Identifying the Multidisciplinary Competencies of Data Science
Earlier this year, individuals from SIAM, the Association for Computing Machinery (ACM), the American Statistical Association (ASA), and the Mathematical Association of America (MAA) came together to form a task force that seeks to produce a multidisciplinary set of competencies for data science that build upon the ACM’s 2021 Computing Competencies for Undergraduate Data Science Curricula. The representatives from these computing, statistics, and applied mathematics societies aim to define common principles of data science, identify knowledge areas that are relevant to this field, and outline a model undergraduate data science curriculum for colleges and universities that wish to implement their own programs.
During the 2024 SIAM Conference on Mathematics of Data Science, which is currently taking place in Atlanta, Ga., several task force members presented some of the group’s initial work and invited participants to respond to their efforts. Mine Çetinkaya-Rundel of Duke University, Maureen Doyle of Northern Kentucky University, Jamie Haddock of Harvey Mudd College, Rachel Levy of North Carolina State University, and Victor Piercey of Ferris State University all briefly addressed the attendees before organizing breakout groups for further discussion.
The forthcoming ACM-ASA-MAA-SIAM++ Competencies for Undergraduate Data Science Curricula will ultimately serve as a guideline for curriculum development that simplifies the creation and maintenance of degree problems, as well as a guide for accreditation agencies such as the Accreditation Board for Engineering and Technology. The team has been working together for about six months and still have much to do. “It’s a long process,” Levy said. “We’re very much in the thick of it and we’re very excited to get feedback.”
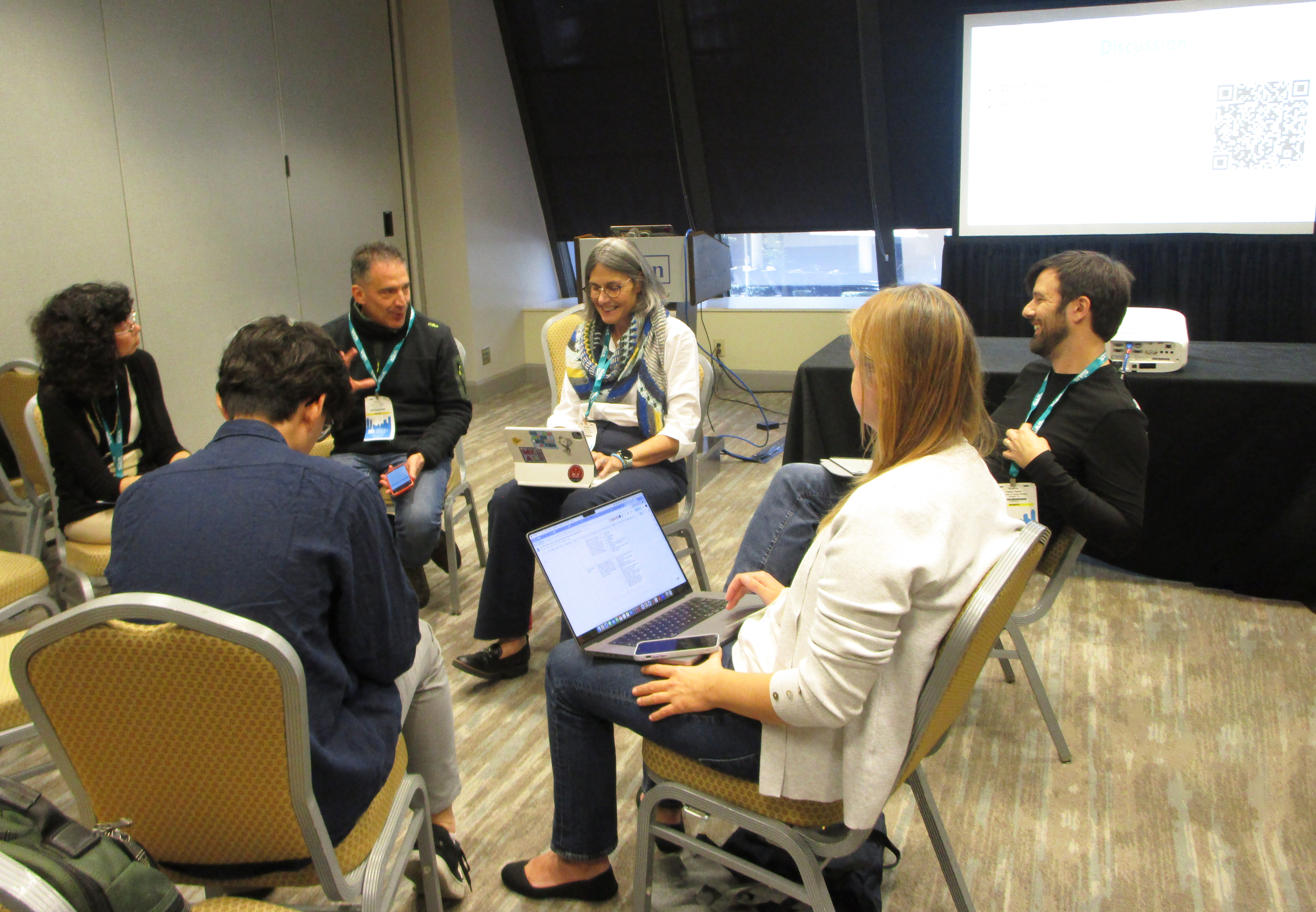
In addition to the aforementioned ACM Computing Competencies document, a multitude of other existing publications provided inspiration for this ongoing endeavor. Such reports include the National Academies of Science, Engineering, and Medicine’s 2018 Data Science for Undergraduates: Opportunities and Options; the 2017 EDISON Data Science Framework; the Park City Math Institute’s 2017 Curriculum Guidelines for Undergraduate Programs in Data Science; and INFORM’s 2015 “Business Analytics Curriculum for Undergraduate Majors.” The representatives are also working with other groups that are undertaking similar projects.
