Leveraging Multi-institutional Collaborations to Unlock Impactful Undergraduate Data Science Programs
The growing demand for a data-skilled workforce underscores the ongoing need for comprehensive data science education. While previous efforts have explored optimal teaching methodologies to acquaint undergraduate students with data science, much work remains to ensure curricula alignment with industry expectations and technological advancements [1, 2, 3]. Given data’s increasingly large role in real-world problems, students should familiarize themselves with the relevant societal contexts in analyses and reports.
To address this workforce challenge, the U.S. National Science Foundation (NSF) National Data Science Alliance (NSF NDSA) has begun to coordinate Curriculum Development Workgroups that catalyze the development of data science majors and minors at historically Black colleges and universities (HBCUs). These workgroups leverage multi-institutional collaborations among HBCUs to create a robust network of data science programs, foster innovation, and instantiate high-impact strategies and promising practices. Here, we summarize key findings from the 2023-2024 academic year about data science in undergraduate education, ethically responsible curricula, and culturally relevant experiences that equip students with the necessary skills to thrive in this evolving field.
Creating Multi-institutional Collaborations
From September 2023 through April 2024, three-member teams from 12 institutions participated in workgroups that focused on developing data science programs. Four of these teams—from Bowie State University, Florida A&M University, Harris-Stowe State University, and Morehouse College—developed proposals for academic majors, while the other eight—from Alabama A&M University, Alabama State University, Chicago State University, Clark Atlanta University, Prairie View A&M University, Tennessee State University, Texas A&M University, and Tuskegee University—prepared proposals for academic minors. All participants attended two live, hour-long virtual sessions each month, and each team also held two additional monthly meetings. At the project’s culmination, every team submitted a proposal for a new academic data science major or minor to the curriculum committees at their respective institutions.
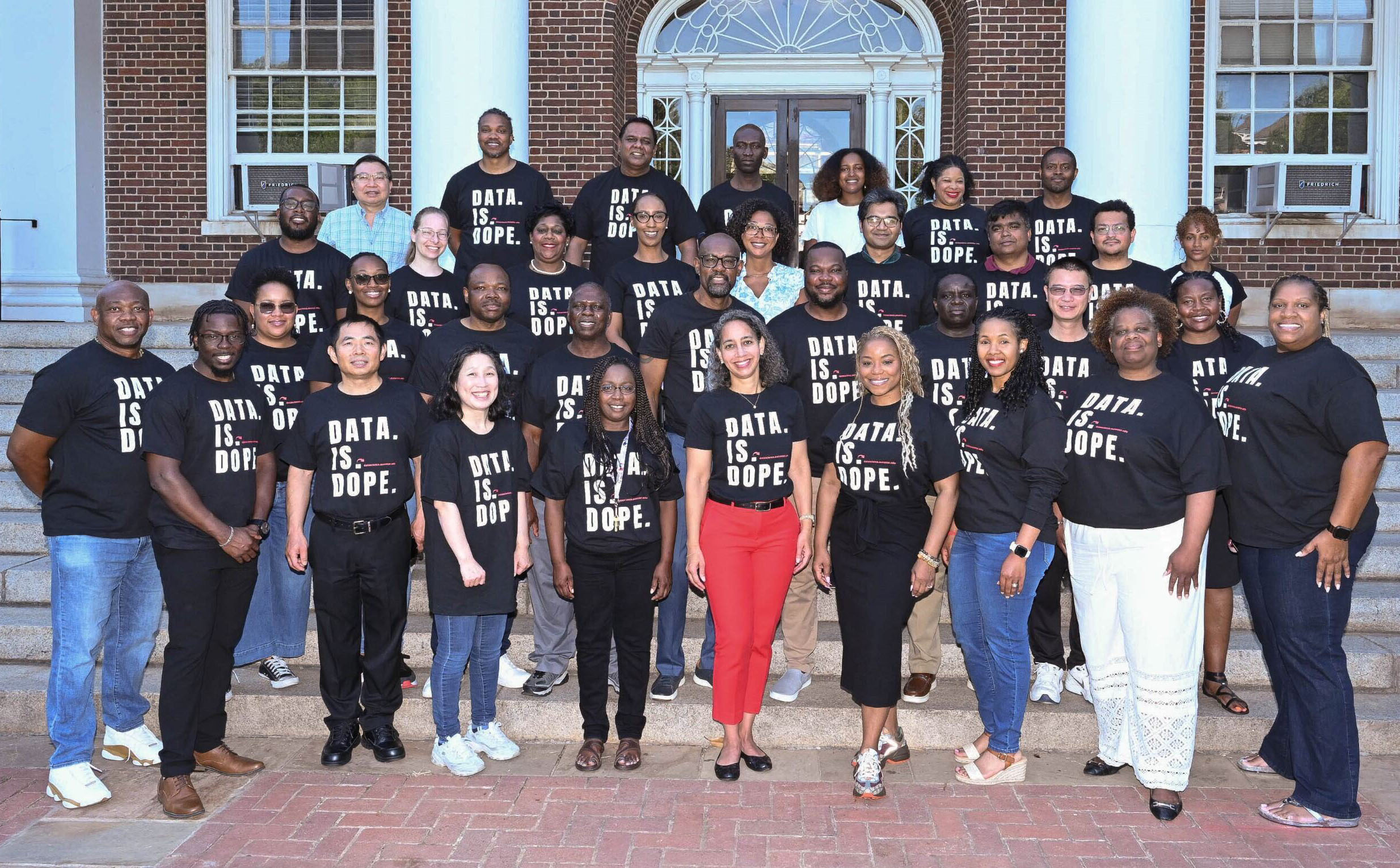
NSF NDSA structured the workgroups based on a cohort model to encourage relationship-building among faculty. Members worked from a shared Google Drive folder to facilitate transparency, communication, and feedback. During the bimonthly group meetings, attendees voiced ideas, insights, and proposals while engaging in ongoing peer reviews of their team’s work products. Participants focused on three guiding questions:
