Feeling Lucky? The Relative Roles of Skill and Chance in Sports
As I wrote this article, I was watching the televised women’s team archery gold medal competition at the 2024 Summer Olympics in Paris. I had never watched team archery before, and I was riveted. The captivating suspense—a signature of all great sports competitions—arises in part from the delicate balance between skill and chance. As spectators, we prize excellence and want highly skilled athletes to be rewarded for their efforts; however, we also love to root for an underdog. It is this tension between supremacy and uncertainty that makes sports so compelling.
The outcomes of competitions—athletic or otherwise—and many other activities are determined by a blend of skill and luck. A variety of factors impact the relative importance of these two effects, including rules and regulations and physical and biological considerations. For example, you likely arrived safely at your destination when you last drove to the office. Though this result is largely due to driving skill, you were also lucky that no other cars collided with you. If you live in an area where car accidents are relatively uncommon, then driving to work lies near the skill end of the skill-luck spectrum.
During the early 2000s, the rise of online poker and the accompanying legal debate1 heightened mathematicians’ collective interest in the estimation of different activities’ placements on this spectrum. In a 2013 paper, Thomas Miles, Steven Levitt, and Andrew Rosenfield proposed a series of mathematical questions to assess the role of chance in the outcome of poker tournaments [2]. An appealing aspect of their framework is that users can formulate the questions purely in terms of inputs (player or team actions) and outputs (win-loss records). We can hence view the game itself as a black box, which eliminates the need to articulate the detailed mathematics that are associated with the rules.
Here, I will focus on a particular question that explores the persistence of skill as a metric for the placement of activities on the skill-luck spectrum [2]. Roughly speaking, if someone is good at a skill-based activity today, they will likely be good at it tomorrow. In contrast, the result of a chance-based activity—e.g., coin flipping—on one day is in no way indicative of future outcomes.
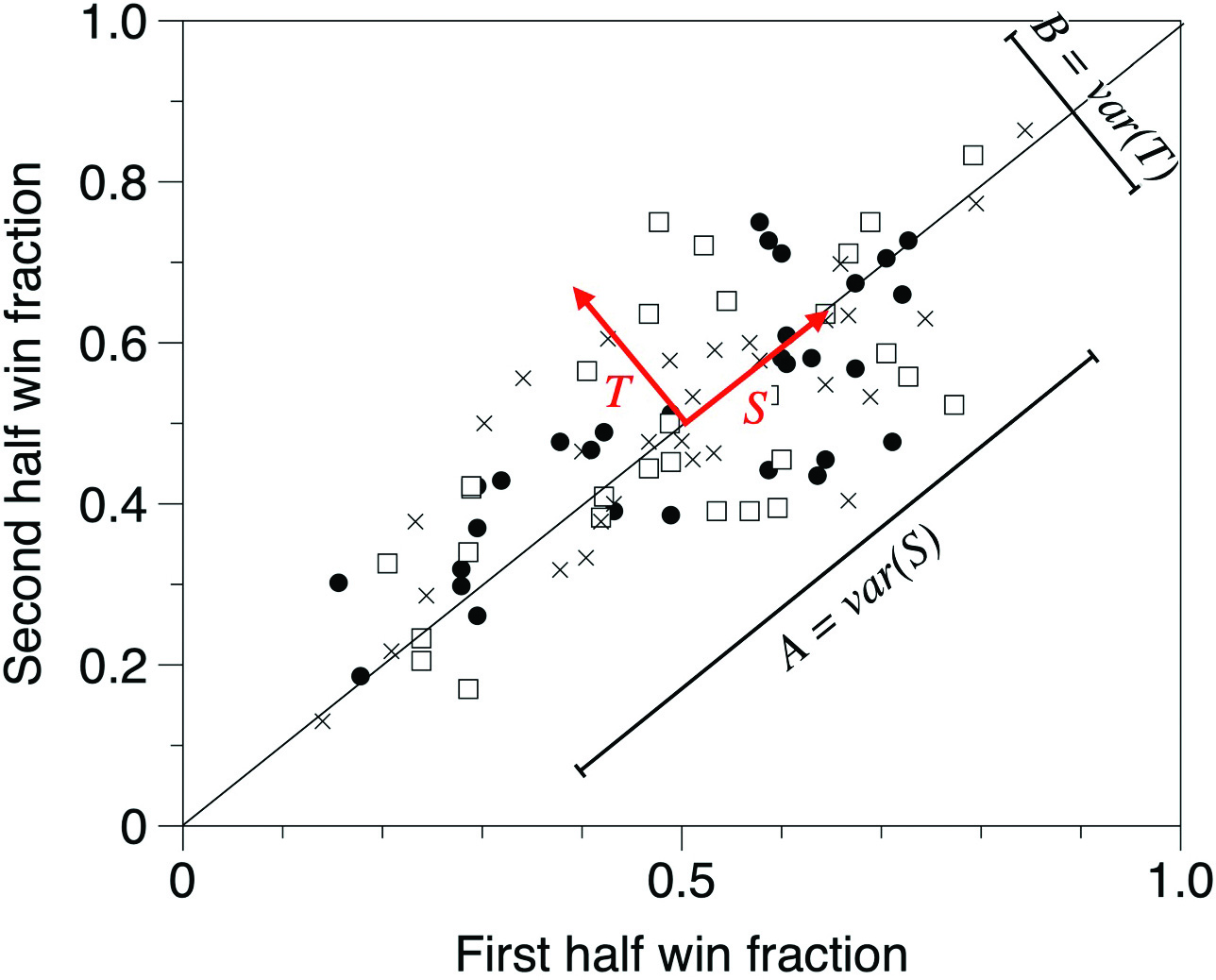
