Forward-looking Panel at CSE25 Contemplates Future Directions of Computational Science and Engineering
The field of computational science and engineering (CSE) continues to evolve at a rapid pace, driven by novel computer architectures and advancements in artificial intelligence (AI), machine learning (ML), and other technologies. This interdisciplinary research area unites theory and experimentation to stimulate scientific discovery in a variety of domains, from healthcare and supply chain logistics to transportation and defense. As such, practitioners must ensure the safe deployment and explainability of AI tools; enable the analysis, visualization, and assimilation of large data sets; and promote the design of scalable, flexible, and robust software.
During the 2025 SIAM Conference on Computational Science and Engineering, which took place in Fort Worth, Texas, this past March, a forward-looking panel anticipated future challenges and opportunities for CSE in an ever-changing world. Orly Alter of Prism AI Therapeutics and the University of Utah organized the panel and moderated an engaging conversation between Raymond Honfu Chan of Lingnan University in Hong Kong; Gilles Gnacadja of Amgen; Jungsang Kim of Duke University; and Boris Kramer of the University of California, San Diego (UCSD). The panelists each delivered a brief presentation that outlined their observations and experiences across both academia and industry, after which they collectively discussed potential future directions for CSE within the larger context of science, technology, engineering, and mathematics (STEM).
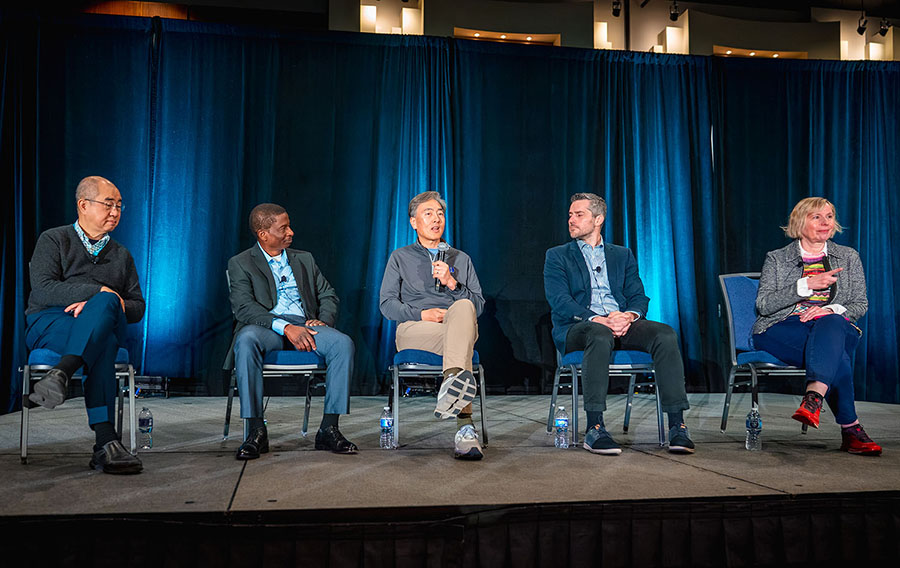
As a professor of scientific computing at Lingnan University, Chan is deeply familiar with the association between STEM-based curricula and the broader research space. Over the years, he has witnessed the development of increasingly sophisticated methods that necessitate novel mathematical models with improved features and more extensive applicability. Prior to the widespread adoption of AI, this evolution usually occurred fairly gradually, which facilitated the organic incorporation of new methods into educational tracks. But as AI expedites the analysis, prediction, and optimization of complex systems, the structure of Ph.D.-level university programs must adapt more rapidly.
Chan asserted that AI-era Ph.D. programs can certainly still emphasize some of the same core mathematical topics as traditional curricula, such as numerical linear algebra, optimization, and ML. But unlike conventional modules that concentrate predominantly on only one method or application, current and future programs should explore the adaptation and mixing of existing models — with a focus on multidisciplinary, diverse applications that reflect the changing occupational landscape. This shift is especially important because recent years have seen Ph.D.-level graduates move away from academia in favor of industry positions that often offer higher salaries than traditional academic posts.
