Geospatial Network Models the Distribution of Electric Vehicle Charging Stations
In the field of mathematics, a network is a graphical representation of relationships between different objects. These graphs—which comprise collections of nodes that are connected by edges—find applications in a wide variety of settings, including biology, the social sciences, and transportation. During the 2024 SIAM Conference on Mathematics of Data Science, which is currently taking place in Atlanta, Ga., Gyaneshwar Agrahari of Louisiana State University designed a real-world geospatial network to model the distribution of electric vehicle (EV) charging stations in Texas.
Concrete, real-world networks are inherently messy. They are dynamic, capable of expansion, exhibit heterogeneous probability, and display preferential attachment for new nodes. Given these elements, Agrahari asks three important questions whenever he designs a network:
- What or whom do the nodes represent?
- What kind of relationships do the edges represent?
- How does the problem relate to the design’s assumptions?
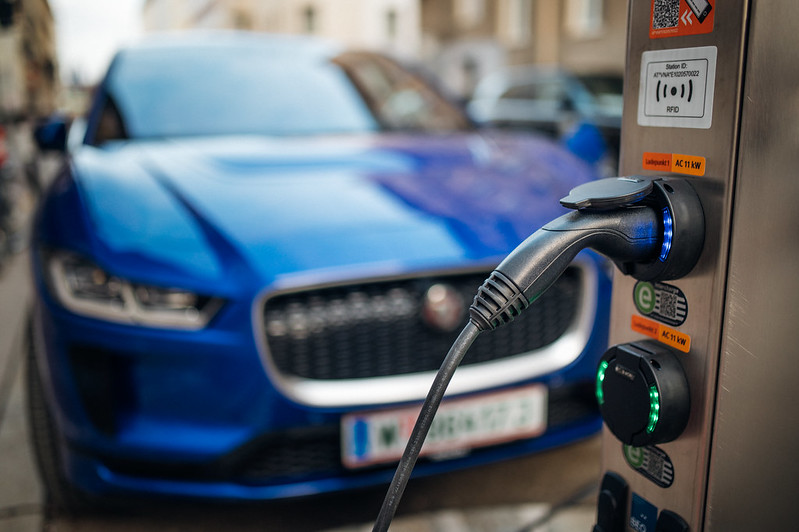
Geospatial networks—wherein the nodes represent physical locations—bring an additional level of complexity to an already complicated situation. Waldo Tobler’s first law of geography states that “everything is related to everything else, but near things are more related than distant things.” While this is often true—i.e., housing prices in adjacent neighborhood are usually similar—Agrahari cautioned that the law does not always apply to geospatial networks. “You have to be very careful when you talk about similarity and the concept of proximity in a geographical setting,” he said.
Networks that map urban areas are especially convoluted because a multitude of factors—including income disparities, social demographics, and housing—contribute to diversity in these spaces. Agrahari focused his presentation on a specific scenario: the locations of EV charging stations in the Dallas-Fort Worth (DFW) metropolitan area. A robust charging infrastructure is necessary to accommodate the growing adoption of EVs and the ongoing transition towards sustainable energy. To strategically expand the burgeoning EV market, governments must understand the spatial distribution of existing frameworks.
Agrahari initially tried using geographical proximity to create edges between charging station nodes, but geographical proximity is not necessarily indicative of real-world proximity — especially in cities. He likewise rejected a road network—where each node is the intersection between a charging station and two roads—because the immense number of nodes and edges quickly became too complex to analyze. Agrahari finally settled on an alternative approach based on travel time, where the edges connect two EV charging stations within a distance of 10 minutes by car. He noticed that the largest cluster of EV stations was centered around downtown Dallas and downtown Fort Worth; these stations ranked higher in closeness and betweenness centrality.
Next, Agrahari sought to enrich the existing structure by identifying relevant node attributes in the EV network, such as traffic density, points of interest (restaurants, malls, hotels, etc.), population density, and EV registration. He also considered the importance of scale. For instance, he looked at average traffic data from the Texas Department of Transportation between 2014-2019 for 4,000 road intersections in the DFW metroplex. When Agrahari examined the data at too small a bandwidth, several hotspots emerged but substantial amounts of traffic were obscured; an appropriately larger bandwidth yielded a more accurate estimation. “Any downstream task using traffic density will rely on the scale of our analysis,” Agrahari said, noting that information can be lost if an incorrect scale is used.
