Foundation Model of the Earth Improves Atmospheric Forecasting
Deep learning foundation models are revolutionizing many scientific fields with an improved sense of flexibility, performance, and robustness. These neural networks learn general-purpose representations from a large corpus of data, then leverage the learned representations to complete new downstream tasks when data is scarce. The associated breakthroughs in artificial intelligence (AI) and machine learning that facilitate these efforts happened much more quickly than scientists had originally anticipated. For example, a 2021 article in Philosophical Transactions of the Royal Society A stated that “It is not inconceivable that numerical weather models may one day become obsolete, but a number of fundamental breakthroughs are needed before this goal comes into reach” [2]. Yet less than three years later, a Financial Times headline reported that “AI outperforms conventional weather forecasting for the first time” [1].
Most non-foundation AI and numerical weather prediction (NWP) models are trained on a single task and have a limited scope, even though the actual Earth system involves many diverse tasks, interconnected components, and multiscale interactions. In fact, there are exabytes of untapped data from satellites; weather stations; and numerical weather prediction (NWP) forecasts, analyses, and reanalyses. “Earth science needs foundation models because there’s tons of data,” Paris Perdikaris of the University of Pennsylvania said. “We need a unified approach that can cross domain boundaries, handle multiple scales, learn general representations, and be adapted efficiently.”
During the 2025 SIAM Conference on Computational Science and Engineering, which is currently taking place in Fort Worth, Texas, Perdikaris introduced Aurora: a flexible, large-scale foundation model of the atmosphere that is trained on more than a million hours of diverse weather and climate data. As an encoder-decoder prediction model, Aurora finds strong applications in atmospheric chemistry and air quality, wave modeling, hurricane tracking, and weather forecasting.
The pre-training process exposes Aurora to a high variety of forecast, analysis, and reanalysis data from sources like NASA and the National Oceanic and Atmospheric Administration. “The model is data-hungry,” Perdikaris said, explaining that it takes two states as inputs and uses them to predict the next state. “We know from other foundation models that diversity in training data is crucial for robust generalization. We want the model to learn something about the underlying structure of atmospheric dynamics.”
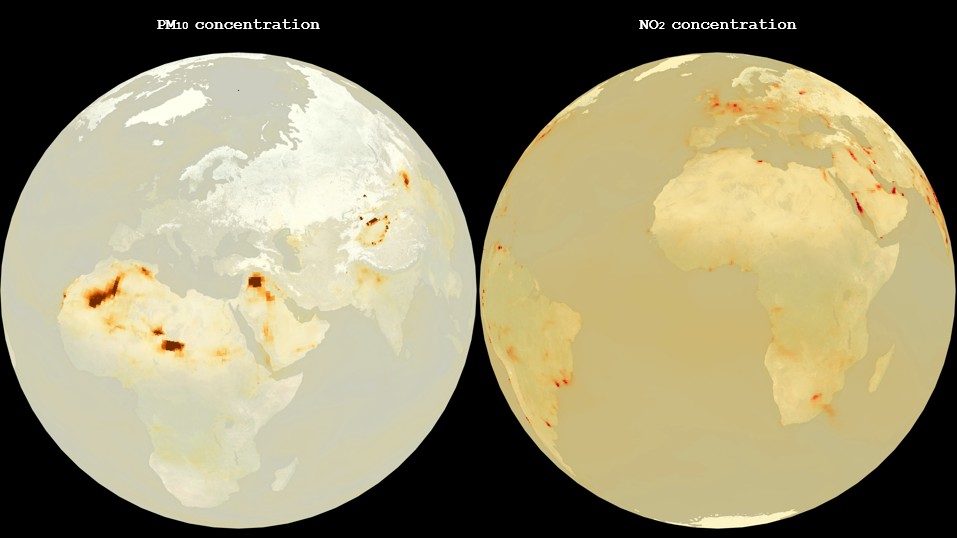
Once Aurora is pre-trained, data-efficient fine-tuning methods use the learned representations to efficiently acclimate the model to novel situations with limited data; doing so increases its ability to accurately solve specific tasks. “We want to cut down on modeling cycles,” Perdikaris said. “We can cut down from years to weeks if we fine tune the model on a new domain.” To illustrate the importance of this step, he presented three unique fine-tuning tasks that challenged Aurora’s ability to adapt to new domains. The first task pertained to air pollution, and Aurora sought to forecast complex chemical processes in the atmosphere—including three different levels of particulate matter and pollutant concentrations for carbon monoxide, nitric oxide, nitrogen dioxide, sulfur dioxide, and ozone—at 0.4° resolution using data from the Copernicus Atmospheric Monitoring Service (CAMS). “We want to predict a new set of variables and generate a five-day forecast globally,” Perdikaris said. Despite the challenging heterogenous nature of air pollution variables, Aurora obtained impressive results with a much lower inference cost. When compared with the CAMS five-day forecast, Aurora proved competitive on 95 percent of all targets and outperformed CAMS on 75 percent. Furthermore, its three-day forecast was competitive on 100 percent of all targets and outperformed CAMS on 86 percent.
