity, and age-related mobility. COVID-19 disproportionately impacted marginalized communities, including religious, ethnic, and economically disadvantaged minorities — the latter of whom often had to continue to commute for work. Data from London and New York demonstrated that mobility in minority-dominated areas was higher than the national average [10]. On the other hand, regions with a more prominent senior population (defined as over 60 years old) saw the greatest decline in mobility. Noteworthy distinctions exist between countries as well, as overall mobility decreased more in Europe than in the U.S. Additionally, air traffic comparisons between India, Japan, and China during the pandemic’s early stages showed minimal movement in Japan with comparatively more activity in China and India [7]. All of these variations contributed to differing COVID-19 case trends.
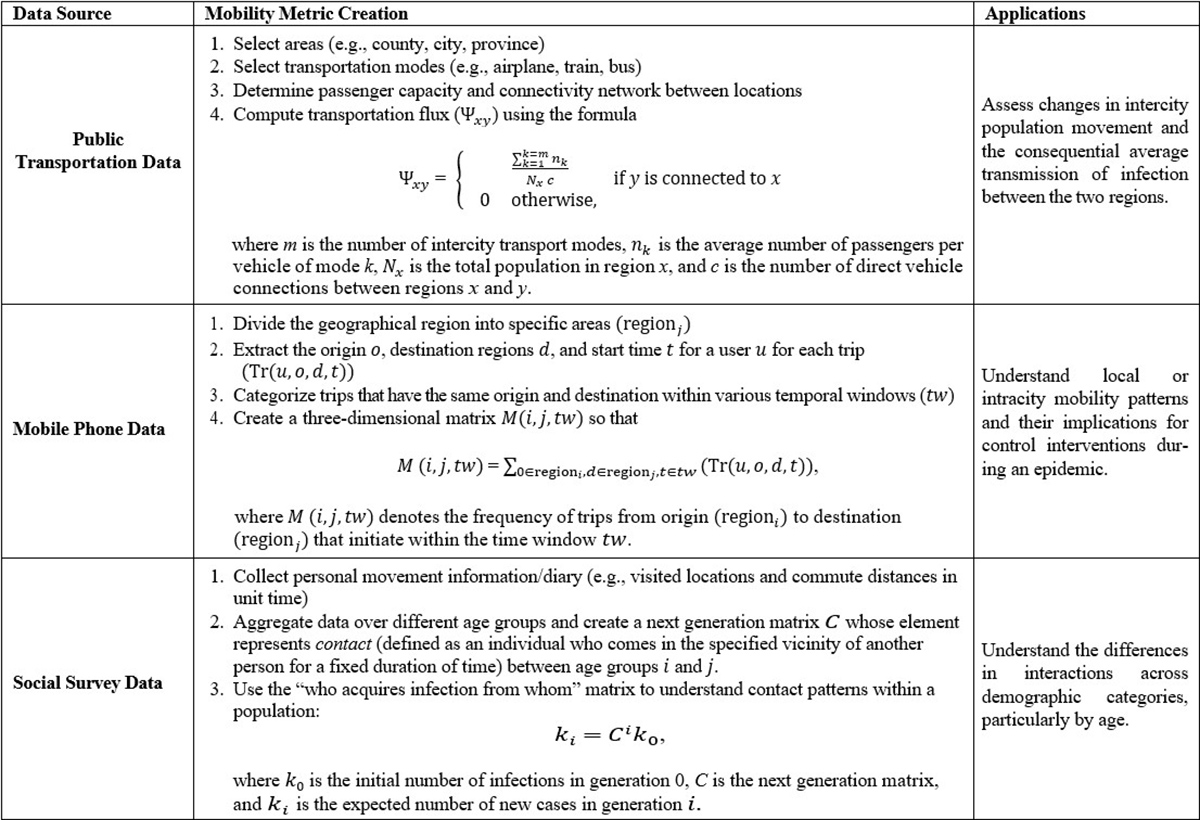
Mobility’s Impact on Health Economics
Mobility patterns allow policymakers to assess unmet needs, understand behaviors, and develop public health policies. By measuring mobility rates, decision-makers can tailor healthcare services to regional demands, identify treatment needs, and recognize determinants of social and behavioral health.
Mobility impairments affect physical health, mental health, and overall quality of life. Mathematical models can evaluate the effects of these improvements on both short-term health outcomes (e.g., functional status) and long-term economic outcomes (e.g., medical costs, nursing home use, and employment) [4]. Patient mobility may also reflect healthcare access, as individuals with inadequate local services often seek care outside of their own regions; preferences for specific hospitals can likewise reveal disparities in service quality. In the U.S. and some parts of Europe, patients can freely choose their own healthcare providers, which promotes competition.
