Environmental Impacts of Artificial Intelligence: Peril, Promise, and Policy
Over the last decade, the amount of computational power (or “compute”) that is required to train cutting-edge artificial intelligence (AI) systems has escalated rapidly [8, 9]. As applications of AI become increasingly common in everyday life, the general public is growing more aware of the environmental costs that are associated with these technologies. Data centers that house the necessary computational resources to train and operate these systems require tracts of land to sit upon, water to cool the servers, and significant amounts of energy to sustain operation (see Figure 1). As the race towards ever-more powerful AIs continues, these demands will only become steeper. In response, the Artificial Intelligence Environmental Impacts Act of 2024 was introduced in U.S. Congress earlier this year to address both AI’s environmental detriments and potential benefits.
Resource Consumption of AI
The Act specifically references the findings of a 2022 Epoch AI study, which concluded that the amount of compute that major corporations used to train large-scale AI models doubled every 10 months between 2015 and 2022 [8]. Earlier this year, Epoch AI updated these findings based on three times more data [9] and concluded more broadly that compute for notable and frontier models has been growing at a rate of around four to five times per year (see Figure 2). The Act cites concerns that as these demands continue to escalate, the associated rapid growth in data center infrastructure (such as cooling systems and power equipment) and the manufacturing processes to support them will further contribute to pollution, heightened water consumption, changes in land use, and electronic waste.
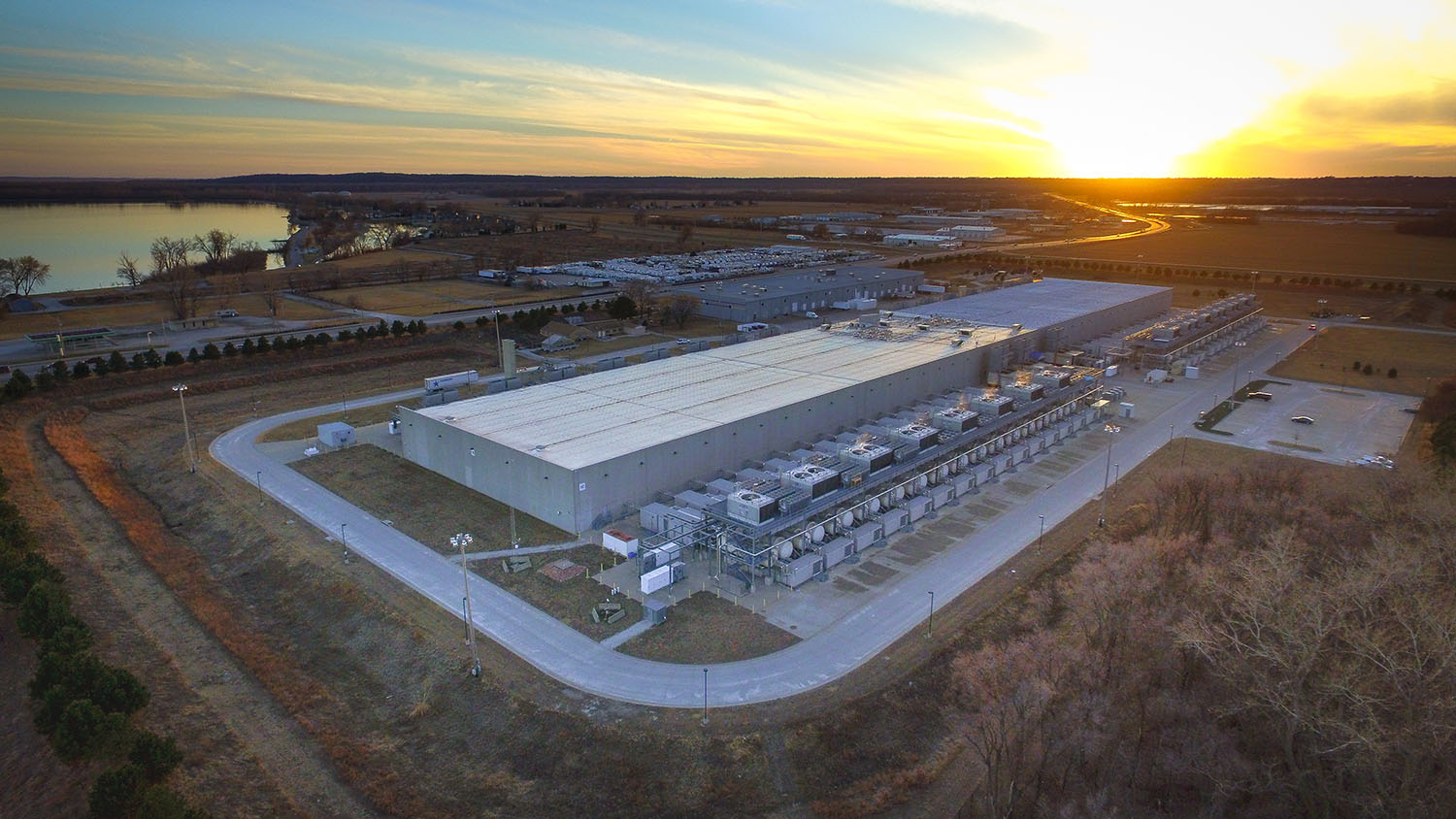
According to the International Energy Agency, data center energy usage increased from roughly 200 terawatt-hours (TWh) in 2015 to between 240 and 340 TWh in 2022 (approximately one percent of the global final electricity demand). As a point of comparison, all U.S. healthcare facilities consumed a combined total of only 170 TWh of energy in 2018. The energy demand of each individual AI varies; for instance, Hugging Face claims that it utilized 433 megawatt-hours (MWh) of energy to train its BigScience Large Open-science Open-access Multilingual (BLOOM) language model, while GPT-3 reportedly used 1,287 MWh for training [2].