To prepare students for newly emerging industry opportunities, Lingnan University’s Ph.D. curriculum underscores interdisciplinary fluency, verification and validation, programming skills, the relationship between ethics and society, the integration of AI and ML, and the “4 Cs” — communication, collaboration, critical thinking, and creativity. A core first-year AI course explores the ethics, values, and proper use of AI, and subsequent courses center on automated reasoning, policy, and risk within the AI framework. “AI is ubiquitous,” Chan said. “We want [the students] to use it properly, and we want to teach them about the humanistic aspects of AI.”
Like Chan, Kramer—who is trained as a mathematician but sits in UCSD’s Department of Mechanical and Aerospace Engineering—aims to prepare graduate students for the increasing number of available industry positions. Because many STEM syllabi are already quite full, he recommended that departments create space in existing courses by retaining core concepts but replacing some classical material with modern ML methodology. Kramer listed a myriad of multiscale application areas that benefit from ML and other progressive techniques, including digital twins, climate forecasting, nuclear fusion, hypersonic flight, autonomous driving, drug discovery, precision medicine, quantum computing, and semiconductor design and manufacturing. “If you think about anything that general society or philanthropists are interested in, there’s so many problems to solve,” he said. “The future is very bright.”
Kramer also noted that researchers in data-driven control spaces often seek fast algorithms that are tailored to the specific architecture at hand. “Experts in a field are very happy when someone comes along and says that they can do what they’re doing but faster, more accurately, with lower variance, and whatnot,” he said, citing relevant CSE competencies in high-performance computing, optimization, uncertainty quantification, surrogate modeling, and inverse problems. “Our community has skills that are and will be in high demand for industrial and societal breakthroughs.”
To embrace domain expertise and build experts’ trust in AI architectures, AI researchers should regularly communicate with the scientists and engineers who ultimately “sign the blueprint”—a phrase attributed to the late Ivo Babuška [1, 3]—to greenlight a process and endorse its use in the real world. Typically, these individuals want to understand the underlying principles and potential impacts of the tool in question before doing so. “We need some of these architectures to be used because they’re powerful, but we need to get the engineers to trust them,” Kramer said. “It really unlocks a lot of potential when you work together.”
Gnacadja seconded the importance of engaging with the individuals who utilize the models, generate the data, and stand to benefit from the results. “Get close to the end users and practitioners and understand what interpretability means to them,” he said, remarking that doing so as a junior scientist can inspire an early sense of ownership — especially since real data is often proprietary. “If there’s a way for students and practitioners to see the reality of real data and work on it, it makes that work more impactful.”
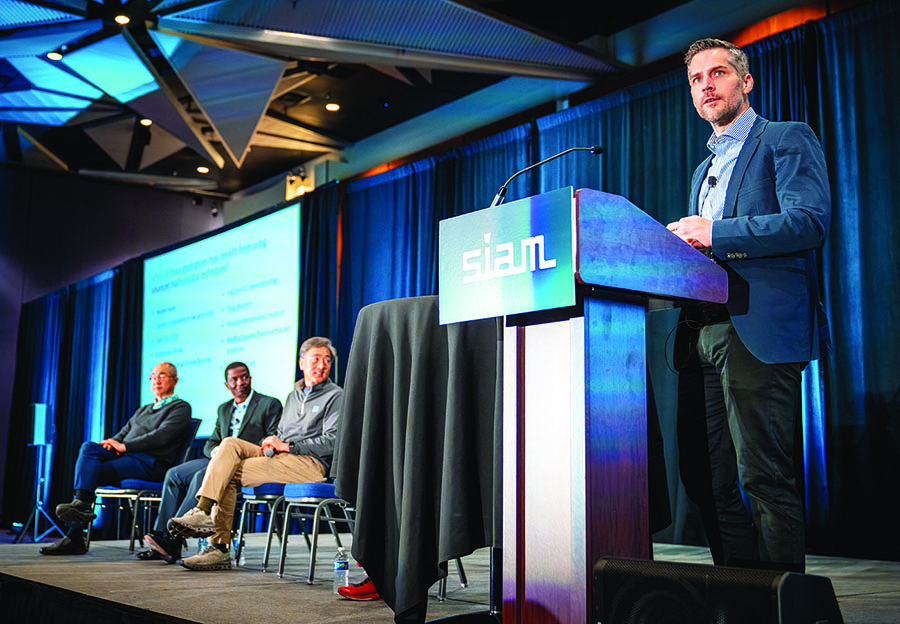
The pharmaceutical industry relies heavily on data, and Gnacadja spoke about Amgen’s efforts to harness state-of-the-art AI technologies to better understand diseases and advance targeted treatments. As the executive director of Amgen’s Data Science and Engineering team, he leads a group of engineers and other specialists who execute innovative AI programs to streamline biopharmaceutical processes. “AI and applied math have contributed a lot to reduce the time for pharma and drug models,” Gnacadja said. These efforts are especially crucial in the drug delivery phase (e.g., clinical trials), which generally requires the largest investment of time and money.