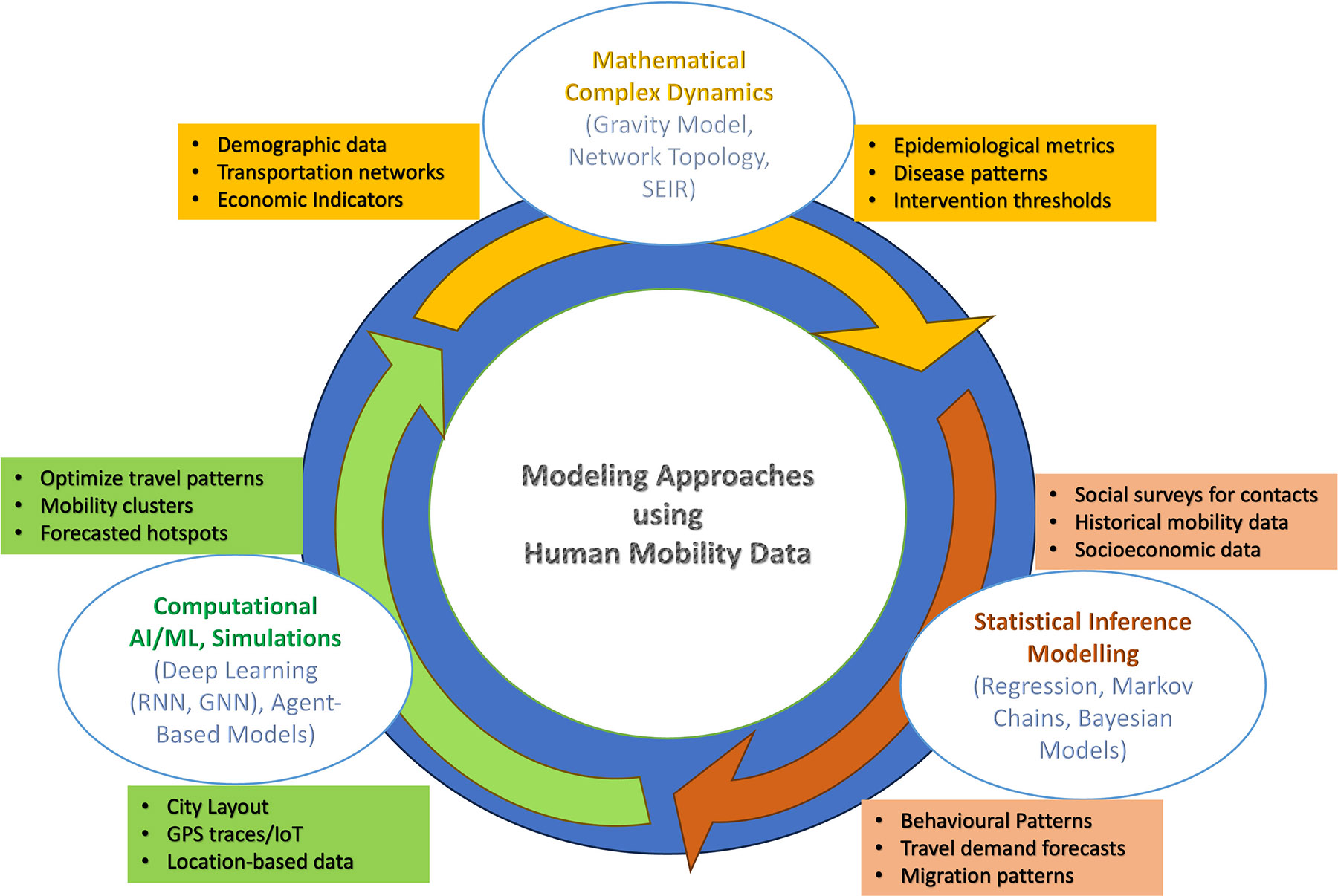
Several recent analyses divulged interesting results about health economics around the world. For instance, a 2018 study utilized mobility data—which comprised health service records that included the visit month and year, residence location, location of the health service facility, service provider, type of institution, and category of medical service—to identify medical regions in Turkey that matched those that were designated by the Ministry of Health, exposing discrepancies in service and planning [3]. And a 2022 study found that the distribution of specialists in Iran significantly affects patient mobility, highlighting the need to optimize the allocation of hospital beds and specialists [2].