Overall, more research has focused on the energy demands for training AI systems than the demands during the inference phase, when the trained AI become available for consumer use to answer queries or perform other tasks [2]. One analysis suggests that the operation of ChatGPT requires 564 MWh per day [2] — a substantial amount when compared to its training run. Furthermore, Google reported that 60 percent of its AI-related energy consumption between 2019 and 2021 stemmed from inferences [2]. However, these figures do not necessarily seem consistent across the board. For instance, Hugging Face’s BLOOM model appears to consume significantly less energy during inference than it did during the training phase, which would indicate that energy demands vary widely across individual models.
On a local level, even a single data center can have a large impact on the surrounding area. For instance, the water requirements to cool data centers in Arizona further strain the already burdened resources of desert cities, and large tech companies make it difficult for journalists or concerned local citizens to access concrete information about their water and power usage [4]. Growing concerns about the construction of new data centers and their supporting infrastructure have prompted grassroots protests around the country. For example, protests throughout Maryland have centered on a proposed power transmission line that would run through the state from a nuclear power plant in Pennsylvania to the data center hub in northern Virginia [5]. Citizens are generally concerned about the possible loss of their property to data center projects, the strain on existing electrical grids, and overall land conservation; in contrast, data center proponents claim that the larger properties on which these facilities are housed could potentially conserve undeveloped areas.
Alternative Energy and Data Centers
Renewable energy is a feasible source of power for AI infrastructure. In fact, the International Energy Agency lists Amazon, Microsoft, Meta, and Google—all major companies in the AI race—as the four largest buyers of corporate renewable energy power purchase agreements. In 2021, these companies matched 100 percent of their operational electricity consumption with the purchase or generation of renewable electricity. This is certainly a striking figure, but the details are a bit more complex. Data centers and transmission networks are not necessarily completely powered with renewable sources; given the need to operate this infrastructure both day and night, gaps in renewable energy supply are often filled by fossil fuels. Though companies then offset this fossil fuel usage with renewable energy purchases, doing so cannot negate the harmful emissions that were already produced [1].
There is also great interest in powering data centers with nuclear energy, since nuclear power plants can operate constantly and are friendlier to the climate. It was recently announced that Three Mile Island—a nuclear power plant in central Pennsylvania that famously played host to a major nuclear accident in 1979—will reopen to power Microsoft data centers [6]. While continued technological advances have improved the safety of nuclear facilities, this news still stoked mixed reactions among Pennsylvania residents.