In a 2018 SIAM Review article, my collaborators and I proposed a metric to quantify the persistence of skill that begins with the following hypothesis [1]: Skill is an intrinsic quality of an athlete or team and does not change significantly over the course of a season. If this is true, in games of skill we expect the win fraction of each player or team in the first half of the season to correlate with that player or team’s win fraction in the second half. To intuitively understand the signatures of skill and luck within this framework, consider an idealized scenario wherein an infinite number of players are playing an infinite number of games. In contests of pure luck (i.e., coin flipping), the anticipated outcome of every player is the same. If we thus plot each player’s first-half win fraction against their second-half win fraction—assuming a zero-sum game in which participants compete against one another—all players converge to a single point at \((1/2, 1/2)\). But in a skill-dominated contest, we expect to observe a distribution of skill across the playing population; for instance, players who win 80 percent of their games in the first half of the season should also win 80 percent in the second half. In this case, the data will converge to a line with slope \(1\).
In reality, however, we have neither infinite players nor infinite games. As such, we anticipate the presence of scatter in the data — even in the extremes of all-skill or all-luck settings. And because neither skill nor luck exclusively determine the outcomes of most activities, the data will likely resemble an elongated cloud; in this format, higher levels of elongation correspond to the increasingly large role of skill in influencing the outcome.
Figure 1 illustrates five years of National Basketball Association (NBA) data, where each point represents one team’s win-loss record in a single season. To quantify the relative importance of skill, we must determine the point cloud’s degree of elongation. To do so, we rotate the coordinate system by \(\pi/4\) so that it becomes an \(S\)-\(T\) coordinate system (shown in red in Figure 1) and consider the ratio of the variance in the \(T\) direction versus the variance in the \(S\) direction. Specifically, we define
\[R=1-B/A,\]
where \(A\) is the variance along \(S\) and \(B\) is the variance along \(T\). For an outcome that is primarily determined by skill, \(B \rightarrow 0\) and \(A \rightarrow\) a finite number; therefore, \(R \rightarrow 1\). For an outcome that is primarily determined by luck, the point cloud approaches a symmetric blob — meaning that \(B \rightarrow A\) and \(R \rightarrow 0\).2 Note that this analysis does not judge the difficulty level of the sport in question (e.g., we do not claim that basketball requires more skill than hockey). Rather, we measure whether the rules of the game or tournament are designed to reward skill; at the end of the season, are the more skilled players or teams ranked the highest, or is the order random?