Discussion
The continual evolution of human mobility data sources reveals ongoing challenges in their preparation for epidemiological modeling and the interpretation of results from their analysis. While traditional sources such as surveys and census data offer insights into regional movement patterns, they often lack the necessary granularity and real-time adaptability for dynamic disease modeling. To gain a more comprehensive understanding of disease spread, researchers are integrating mobile phone data, transportation records, and index-based metrics. However, this integration invokes other challenges that stem from the sheer volume of data and its availability, heterogeneity, and representativeness.
Here, we aimed to provide insights into human mobility data sources, the creation of mobility metrics, factors that influence disease transmission, and the standardization of real-time data collection — all of which significantly impact disease dynamics and health economics. By using these data sources as proxies for contact rates in infectious disease models (see Figure 3 and Figure 4), we demonstrate that mobility metrics can significantly enhance our ability to track, predict, and mitigate outbreaks. We thus urge researchers to strike a balance between traditional and modern mobility data and estimation methods to improve data accuracy and applicability.
Novel artificial intelligence (AI) techniques can also augment human mobility data analysis by processing vast amounts of diverse data and supporting real-time outbreak detection and response planning. AI-driven approaches can help address data sparsity, inconsistencies, and biases by identifying meaningful mobility patterns that may otherwise be overlooked. To better detect, mitigate, and manage current and future health challenges, we encourage scientists to adopt advanced modeling techniques and focus on enriching human mobility data through varied, modern sources and AI-powered analytics. Strengthening human mobility data infrastructures, ensuring ethical and privacy-conscious data usage, and promoting standardized methodologies will further enhance the role of mobility data in infectious disease research and public health decision-making.
References
[1] Bali, Y., Bajiya, V.P., Tripathi, J.P., & Mubayi, A. (2024). Exploring data sources and mathematical approaches for estimating human mobility rates and implications for understanding COVID-19 dynamics: A systematic literature review. J. Math. Biol., 88(6), 67.
[2] Hekmat, S.N., Haghdoost, A.A., Zamaninasab, Z., Rahimisadegh, R., Dehnavieh, F., & Emadi, S. (2022). Factors associated with patients’ mobility rates within the provinces of Iran. BMC Health Serv. Res., 22(1), 1556.
[3] Koylu, C., Delil, S., Guo, D., & Celik, R.N. (2018). Analysis of big patient mobility data for identifying medical regions, spatio-temporal characteristics and care demands of patients on the move. Int. J. Health Geogr., 17(1), 32.
[4] MacEwan, J.P., King, A.A., Nguyen, A., Mubayi, A., Agodoa, I., & Smith-Whitley, K. (2023). Cognition and education benefits of increased hemoglobin and blood oxygenation in children with sickle cell disease. PLOS One, 18(8), e0289642.
[5] Mikolajczyk, R.T., Akmatov, M.K., Rastin, S., & Kretzschmar, M. (2008). Social contacts of school children and the transmission of respiratory-spread pathogens. Epidemiol. Infect., 136(6), 813-822.
[6] Pérez-Arnal, R., Conesa, D., Álvarez-Napagao, S., Suzumura, T., Català, M., Alvarez-Lacalle, E., & Garcia-Gasulla, D. (2021). Comparative analysis of geolocation information through mobile-devices under different COVID-19 mobility restriction patterns in Spain. ISPRS Int. J. Geo-Inf., 10(2), 73.
[7] Ren, Z., Li, R., Zhang, T., Chen, B., Wang, C., Li, M., … Xu, B. (2021). Reduction of human mobility matters during early COVID-19 outbreaks: Evidence from India, Japan and China. Int. J. Environ. Res. Public Health, 18(6), 2826.
[8] Souch, J.M., Cossman, J.S., & Hayward, M.D. (2021). Interstates of infection: Preliminary investigations of human mobility patterns in the COVID-19 pandemic. J. Rural Health, 37(2), 266-271.
[9] Velias, A., Georganas, S., & Vandoros, S. (2022). COVID-19: Early evening curfews and mobility. Soc. Sci. Med., 292(1), 114538.
[10] Yechezkel, M., Weiss, A., Rejwan, I., Shahmoon, E., Ben-Gal, S., & Yamin, D. (2021). Human mobility and poverty as key drivers of COVID-19 transmission and control. BMC Public Health, 21(1), 596.