As Amgen works to improve the quality and speed of processes that are already intact, Gnacadja envisioned the use of generative AI to analyze and enhance documentation. “We produce mountains of documents to submit to agencies at various stages of the [drug development] process,” he said. “But I can see a future where instead of sending documents, we send data.” While standards are not yet in place to shape the structure and format of data as an alternative deliverable, Gnacadja is confident that the eventual creation of updated protocols could reduce the time and monetary demands of clinical trials.
Another area that will both inspire and benefit from new approaches is quantum computing, and Kim identified quantum superposition and quantum entanglement as two examples of phenomena with enormous potential. In general, quantum computing presents a unique opportunity to train machines and conduct complex calculations with significantly less data. “We need a different framework of thinking,” Kim said. “In that sense, quantum computing is fairly interesting because it gives you a fundamentally different way of computing than anything we’ve had in history.”
Kim referenced Peter Shor’s 1994 factoring algorithm as a significant breakthrough in the quantum realm [2]. “A quantum computer can factor numbers exponentially faster than classical computers,” he said, adding that quantum computing can also turn exponential scaling into polynomial scaling and offer order-of-magnitude improvements. Yet because researchers have only been controlling and testing quantum systems for roughly the last four decades, many open questions remain. Kim identified two important lines of inquiry: Can quantum models be more compact than classical models, i.e., can researchers build models with fewer parameters but similar structures? And are qubits and gates the most effective solution? “There are many applications where we don’t have enough data for AI to be powerful, but quantum can likely help with this,” Kim said. “And if quantum systems present additional degrees of freedom other than qubits and gates, should we take advantage of them? There’s a lot of really interesting opportunities here, and I feel like the field is just beginning.”
As the session drew to a close, Kim urged attendees to remain open-minded, take risks, stay up to date with new AI tools and other emerging technologies in the CSE space, and train the next generation to venture confidently into a rapidly changing world. “At the end of the day, it’s the structure of real-world data that we have to learn,” he said. “That’s where the foundation and the real knowledge is.”
References
[1] Arnold, D.N. (2023, July 10). Remembering Ivo Babuška. SIAM News, 56(6), p. 2.
[2] Shor, P.W. (1994). Algorithms for quantum computation: Discrete logarithms and factoring. In Proceedings of the 35th annual symposium on foundations of computer science (pp. 124-134). Santa Fe, NM: IEEE Computer Society.
[3] Segeth, K. (2015). Professor Ivo Babuška. Appl. Math., 60(5), 469-472.
About the Author
Lina Sorg
Managing editor, SIAM News
Lina Sorg is the managing editor of SIAM News.
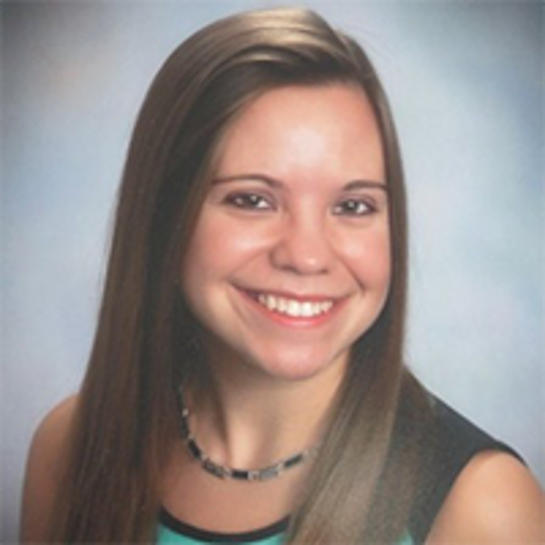
Stay Up-to-Date with Email Alerts
Sign up for our monthly newsletter and emails about other topics of your choosing.