Armed with this metric, we can calculate the \(R\) value for different sports and place them on a skill-luck spectrum. Figure 2 displays \(R\) values for 10-year spans of five professional sports leagues: the NBA from 2008 to 2018 (excluding 2011), Premier League soccer from 2012 to 2021, Major League Baseball (MLB) from 2012 to 2021, the National Hockey League (NHL) from 2007 to 2017 (excluding 2013), and the National Football League (NFL) from 2014 to 2023.3 The plot suggests a possible “sweet spot” for sports that balances the roles of skill and chance (indicated by the gray box). In this regime, talent is rewarded but surprises are possible and underdogs can still prevail.
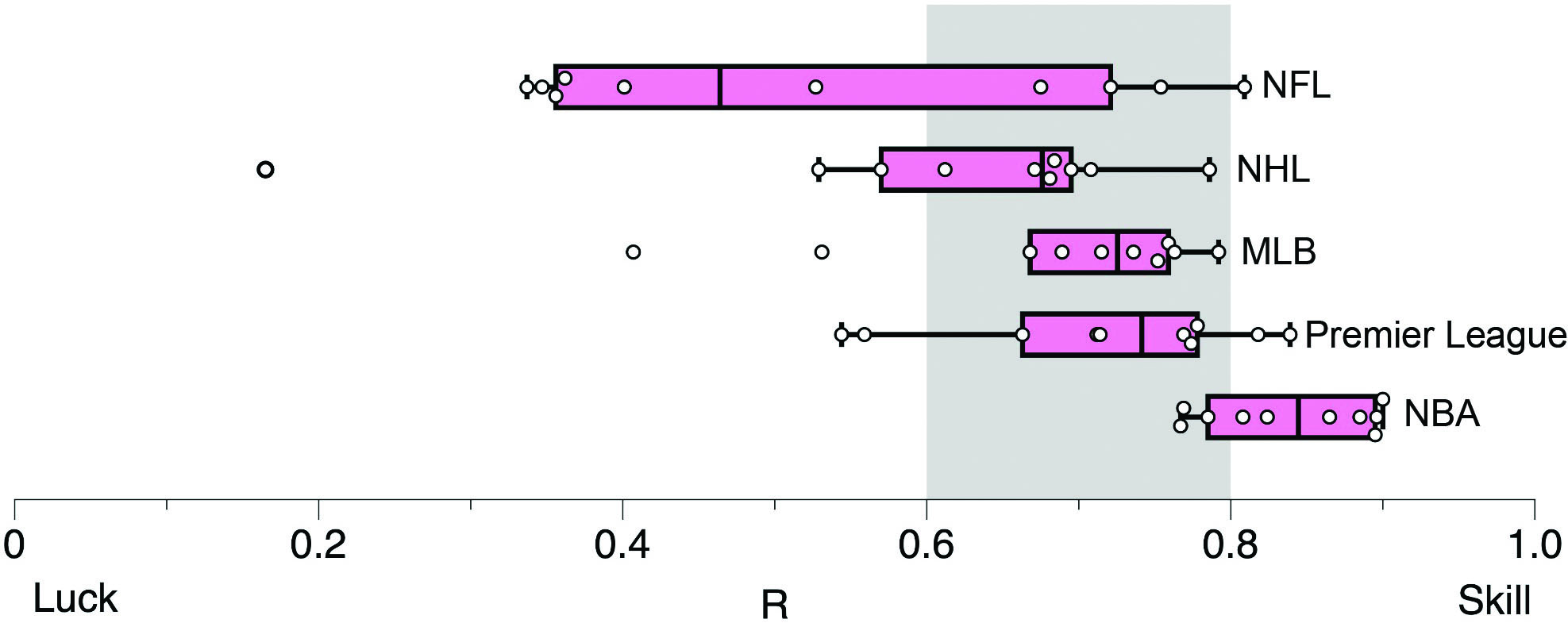
If a league’s objective is to hit this sweet spot and make the game more compelling to fans, several strategies can nudge competitions into this region of the spectrum. For instance, leagues could adjust the number of scoring opportunities, as “even tiny differences in skill manifest themselves in near certain victory if the time horizon is long enough” [2]. If two competitors are separated by a small amount of skill, the lesser player may win a single game but will struggle to prevail in four out of seven games, for example. Increasing the number of games and/or the scoring opportunities per game increases the odds that the more skilled player will triumph.