About the Authors
Yogesh Bali
Ph.D. student, Johannes Gutenberg University Mainz
Yogesh Bali is a Ph.D. student in the Department of Mathematics at Johannes Gutenberg University Mainz in Germany. His research focuses on the intersection of mathematics and biology, with a focus on mathematical immunology and disease modeling. Bali uses advanced mathematical techniques to understand the immune system and disease dynamics, bridging the gap between these fields and contributing to innovative global health solutions.
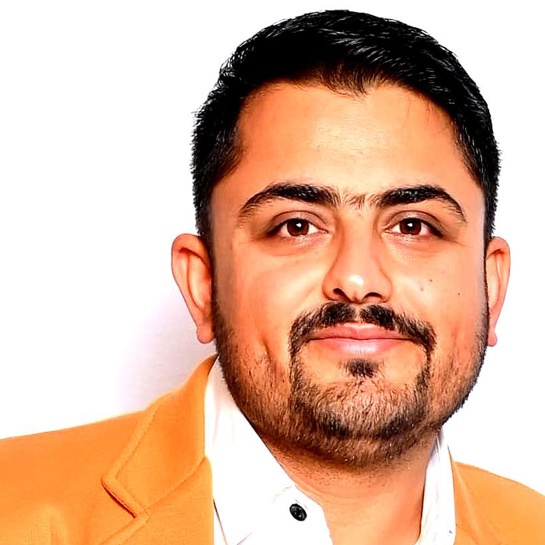
Jai Prakash Tripathi
Professor, Central University of Rajasthan
Jai Prakash Tripathi is a professor of mathematics at Central University of Rajasthan in India. His research interests include mathematical biology and nonlinear dynamics in the context of ecological modeling and mathematical epidemiology. Tripathi uses a variety of mathematical techniques to study the nonlinear features of ecosystems and disease models, including stability, resilience, robustness, transient dynamics, bifurcations, periodicity, and oscillations.
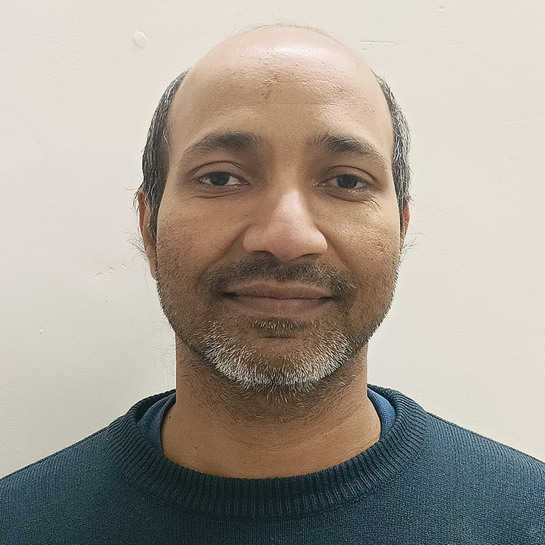
Anuj Mubayi
Fellow in Residence, Intercollegiate Biomathematics Alliance
Anuj Mubayi is a Fellow in Residence for the Intercollegiate Biomathematics Alliance, which is housed at Illinois State University; an honorary fellow at the Kalam Institute of Health Technology in India; an adjunct faculty member in the Department of Mathematics and Computer Science at the Sri Sathya Sai Institute of Higher Learning in India; and a scientific advisor for Kalam Experts, a healthcare consulting company. Mubayi previously served as director of the Mathematical and Theoretical Biology Institute’s Research Experiences for Undergraduates program. His expertise lies in health decision science and encompasses health economics, data-driven disease modeling, and real-world evidence generation.
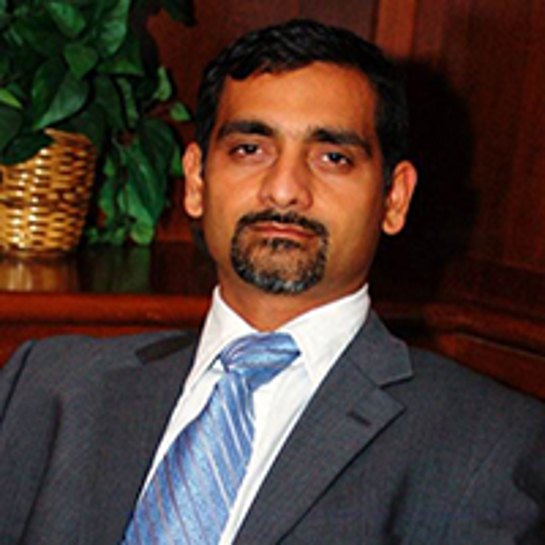
Stay Up-to-Date with Email Alerts
Sign up for our monthly newsletter and emails about other topics of your choosing.