Joint SIAM-BFS Mathematical Finance Online Seminar Series
SIAM is pleased to partner with the Bachelier Finance Society (BFS) on a new joint online seminar series. The Joint SIAM-BFS Mathematical Finance Online Seminar will be held monthly, beginning on March 20, and operated by the SIAM Activity Group on Financial Mathematics and Engineering and BFS. The series aims at keeping the mathematical finance community connected worldwide beyond traditional formats. The goal is to host a lineup of prominent speakers that will present the latest developments in financial mathematics and engineering.
For the March 20 seminar, Terry Lyons, Oxford University, will be joining us to present his work on “The Mathematics of Complex Streamed Data,” followed by Luhao Zhang, Johns Hopkins University, presenting her work on “A Class of Interpretable and Decomposable Multi-period Convex Risk Measures.” Each presentation is planned to be 25 minutes, with five minutes afterward for questions. Learn more about their talks below. Register now for the online seminar series. See you March 20!
Terry Lyons
“The Mathematics of Complex Streamed Data”
Multimodal streamed data is essentially different to unimodal streamed data. Consider this:
‘A commuter arrives at a bus stop before the bus’ – they catch it;
‘The bus arrives first’ – they miss it.
These are the same two events, but the order changes everything. Yet most models treat these as identical: ‘A bus and a person arrived’ They ignore timing and relationships.
This simplification isn’t harmless. A timed series gives no information about order within sampling intervals. As a result, the sampling rate has to come from the bottom up if it is to preserve this order information. Rough path theory makes a radical change and describes the stream over an interval using a group element. According to the choice of group it is possible to capture order information and to allow a top down description of the data stream without using essential information about the order of events.
This approach to describing streamed data is important to data science because it reduces the dimension needed for descriptive feature sets and so reduces the size of the data set needed to train. There are numerous prize winning illustrations of the methodology in use and the impact can be measured in the hundreds of millions of US dollars.
Luhao Zhang
“A Class of Interpretable and Decomposable Multi-period Convex Risk Measures”
Multi-period risk measures evaluate the risk of a stochastic process by assigning it a scalar value. A desirable property of these measures is dynamic decomposition, which allows the risk evaluation to be expressed as a dynamic program. However, many widely used risk measures, such as Conditional Value-at-Risk, do not possess this property. In this work, we introduce a novel class of multi-period convex risk measures that do admit dynamic decomposition.
Our proposed risk measure evaluates the worst-case expectation of a random outcome across all possible stochastic processes, penalized by their deviations from a nominal process in terms of both the likelihood ratio and the outcome. We show that this risk measure can be reformulated as a dynamic program, where, at each time period, it assesses the worst-case expectation of future costs, adjusting by reweighting and relocating the conditional nominal distribution. This recursive structure enables more efficient computation and clearer interpretation of risk over multiple periods.
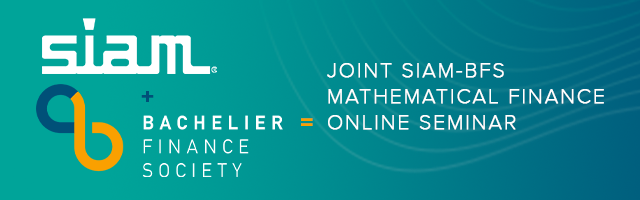
Related Reading
Stay Up-to-Date with Email Alerts
Sign up for our monthly newsletter and emails about other topics of your choosing.