To confirm this strategy’s consistency with the previously computed \(R\) values, Figure 3 summarizes the number of scoring opportunities per season for each of the five leagues. As expected, more “scores” per season per team shifts the location of the league towards the skill side of the skill-luck spectrum.
The Premier League is the sole exception to this trend, which suggests a second strategy to adjust location on the skill-luck spectrum: concentrate or diffuse the distribution of talent. Consider the aforementioned Olympic archery championship. If I were to participate in the competition, my arrow would likely not land anywhere near the target; the outcome of the contest would be a foregone conclusion (my loss), and skill—or lack thereof—would be the primary factor in determining the winner. But in the elite faceoff that I witnessed, the differences in skill are so slight that the timing of a random gust of wind could serve as a key differentiator. The role of skill in an outcome thus depends on the distribution of talent in the playing population.
This distribution can be adjusted with rules and regulations that allow or constrain the concentration of talent — e.g., by employing a salary cap, weighting draft order inversely with performance, or dividing tournaments into classes of different skill levels. Of the five leagues that we considered, the Premier League is the only one that does not have a salary cap. Given the number of scoring opportunities in soccer, one might expect the Premier League to sit closer to the luck end of the spectrum. However, the lack of salary cap enables the concentration of skill in wealthier teams and shifts the league back towards the skill end of the spectrum so that it falls solidly within the desirable region.4
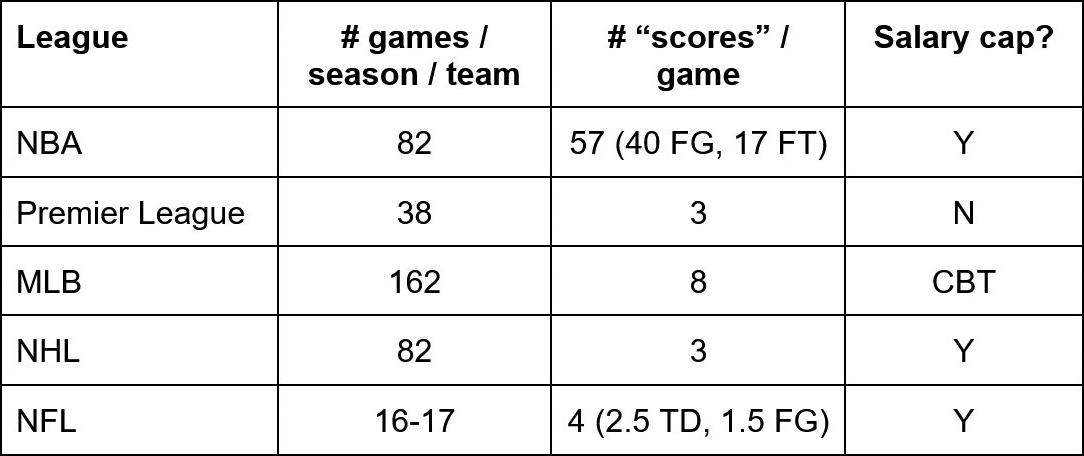
Finally, a third strategy to manipulate the relative role of skill versus luck involves adjusting the equipment or physical environment of the game. In the 2017 MLB season, a striking increase in the rate of home runs corresponded with a decrease in the average drag of MLB baseballs. Fans may recall the “juiced” ball debate and the accompanying outcry from spectators and players alike. Interestingly, this decrease in drag coefficient was small compared to the natural variance in drag among baseballs. A beautiful graphic from Baseball Savant illustrates both the variance in drag for each season and the change in these distributions from year to year. Because modern-day baseballs are still hand stitched and made of natural materials, much of this inconsistency is inevitable. Given that miniscule changes in the ball’s surface can yield significant variations in home run rate, an ongoing debate pertains to the possible integration of modern materials and manufacturing techniques to decrease variance across baseballs.
