Mathematical Model Explores Clinical Biomarkers and Personalized Treatment for Alzheimer’s Disease
Article updated on November 14, 2023
According to the Centers for Disease Control and Prevention, Alzheimer’s disease (AD) is the most common form of dementia and the fifth leading cause of death in U.S. adults who are 65 years or older. In fact, the Alzheimer’s Association estimates that roughly 6.7 million Americans in this age demographic are currently afflicted with AD, though that number is expected to grow to 12.7 million by 2050.
AD is named after Alois Alzheimer (1864-1915), a German psychiatrist and neuropathologist who was the first person to study and report on a patient with “presenile dementia.” The patient—Aguste Deter—experienced a rapid escalation in memory loss in her late 40s that led to delusions, paranoia, and general confusion; she ultimately lost the ability to read, recall facts about her life, and even remember her own name. Alzheimer observed Deter in a Frankfurt asylum until her death in 1906, after which he autopsied her brain to better understand the mental deterioration. He noted the presence of amyloid plaques and neurofibrillary tangles (NFTs): brain anomalies that are now hallmark identifiers of AD.
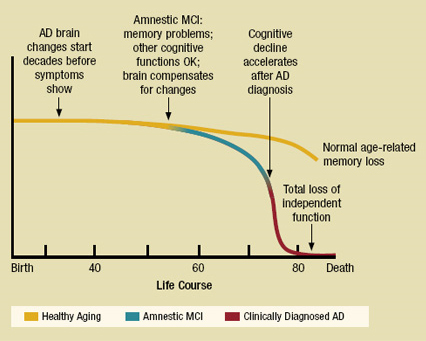
Figure 1 compares the progression of AD with healthy aging and amnestic mild cognitive impairment (MCI), a precursor to AD during which patients undergo memory loss but retain other cognitive functions. Because AD-related changes in the brain begin decades before symptoms arise, no distinction is initially evident between AD and the normal aging process. Between age 50 and 60, people with AD begin to exhibit symptoms of amnestic MCI. Cognitive decline then swiftly accelerates after AD diagnosis, ultimately causing a total loss of independent function and eventual death. Figure 2 displays a brain cross section for patients with normal cognition versus those with AD. As evident in the figure, the disease targets areas that pertain to memory and language before eventually spreading throughout the entire brain to destroy the remaining functions.
During a minisymposium presentation at the 2023 SIAM Conference on Control and Its Applications, which took place in July in Philadelphia, Pa., Wenrui Hao of the Pennsylvania State University shared a mathematical model of AD that describes the progression of clinical biomarkers and incorporates public patient data for personalized prediction and optimal treatment. Hao began by referencing his 2016 model of AD with Avner Friedman that utilized a system of partial differential equations (PDEs) and included neurons, astrocytes, microglias, peripheral macrophages, and amyloid beta (\(\rm{A}\beta\)) aggregation and hyperphosphorylated tau proteins [1]. Subsequent work identified three classical biomarkers for AD diagnosis: \(\rm{A}\beta42\), total tau (t-tau), and phosphorylated tau (p-tau) [2].
\(\rm{A}\beta\) and the amyloid precursor protein (APP) are critical to the study of AD. AAP is a transmembrane protein that serves as a cell surface receptor. It is created by neurons and other brain cells whose breakdown produces \(\rm{A}\beta\) peptides. “\(\rm{A}\beta\) is cut from APP by the enzymes \(\beta\)-secretase and \(\gamma\)-secretase, which release \(\rm{A}\beta\) monomers into the extracellular environment,” Hao said. In individuals with AD, a mutation compels \(\rm{A}\beta42\) to clump together and form a plaque that collects between neurons and affects cell function.
Internal structures called microtubules support neurons by guiding nutrients and molecules to their designated locations. In healthy neurons, tau proteins bind to and stabilize microtubules. But in the presence of AD, abnormal chemical changes cause tau to detach from the microtubules and bind to other tau molecules. This accumulation of phosphorylated tau leads to the formation of NFTs inside of neurons, which is linked to synaptic impairment and neuronal dysfunction.
