Mathematical Characterization of Preterm Infant Movement Over Time
Movement is a vital part of everyday life that provides insight into our overall health. As with elevated blood pressure or heart rate, difficulty moving may indicate an underlying health problem. This is especially pertinent for preterm infants: babies that are born before 37 weeks of pregnancy (roughly one out of every nine live births in the U.S.). Although neonatal intensive care units (NICUs) track common vitals like heart rate and oxygen saturation, they do not monitor movement as a continuous vital sign. However, the availability of a continuous, quantitative measure of movement in the NICU would enable the study of general movement development and could facilitate earlier diagnoses and treatments for common motor conditions, such as cerebral palsy (CP). Here, we present an approach that applies a validated movement detection algorithm to routine NICU data in order to monitor movement in preterm infants [4].
Automated Movement Detection
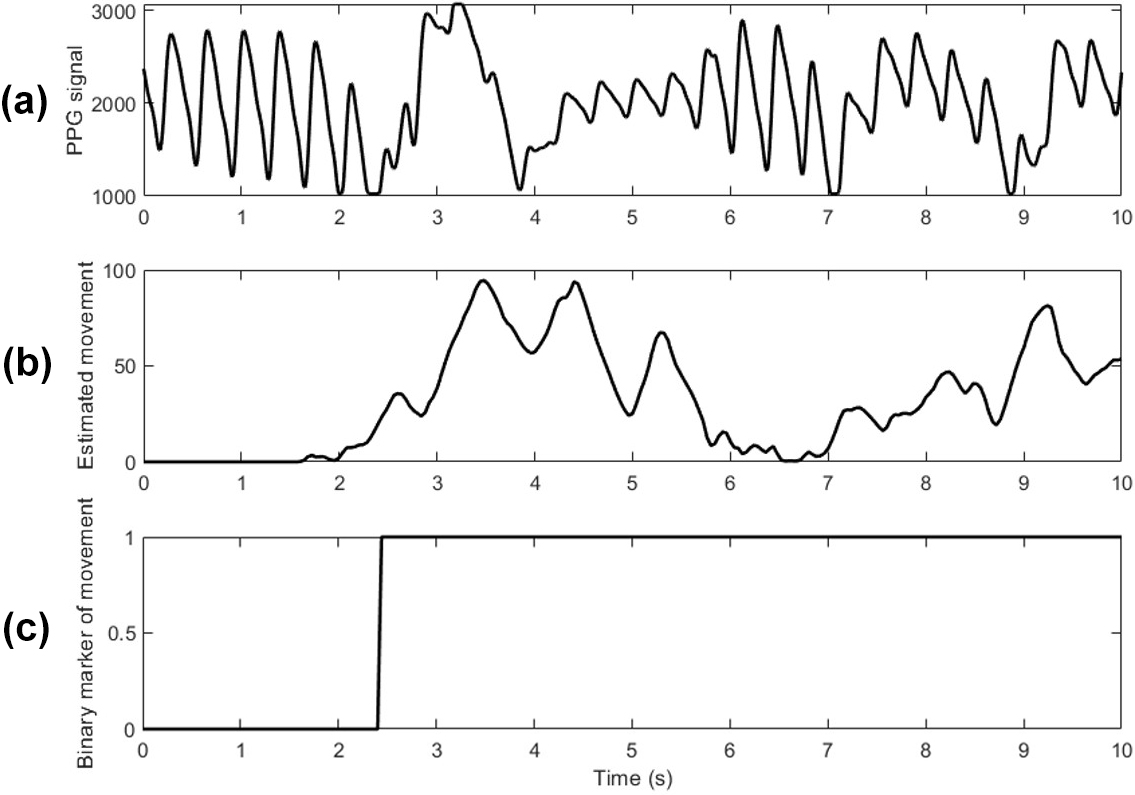
Premature birth can have both short-term and lifelong consequences for an infant. For instance, prematurity correlates with neurological disorders such as CP, which occurs in five to 10 percent of preterm births [2], and approximately 40 percent of preterm infants without CP still experience mild impairment in motor skills [1]. While previous studies have used additional sensors (like accelerometers) to examine preterm infant movement, the challenge of obtaining informed consent has resulted in small cohorts and limited data collection.