- What is data science?
- How is data science useful?
- Why should students major or minor in data science?
Throughout the academic year, the institutional teams worked through several tasks that fit into the following defined phases:
- Phase 1 – Research and Planning: Teams researched the program approval process, analyzed similar programs at other institutions, and defined their prospective programs’ core focus, name, and intended learning outcomes.
- Phase 2 – Curriculum Development: Building upon their research, teams identified existing courses at their schools that were suitable for inclusion within a data science program, created new courses to address any gaps in existing curricula, and established criteria for the selection of relevant elective courses.
- Phase 3 – Proposal Submission: Teams synthesized their work into comprehensive program proposals, navigated the approval process, presented their proposals to various committees, and incorporated feedback. Proposal submission marked the official end of the workgroups’ cooperative endeavors.
- Follow-up – Implementation and Management: We encouraged workgroups to connect informally with NSF NDSA or other cohort members to share questions and updates about the implementation of their academic programs.
During these phases, workgroups collaborated to identify key elements of data science majors and minors, essential learning outcomes, structural components, recruitment strategies, and alignment to industry needs. Using a participatory action research approach [4], we generated findings by gathering relevant material from bimonthly meetings, surveys, group discussions, reflections, and institutional records (like demographic data, mission statements, and program information). We then conducted a thematic investigation of workgroup discussions that we triangulated with analyses of meeting notes, reflections, and team documentation. This approach enabled a deeper understanding of perspectives and experiences, corroborated by a comprehensive review of program proposals and institutional characteristics.
Defining Data Science
The workgroups used a working definition of data science as “the study of data to extract meaning” to provide context for the subject within their institutions and departments. Several critical components of data science emerged as central for all proposed programs:
- Data storytelling and communication
- Intersections between multiple fields, both within and outside of science, technology, engineering, and mathematics (STEM)
- The systematic collection, cleaning, analysis, and interpretation of data
- The combination of computer science and statistics to analyze big data
- Relevant skills, such as data curation, analysis, communication, and interdisciplinary problem-solving.