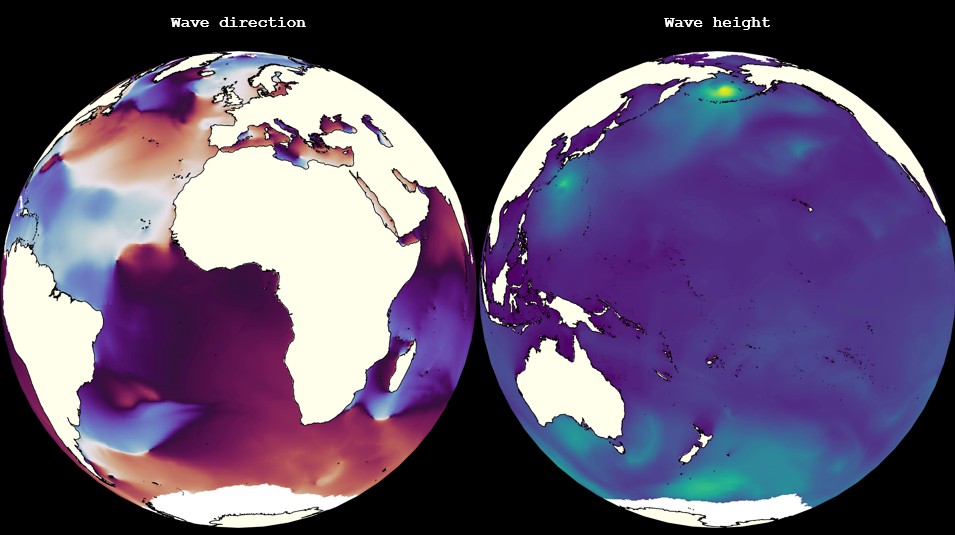
Perdikaris’ second fine-tuning test involved the prediction of ocean wave height, period, and direction based on data from the High RESolution WAve Model (HRES-WAM) of the European Centre for Medium-Range Weather Forecasts’ Integrated Forecasting System (IFS). At 0.25° resolution, Aurora outperformed the HRES-WAM’s operational 10-day forecast for 86 percent of the targets and the three-day forecast for 91 percent of the targets. To further prove Aurora’s prowess, Perdikaris applied it to visualizations of Typhoon Nanmadol, the most intense tropical cyclone of 2022. “Aurora accurately predicts significant wave height and mean wave direction for Typhoon Nanmadol,” he said.
The last and most ambitious fine-tuning task concerned high-resolution weather forecasts at 0.1° resolution. “This is a heavy task on the engineering side,” Perdikaris said. Variables included wind velocity, temperature, specific humidity, and geopotential, and the fine-tuning data was courtesy of the IFS’ highest resolution configuration (HRES). After Aurora outperformed HRES’ 10-day forecast for 92 percent of the targets, Perdikaris compared it at a 0.25° resolution to a 10-day forecast from Google DeepMind’s GraphCast, which represents the state of the art in AI weather prediction. Aurora performed better for 94 percent of the targets.
Perdikaris concluded his presentation with a brief discussion about the importance of data diversity. “Data diversity plays a crucial role in improving generalization, model robustness, and extreme value prediction,” he said. “The highest quality data mix achieves the best overall performance.”
Acknowledgments: As a full-time employee of Microsoft Research in 2023 and 2024, Perdikaris conducted this work with Cristian Bodnar of Silurian AI, Wessel Bruinsma and Megan Stanley of Microsoft Research, Ana Lučić of the University of Amsterdam, and Anna Vaughan and Richard Turner of the University of Cambridge.
References
[1] Cookson, C. (2023). AI outperforms conventional weather forecasting methods for first time. Financial Times. Retrieved from https://www.ft.com/content/ca5d655f-d684-4dec-8daa-1c58b0674be1.
[2] Schultz, M.G., Betancourt, C., Gong, B., Kleinert, F., Langguth, M., Leufen, L.H., … Stadtler, S. (2021). Can deep learning beat numerical weather prediction? Philos. Trans. A: Math. Phys. Eng. Sci., 379(2194), 20200097.
About the Author
Lina Sorg
Managing editor, SIAM News
Lina Sorg is the managing editor of SIAM News.
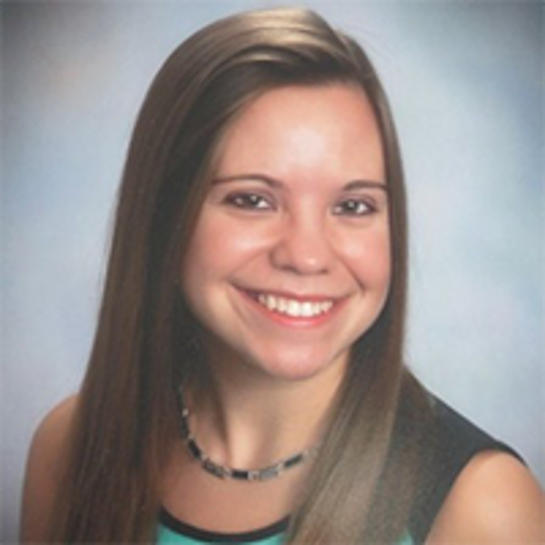
Related Reading
Stay Up-to-Date with Email Alerts
Sign up for our monthly newsletter and emails about other topics of your choosing.