Doyle clarified that the task force is not seeking to design a specific data science course, but rather to identify relevant content that should be included in such courses. “What is the required knowledge that every graduate should know?” she asked. “A data science degree might have a core with a number of different concentrations in other disciplines. That’s why we need to see what’s out there and why we need your feedback.”
The task force is working to classify scopes (what individuals are expected to know), competencies (proof of graduates’ abilities to perform in the job force), and subdomains (more niche areas of study) for a prospective data science degree. Haddock used an example from the 2021 ACM report to better illustrate this concept. For instance, the topic of “computing fundamentals of algorithms” could have a scope of “Comparison of well-known algorithms’ complexity, including machine learning and statistics techniques” and a competency of “provide the big O time and space complexity for a given procedure.”
Doyle explained that competencies exist at the intersection of the following three elements:
- Knowledge, i.e., mastery of content and transfer of learning
- Skills, i.e., abilities and strategies for higher-order thinking and interactions with other people/one’s surroundings
- Dispositions, i.e., personal qualities—such as socioemotional skills, behaviors, and attitudes—that are associated with success in college and one’s career.
At the moment, three different rankings comprise the task force’s hierarchy of competencies. Members are working to assign each knowledge area/competency one of the following labels based on its importance to prospective data science curricula:
- Tier 1 (T1): Required for all data science programs, items that all data science graduates should master
- Tier 2 (T2): Recommended for most programs; any data science graduate is expected to have mastered a majority of T2 items
- Elective (E): Items that belong within data science curricula but could reasonably be regarded as optional; they may contain a deeper knowledge level of T1 or T2 materials.
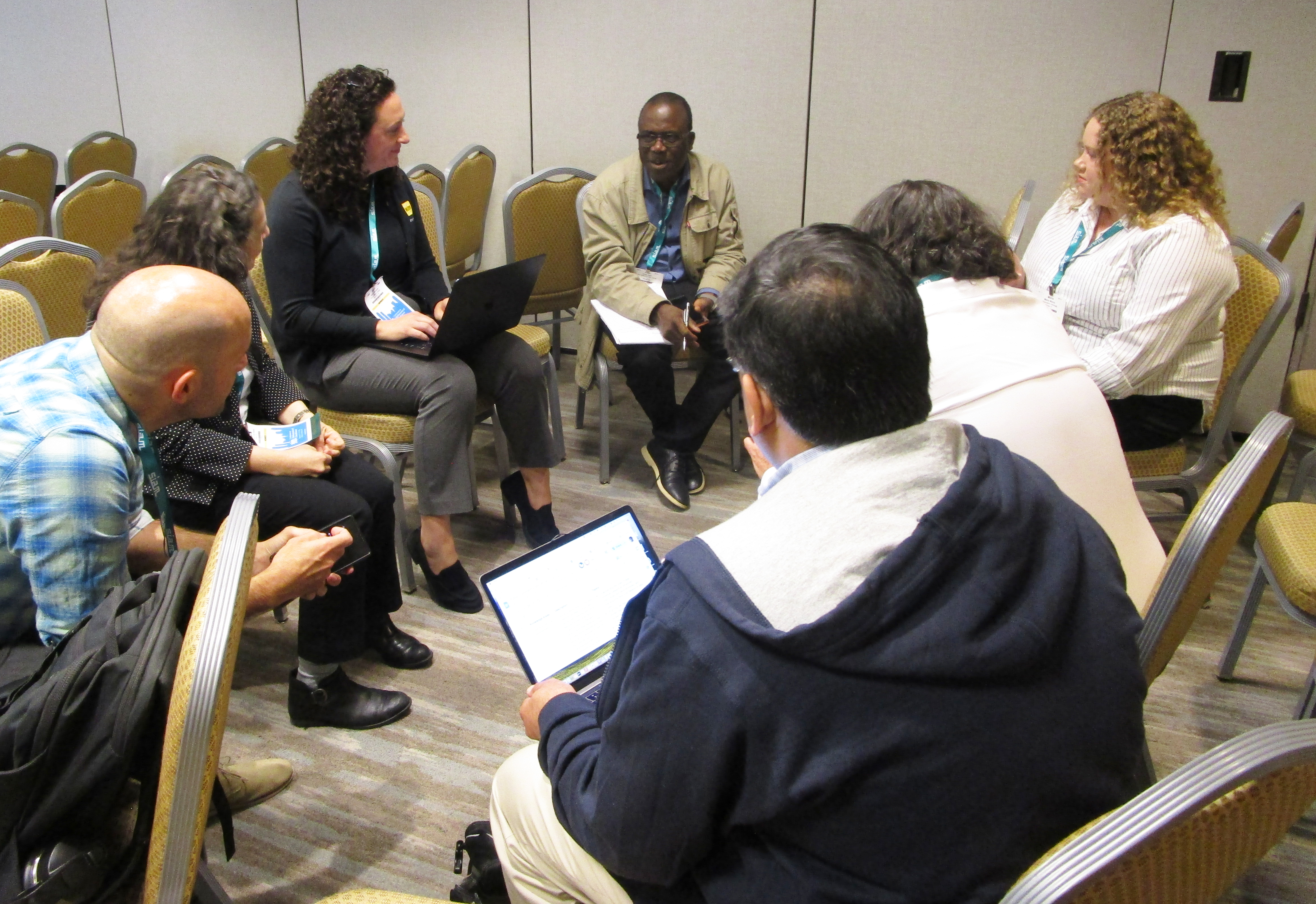