To mitigate the constraints of minimal data, we utilized artifacts of routinely collected photoplethysmography (PPG) signals and applied a validated wavelet-based detection algorithm to identify infant movement [4]. As another advantage, this approach does not require the placement of an additional sensor on the infant. Figure 1a depicts the PPG signal, wherein disturbances that signify movement are first evident at roughly two seconds. Figure 1b shows the estimated movement signal after the wavelet transformation; using various thresholds, we obtained a binary signal of \(1\) when the infant is moving and \(0\) when the infant is still (see Figure 1c). Based on this binary signal, we analyzed movement patterns in eight weeks of NICU data. Our study protocol was approved by the Institutional Review Board at The University of Texas at Austin, and we employed the Sickbay Analytics data acquisition system to collect data from two NICUs in Austin, Texas.
Characterizing the Maturation of Movement
We found that bouts of movement over a 24-hour period exhibit a long tail distribution (see Figure 2). The residuals displayed a normal distribution after undergoing a log-log transformation, which indicates that a power law is a good fit. Power laws are common in models of physiological systems, including metabolic rate, heart rate, and growth rate [3]. We defined the power law as
\[P(m) \sim m^{\alpha},\]
where \(P\) is the normalized count of movement bouts, \(m\) is the bin number, and \(\alpha\) is the power law exponent. We then analyzed the change in the exponent to better understand the way in which the distribution of movement shifts as an infant reaches full term, i.e., 40 weeks postmenstrual age (PMA).
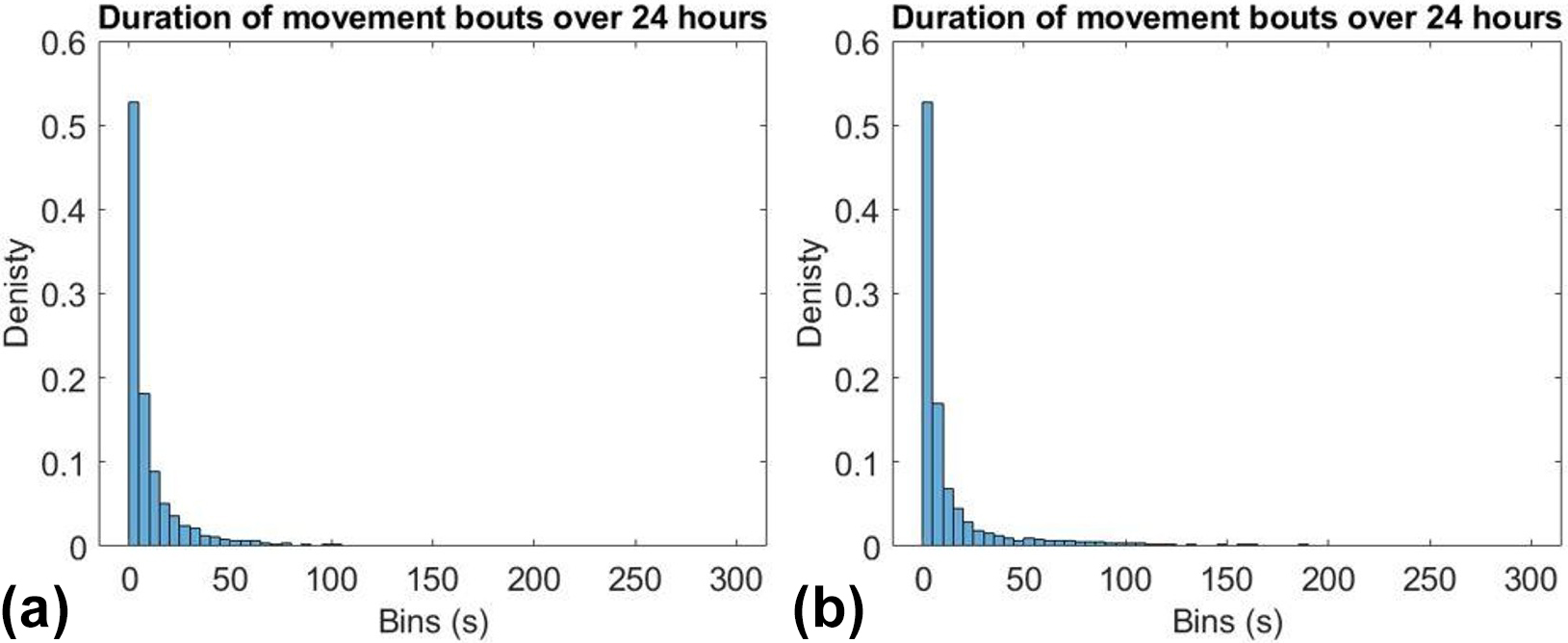
Figure 2 tracks movement bouts for the same infant at 30 and 38 weeks PMA. The infant experienced a higher number of long bouts of movement at 38 weeks than at 30 weeks, which corresponds with a higher power law exponent. Figure 3a illustrates the direct relationship between the power law exponent and PMA. While the distribution of movement bouts does change in relation to PMA, the percent of time that the infant spent moving over a 24-hour period remains consistent (see Figure 3b).