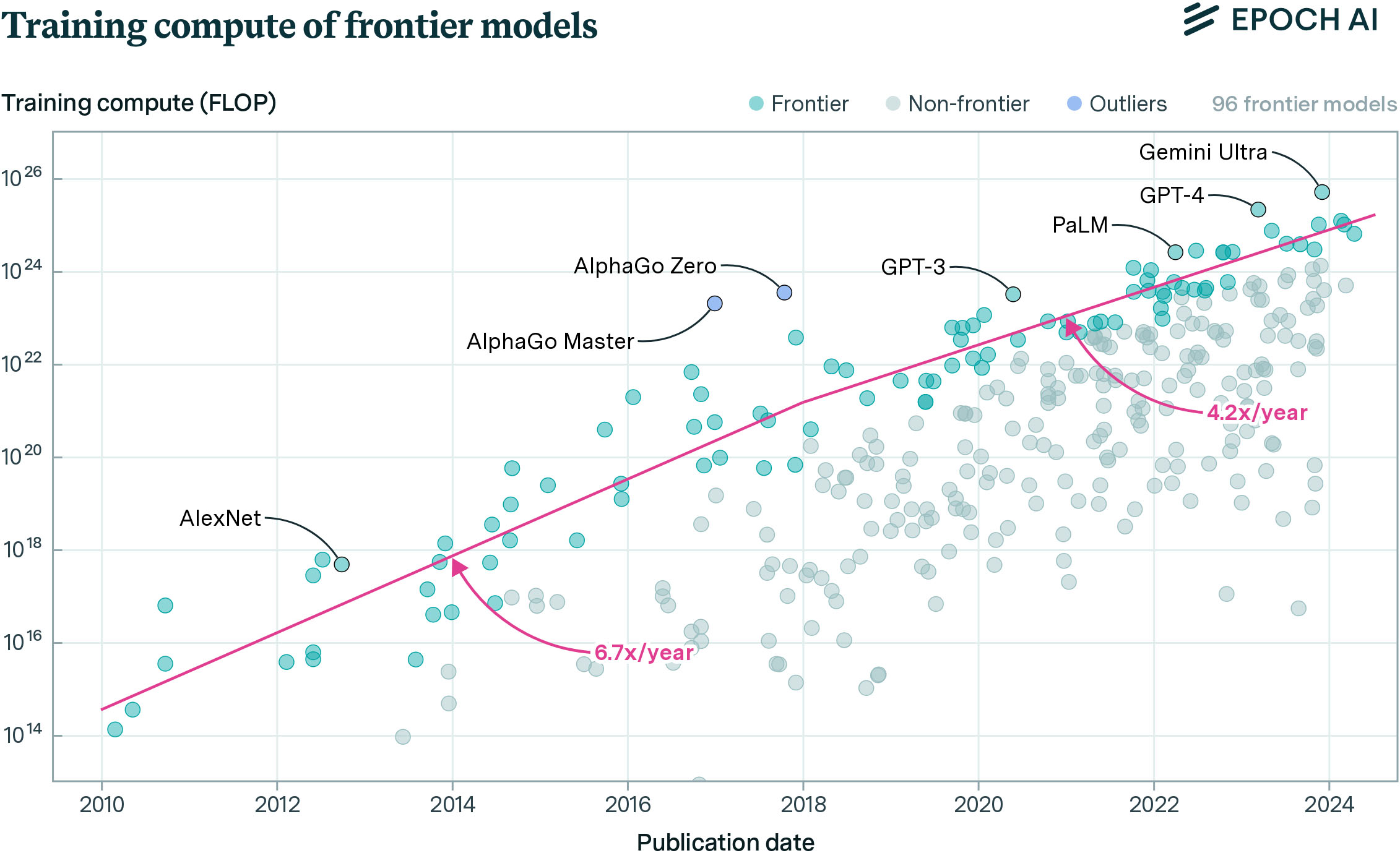
Potentially Beneficial Applications of AI
In addition to raising concerns about AI’s resource consumption, the Artificial Intelligence Environmental Impacts Act of 2024 also seeks to encourage the application of AI for direct or indirect environmental benefit. For instance, AI can help to optimize energy efficiency, spur the development of renewable technologies, and improve environmental monitoring. However, the Act also recognizes that AI applications in industry can equivalently impose negative environmental impacts—e.g., through fossil fuel production—or encourage environmentally detrimental behaviors like hyper-consumption.
Many members of the SIAM community are involved in the pursuit of beneficial AI applications, like the use of machine learning to forecast wildfires, improve Earth system models, and accelerate weather simulations. Furthermore, an organization called Climate Change AI comprises researchers from academia and industry who specifically aim to address climate change via machine learning. In a 2022 report [7], members of Climate Change AI identified numerous high-impact problems wherein AI developers could collaborate with experts in other domains to fill gaps in knowledge or capabilities.
Prospective Policy
On February 1, 2024, a group of Democratic lawmakers introduced the Artificial Intelligence Environmental Impacts Act of 2024 [3]. The Act proposes several actions that would address AI and the environment. First, it would mandate the U.S. Environmental Protection Agency (EPA) to study AI’s climate impacts and submit a comprehensive report to Congress within two years. This study would address a variety of factors, including pollution, energy and water consumption at data centers, acute impacts at local scales, positive and negative effects of AI applications, and the potentially unequal distribution of these effects throughout the population.