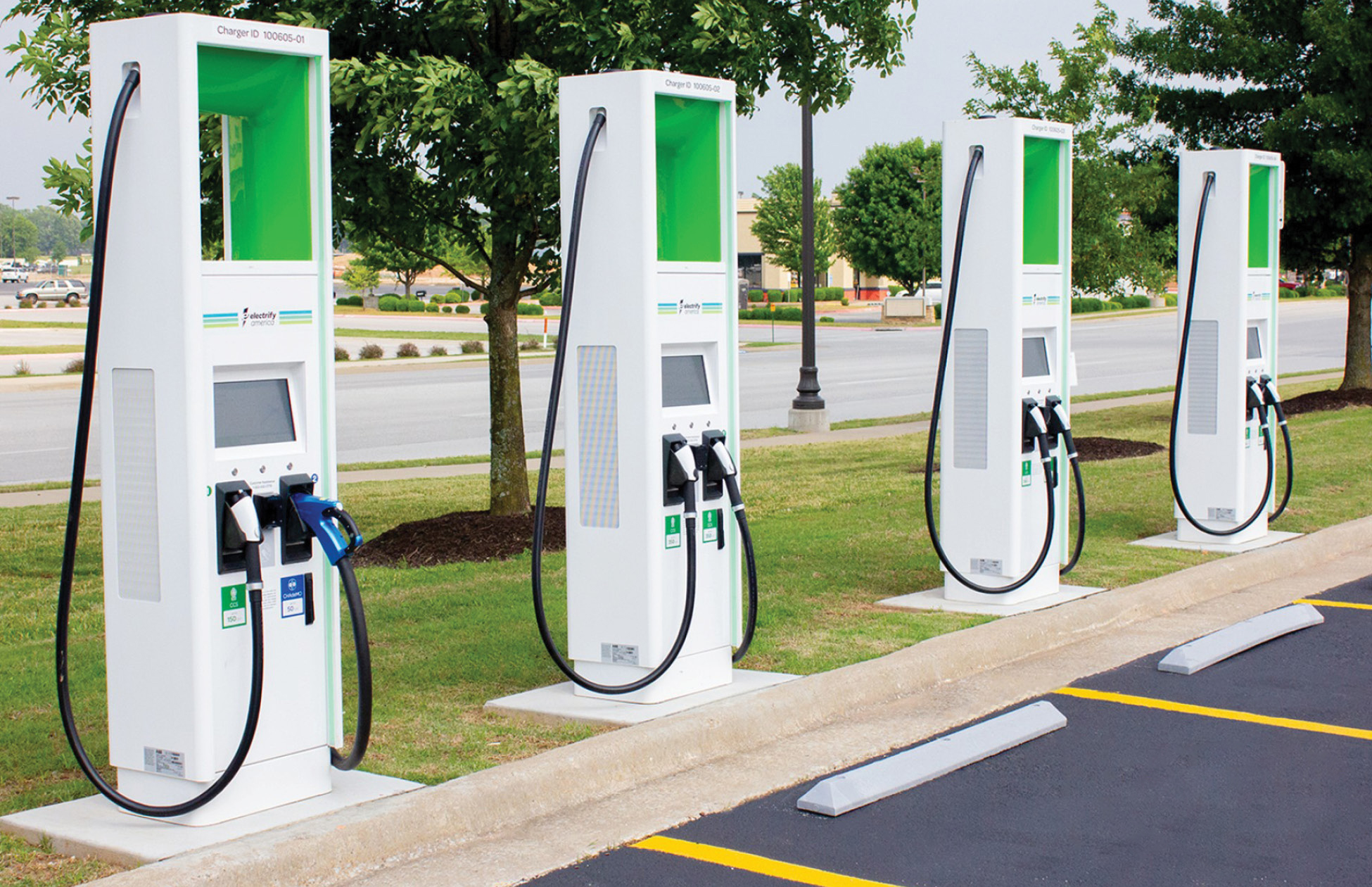
The availability of EV charging stations is particularly relevant to the Justice40 Initiative, part of an executive order that U.S. President Joe Biden issued in January 2021. The Initiative directs 40 percent of the benefits of certain federal investments—including those related to clean energy and energy efficiency, clean transit, affordable and sustainable housing, workforce development, the remediation and reduction of legacy pollution, and clean water infrastructure—to disadvantaged communities (identified via census tract) that are overburdened by pollution. Among other goals, Justice40 aims to increase the number of EV charging stations in these neighborhoods.
The Climate and Economic Justice Screening Tool is a geospatial mapping tool that identifies disadvantaged areas based on a variety of factors, such median household income, housing availability, health, environmental pollution, and the impact of climate change. Despite its good intentions, this method does not consider population in its evaluations and can thus yield some questionable results. For example, it identified the census tract that contains the Dallas Fort Worth International Airport as disadvantaged and in need of EV charging stations; however, the tract’s meager population of only 12 individuals suggests that EV infrastructure is unnecessary in this space.
Agrahari affirmed that limitations of the screening tool can distort information. “If I create a network graph based on government data, you can see how misinformative that graph could be,” he said, adding that such tools should ideally be more precise. “Scale really, really matters. In the government research, you can see that the scale and definition of disadvantaged communities is distorted.” As Agrahari continues this research in the future, he hopes to further examine the government’s policy on EV demand and investigate the tradeoff between the equity and utility of EV charging stations.
About the Author
Lina Sorg
Managing editor, SIAM News
Lina Sorg is the managing editor of SIAM News.
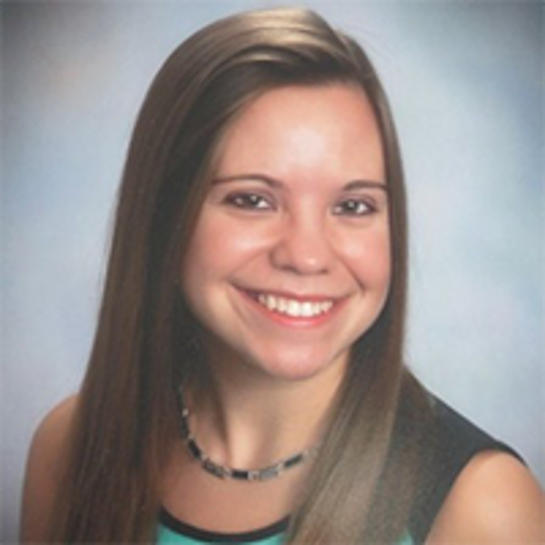