Now that we have established an approach that identifies the movement trajectory of infants, we aim to apply this method to a data set of over 200 babies who spent more than eight weeks in the NICU. Doing so will help us establish the normal trajectory of movement maturation for preterm infants, after which we will be able to compare various clinical outcomes to determine whether the movement trajectories differ significantly. Our findings could potentially lead to earlier diagnoses of movement disorders in the NICU.
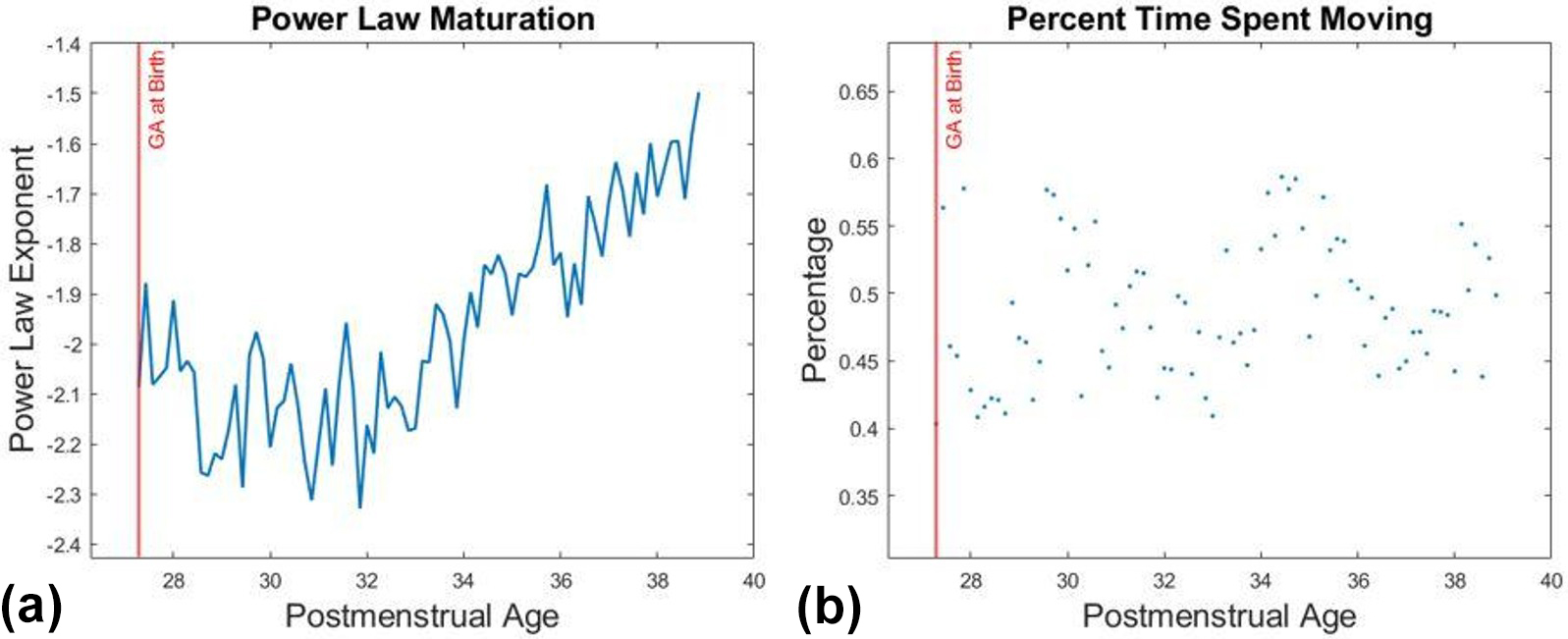
Concluding Remarks
To summarize, we used a validated wavelet detection algorithm to extract a movement signal from the routinely monitored PPG signal. This binary signal displays a power law distribution over a 24-hour period. The number of longer movements increases as the infants reach full term, even though the total time spent in motion remains consistent throughout their stay in the NICU. Moving forward, we plan to apply this framework to our larger data set in order to characterize the normal patterns of movement maturation in the NICU. Deviations from this trajectory may correlate with movement disorders and ultimately allow for earlier detection.