The task force’s working draft of knowledge areas includes computing and computer fundamentals; computing fundamentals and algorithms; data acquisition and representation; exploratory data analysis; data mining and inference; data science project management and collaboration; machine learning and artificial intelligence; model analysis and uncertainty quantification; modeling; scientific computing; presentation, visualization, and communication; professionalism and responsibility; research methods and experimental design; and software development and maintenance. Haddock emphasized the in-progress nature of this list and asked attendees to note any missing areas based on their individual expertise.
The group is presently working to finalize the knowledge areas and subdomains before devoting more effort to competencies. “The goal is to further disseminate these [ideas] in venues like this, or by reaching out to other communities that are teaching data science at the undergraduate level,” Çetinkaya-Rundel said, adding that she and her colleagues are continuously thinking about how each identified area fits into the larger domain of data science. “The goal is to come up with a document that can be all encompassing, but we’re not saying that every single data science degree has to check off every bullet point.”
After the presentation, Piercey divided participants into smaller breakout groups for more intimate conversation. He encouraged them to identify any absent “big pieces” from the existing documentation; review and assess the knowledge areas; label each area as T1, T2, or E based on their own perceptions; and provide any other feedback or comments. At the conclusion of the session, task force members urged everyone to stay in touch and provide survey feedback based on their discussions. Given the rapidity with which the field of data science evolves, they want to reach as many people as possible. “This field is changing so often,” Doyle said. “It’s important for us to get input and feedback so we have something that can be used with other schools as guidelines.”
About the Author
Lina Sorg
Managing editor, SIAM News
Lina Sorg is the managing editor of SIAM News.
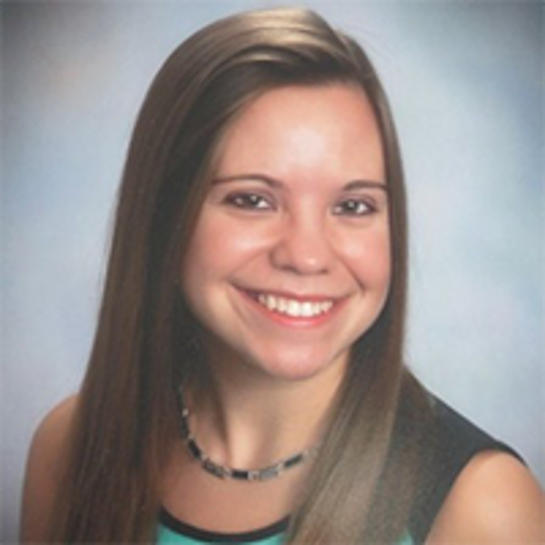
Stay Up-to-Date with Email Alerts
Sign up for our monthly newsletter and emails about other topics of your choosing.