Developing Impactful Data Science Programs
Workgroups prioritized creating data science programs for undergraduate students across all fields of study—including STEM, business, social science, and the humanities—to meet workforce demands for data-driven decision-making and innovation skills. They shaped their proposals based on institutional processes, peer programs, and existing courses. The development of data science minors required minimal additions to complement all existing majors without significant course overlap, while the development of data science majors was a more substantial project centered on foundational data science skills. The workgroups’ key focus areas included curriculum design, hands-on experiences, faculty development, student support, and real-world applications. Program placement varied by institution, with most programs falling in departments of mathematics, computer science, business, interdisciplinary studies, and the like. Integration of courses from multiple departments strengthened programs and ensured alignment with evolving data science trends.
Emerging Themes From the Workgroups
The collaborative efforts of workgroup faculty yielded valuable insights into developing and implementing data science programs in higher education, fostering a deeper understanding of the unique needs and opportunities at HBCUs. By sharing effective practices, collaborating on curriculum development, and utilizing a co-developed schedule, participants ultimately produced robust, relevant data science program proposals that can empower their students and contribute to a more skilled data science ecosystem. We found that it was important to tailor data science programs to the unique needs and strengths of each institution according to its mission, strategic plan, and academic offerings; aligning with industry expectations was also essential. Many participants indicated that adherence to these goals—and the subsequent feedback, discussions, and exposure to current data science trends— accelerated the progression of their educational programs.
Next Steps
In the future, NSF NDSA intends to gather feedback from additional workgroups and assess the early implementation of the proposed data science programs from the 2023-2024 academic year. We will also collect data about the programs’ evolution to ensure we can build upon existing progress and insights. By identifying promising practices and strategies that are associated with these new majors and minors, we aim to generate a useful guide for the future creation of data science programs at other institutions.
Acknowledgments: The authors are thankful to the workgroup participants and the U.S. National Science Foundation (NSF) National Data Science Alliance. This work was supported by NSF grant #2217346; any opinions, findings, conclusions, or recommendations in this material are those of the authors and do not necessarily reflect the views of the NSF.
References
[1] Computing Accreditation Commission. (2021). Criteria for accrediting computing programs: Effective for reviews during the 2021-2022 accreditation cycle. ABET. Retrieved from https://www.abet.org/wp-content/uploads/2021/01/C001-21-22-CAC-Criteria.pdf.
[2] De Veaux, R.D., Agarwal, M., Averett, M., Baumer, B.S., Bray, A., Bressoud, T.C., … Ye, P. (2017). Curriculum guidelines for undergraduate programs in data science. Annu. Rev. Stat. Appl., 4, 15-30.
[3] National Academies of Sciences, Engineering, and Medicine. (2018). Data science for undergraduates: Opportunities and options. Washington, D.C.: The National Academies Press.
[4] United Negro College Fund. (n.d.). The impact of HBCUs on diversity in STEM fields. UNCF. Retrieved from https://uncf.org/the-latest/the-impact-of-hbcus-on-diversity-in-stem-fields.Further Reading
• Coburn, C.E., & Penuel, W.R. (2016). Research–practice partnerships in education: Outcomes, dynamics, and open questions. Educ. Res., 45(1), 48-54.
• Creswell, J.W., & Creswell, J.D. (2023). Research design: Qualitative, quantitative, and mixed methods approaches (6th ed.). Thousand Oaks, CA: SAGE Publications.
• National Center for Science and Engineering Statistics. (2023). Diversity and STEM: Women, minorities, and persons with disabilities (Special Report NSF 23-315). Alexandria, VA: National Science Foundation.
• Susman, G. (1983). Action research: A sociotechnical systems perspective. In G. Morgan (Ed.), Beyond method: Strategies for social research (pp. 95-113). Newbury Park, CA: SAGE Publications.
• Washington, T., & Ballenger, H.J. (2024). Atlanta University Center (AUC) Data Science Initiative – Data science framework. SSRN. Retrieved from https://papers.ssrn.com/sol3/papers.cfm?abstract_id=4821889.
• Wentworth, L., Arce-Trigatti, P., Conaway, C., & Shewchuk, S. (2023). Brokering in education research-practice partnerships: A guide for education professionals and researchers (1st ed.). New York, NY: Routledge.