Ally Richardson delivered a minisymposium presentation on this research at the 2023 SIAM Conference on Applications of Dynamical Systems (DS23), which took place in Portland, Ore. She received funding to attend DS23 through a SIAM Student Travel Award. To learn more about Student Travel Awards and submit an application, visit the online page.
SIAM Student Travel Awards are made possible in part by the generous support of our community. To make a gift to the Student Travel Fund, visit the SIAM website.
Acknowledgments: I would like to thank David Paydarfar and Joshua Chang of The University of Texas at Austin for their comments on this article.
References
[1] Barre, N., Westrupp, E.M., Howard, K., & Anderson, P.J. (2011). Long-term outcome following preterm birth. In G. Goldstein, T.M. Incagnoli, & A.E. Puente (Eds.), Contemporary neurobehavioral syndromes. New York, NY: Psychology Press.
[2] Sellier, E., Platt, M.J., Andersen, G.L., Krägeloh-Mann, I., De La Cruz, J., & Cans, C. (2016). Decreasing prevalence in cerebral palsy: A multi-site European population-based study, 1980 to 2003. Dev. Med. Child Neurol., 58(1), 85-92.
[3] West, G.B., & Brown, J.H. (2005). The origin of allometric scaling laws in biology from genomes to ecosystems: Towards a quantitative unifying theory of biological structure and organization. J. Exp. Biol., 208(9), 1575-1592.
[4] Zuzarte, I., Indic, P., Sternad, D., & Paydarfar, D. (2019). Quantifying movement in preterm infants using photoplethysmography. Ann. Biomed. Eng., 47(2), 646-658.
About the Author
Ally Richardson
Ph.D. candidate, The University of Texas at Austin
Ally Richardson is a Ph.D. candidate in the Computational Science, Engineering, and Mathematics program of the Oden Institute for Computational Engineering and Sciences at The University of Texas at Austin. She is interested in computational medicine, particularly the application of modeling and simulation techniques to biomedical problems. Richardson holds a B.S. in applied mathematics and B.A. in physics from Buffalo State University.
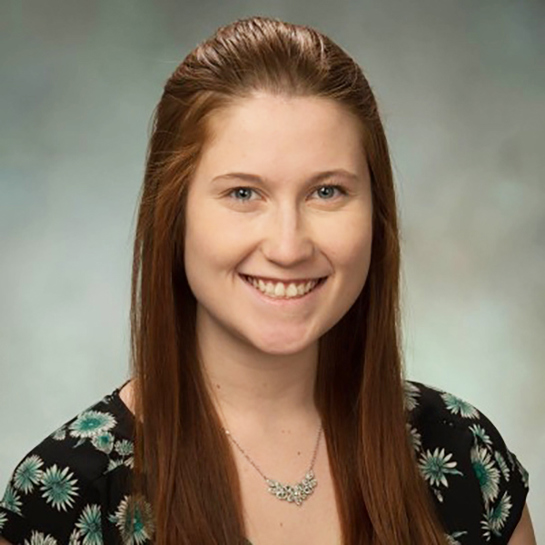
Stay Up-to-Date with Email Alerts
Sign up for our monthly newsletter and emails about other topics of your choosing.