Moreover, the National Institute of Standards and Technology (NIST) would be directed to convene a consortium of stakeholders from academia, industry, and civil society to identify methods for measuring and reporting on AI’s environmental impacts. The consortium would aim to facilitate trustworthy reporting, develop open-source software and hardware tools, and provide recommendations for promoting positive environmental impacts of AI and mitigating negative ones. NIST would also be tasked with creating a voluntary and publicly available reporting system on AI models’ climate impacts.
Finally, within four years of the legislation’s enactment, the Department of Energy, EPA, and NIST would be required to submit a joint report to Congress about the consortium’s findings, details of the new reporting system, and recommendations for future legislative or administrative actions [3].
Outlook
After lawmakers introduced the Artificial Intelligence Environmental Impacts Act to the Senate this February, it was referred to the Committee on Commerce, Science, and Transportation. The public has not seen much movement since then, so its future is unclear. If ultimately passed, the Act will provide more clarity on the past, present, and future environmental costs of AI development and its disparate effects on different regions and communities. The promotion of transparent reporting and environmental protection measures could ultimately serve to safeguard the future of both people and the environment in the face of rapid technological development.
Jillian Kunze prepared this article as part of the final project of the AI Safety, Ethics and Society Course that ran from July to October 2024 through the Center for AI Safety.
References
[1] de Chalendar, J. (2019, December 2). Why ‘100% renewable energy’ pledges are not enough. Financial Times. Retrieved from https://www.ft.com/content/d75f49d0-103f-11ea-a225-db2f231cfeae.
[2] de Vries, A. (2023). The growing energy footprint of artificial intelligence. Joule, 7(10), 2191-2194.
[3] Heilweil, R. (2024, February 1). Democratic lawmakers propose legislation to study AI’s environmental impacts. Fedscoop. Retrieved from https://fedscoop.com/democratic-lawmakers-propose-legislation-to-study-ais-environmental-impacts.
[4] Kim, S.E. (2024, May 28). Karen Hao combats company stonewalling about AI’s resource demands. The Open Notebook. Retrieved from https://www.theopennotebook.com/2024/05/28/karen-hao-combats-company-stonewalling-about-ais-resource-demands.
[5] Kurtz, J. (2024, August 30). Data center conference gets caught up in power line controversy. Maryland Matters. Retrieved from https://marylandmatters.org/2024/08/30/data-center-conference-gets-caught-up-in-power-line-controversy.
[6] Mandler, C. (2024, September 20). Three Mile Island nuclear plant will reopen to power Microsoft data centers. NPR. Retrieved from https://www.npr.org/2024/09/20/nx-s1-5120581/three-mile-island-nuclear-power-plant-microsoft-ai.
[7] Rolnick, D., Donti, P.L., Kaack, L.H., Kochanski, K., Lacoste, A., Sankaran, K., … Bengio, Y. (2022). Tackling climate change with machine learning. ACM Comput. Surv., 55(2), 42.
[8] Sevilla, J., Heim, L., Ho, A., Besiroglu, T., Hobbhahn, M., & Villalobos, P. (2022). Compute trends across three eras of machine learning. In 2022 international joint conference on neural networks (IJCNN) (pp. 1-8). Padua, Italy: Institute of Electrical and Electronics Engineers.
[9] Sevilla, J., & Roldán, E. (2024, May 28). Training compute of frontier AI models grows by 4-5x per year. Epoch AI. Retrieved from https://epochai.org/blog/training-compute-of-frontier-ai-models-grows-by-4-5x-per-year.
About the Author
Jillian Kunze
Associate editor, SIAM News
Jillian Kunze is the associate editor of SIAM News.
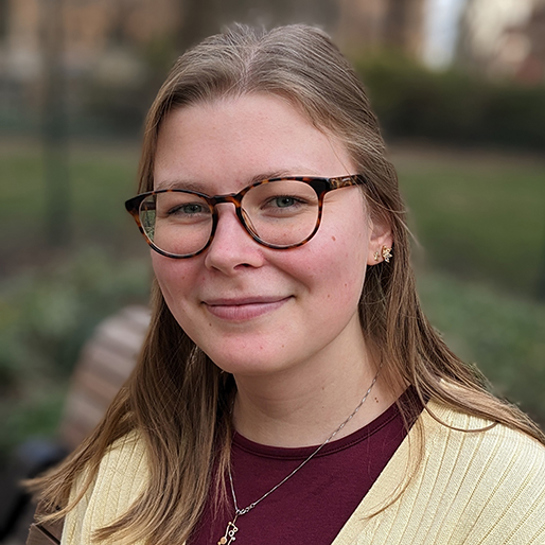
Stay Up-to-Date with Email Alerts
Sign up for our monthly newsletter and emails about other topics of your choosing.