About the Authors
Talitha M. Washington
Professor, Howard University
Talitha M. Washington is executive director of the Center for Applied Data Science and Analytics; the Sean McCleese Endowed Chair in Computer Science, Race and Social Justice; and a professor of mathematics at Howard University. She is the former principal investigator and director of the U.S. National Science Foundation National Data Science Alliance.
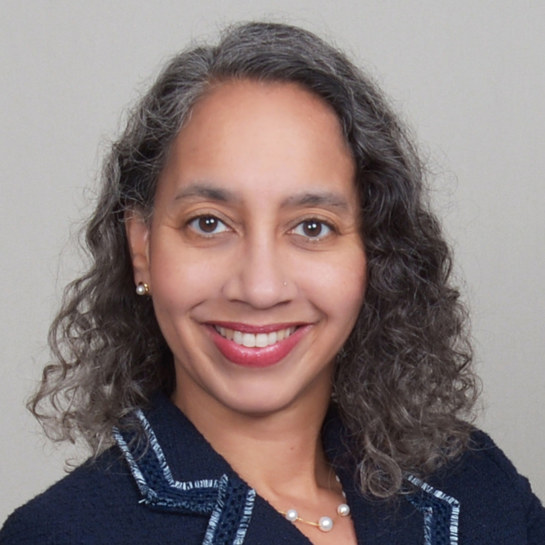
H. Justin Ballenger
Assistant professor, Morehouse College
H. Justin Ballenger is an assistant professor of science, technology, engineering, and mathematics education at Morehouse College. He is the lead researcher for the U.S. National Science Foundation National Data Science Alliance.
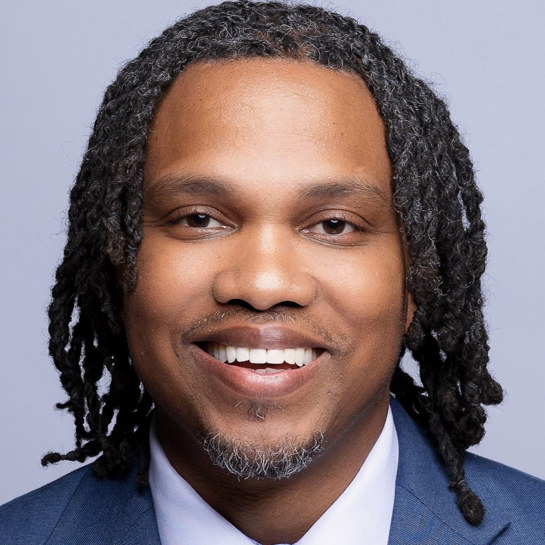
Elycia Daniel
Assistant professor, Clark Atlanta University
Elycia Daniel is an assistant professor of criminal justice at Clark Atlanta University. She serves as the internal evaluator for the U.S. National Science Foundation National Data Science Alliance.
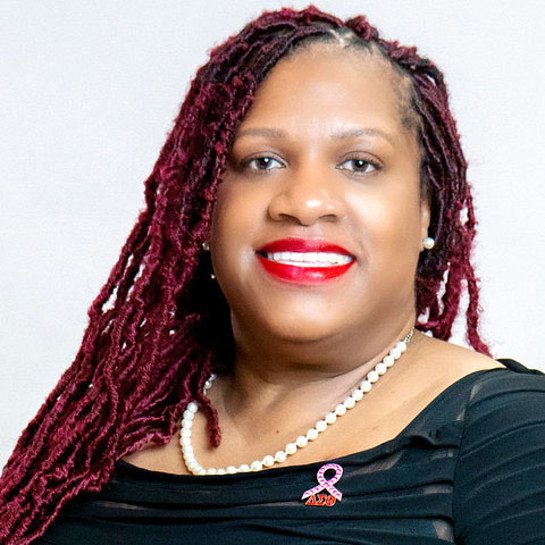
Stay Up-to-Date with Email Alerts
Sign up for our monthly newsletter and emails about other topics of your choosing.