But in light of MLB’s current placement on the skill-luck spectrum, should the balls be more uniform? After all, the randomness that is associated with a high- or low-drag ball is an intrinsic component of each at-bat. Removing that variance would push MLB further towards the skill end of the spectrum, and it is unclear whether such a shift would benefit the game.
When I turned back to my television, the Olympics had moved on to diving — the outcome of which seems to be largely determined by skill (the variance of talent across athletes is large due to the dominance of the Chinese divers). The competition showcased exquisite physical capabilities of extraordinary athletes. But it captivated my attention in a different way than the archery competition, which had an element of tension driven by uncertainty. So, I will end by celebrating this uncertainty. The creation of contests that are compelling to watch is ultimately an optimization problem that must strike the desired balance between determinism and chance. And if leagues perform this calculation correctly, we can all join the throngs of sports fans who support underdogs and believe that “maybe this year will be different.”
1 If a game’s outcome is determined predominantly by chance, then the activity is typically classified as gambling and subject to laws such as the Unlawful Internet Gambling Enforcement Act of 2006.
2 We originally performed this analysis as part of a legal argument in The People of the State of New York v. FanDuel, Inc. [3]. In that case, we defined \(R=1-B/A\) (rather than \(R=B/A\)) because the lawyers suggested that it is more intuitive to associate \(1\) with skill and \(0\) with luck.
3 We omitted the 2011 NBA and 2013 NHL seasons because lockouts resulted in significantly fewer competitions.
4 The Premier League will implement a salary cap for the 2025-2026 season, thus providing a beautiful natural experiment of the salary cap’s role in setting the skill-luck balance.
References
[1] Getty, D., Li, H., Yano, M., Gao, C., & Hosoi, A.E. (2018). Luck and the law: Quantifying chance in fantasy sports and other contests. SIAM Rev., 60(4), 869-887.
[2] Miles, T.J., Levitt, S.D., & Rosenfield, A.M. (2013). Is Texas hold ’em a game of chance? A legal and economic analysis. Georgetown Law J., 101(3), 581-636.
[3] The People of the State of New York v. FanDuel, Inc. (2015). New York County Clerk’s Index No. 453056/15. Retrieved from https://www.legalsportsreport.com/wp-content/uploads/2016/02/2015_453056_People-v.-FanDuel_FanDuel_appbrief-1.pdf.
About the Author
Anette Hosoi
Professor, Massachusetts Institute of Technology
Anette (Peko) Hosoi is the Neil and Jane Pappalardo Professor of Mechanical Engineering and a professor of mathematics at the Massachusetts Institute of Technology (MIT). She is also co-founder of the MIT Sports Lab, which connects the MIT community with professional teams and leagues to address data and engineering challenges in the sports domain.
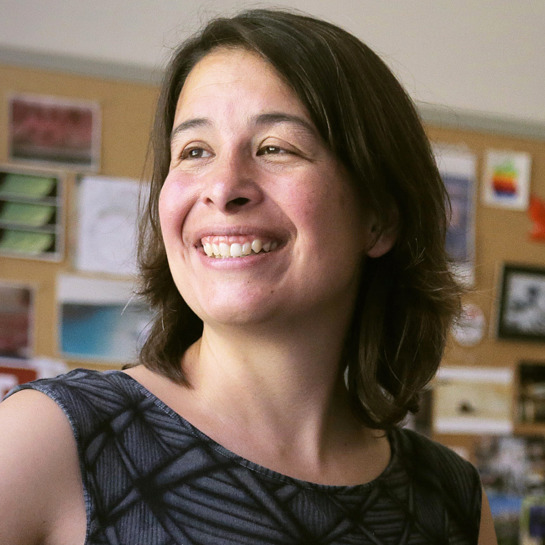
Related Reading
Stay Up-to-Date with Email Alerts
Sign up for our monthly newsletter and emails about other topics of your choosing.