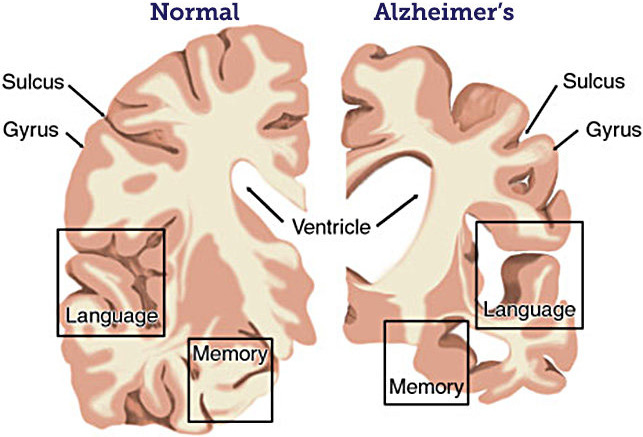
Hao next introduced the popular amyloid cascade hypothesis, which suggests that the abnormal accumulation of \(\rm{A}\beta\) plaque throughout the brain is primarily responsible for the neurodegeneration that is associated with AD. “Most people accept it, but there’s no equation for it,” Hao said. He and his collaborators thus sought to build a model with clinical biomarkers that is based on this hypothesis.
Hao presented a simple system of ordinary differential equations (ODEs) that illustrates the numerical algorithm of personalized parameter estimations. “You play with the parameters so you can get different disease progressions of AD,” he said; such parameters represent early-onset AD, autosomal dominant AD, and late-onset tau-first AD. He then personalized the model for a variety of patients with comprehensive data from the Alzheimer’s Disease Neuroimaging Initiative (ADNI).
Because the system is a cascade model, Hao utilized an equation-by-equation algorithm that eventually yields an initial condition. After solving the optimization problem and assessing the predictive accuracy, he used a polynomial basis to verify his model and approximate the ODE system. “The parameters of the ODE model are fitted based on the ADNI dataset by minimizing the sum of squared differences between the data and the solution of the causal model,” Hao said. He found that the onset of disease and rate of progression vary within and among subject classes of cognitively normal, late amnestic MCI, and AD individuals in the ADNI. The results also fluctuated depending on the number of complete training sets.
Hao concluded his presentation with a brief discussion of AD clinical trials. In June 2021, the U.S. Food and Drug Administration approved an anti-\(\rm{A}\beta\) antibody called aducanumab (sold under the brand name Aduhelm) that targets the fundamental pathophysiology of AD. Hao applied his cascade model to personalize and optimize doses of aducanumab based on clinical trial data. He modeled an anti-amyloid therapy for an AD patient in short-term treatment (78 weeks) and long-term treatment (10 years) to (i) minimize cognitive impairment and any side effects over the treatment interval and (ii) minimize the cognitive impairment and level of amyloid by the treatment’s end. Hao found that beginning treatment by age 60 can reduce cognitive decline by five percent.
Ultimately, Hao’s model of clinical biomarkers helps to influence personalized prediction and optimal treatment studies for AD. In this context, personalized models also perform more accurately than traditional methods. “Each patient has different personalized parameters and optimal dosing functions,” Hao said. In the future, he hopes to incorporate imaging data into this model by extending the ODE system to a system of PDEs.
References
[1] Hao, W., & Friedman, A. (2016). Mathematical model on Alzheimer’s disease. BMC Syst. Biol., 10(1), 108.
[2] Janeiro, M.H., Ardanaz, C.G., Sola-Sevilla, N., Dong, J., Cortés-Erice, M., Solas, M., … Ramírez, M.J. (2021). Biomarkers in Alzheimer’s disease. Adv. Lab. Med., 2(1), 27-37.Further Reading
- Hao, W., Lenhart, S., & Petrella, J.R. (2022). Optimal anti-amyloid-beta therapy for Alzheimer’s disease via a personalized mathematical model. PLoS Comput. Biol., 18(9), e1010481.
- Petrella, J.R., Hao, W., Rao, A., & Doraiswamy, P.M. (2019). Computational causal modeling of the dynamic biomarker cascade in Alzheimer's disease. Comput. Math. Methods Med., 3, 6216530.
- Zheng, H., Petrella, J.R., Doraiswamy, P.M., Lin, G., & Hao, W. (2022). Data-driven causal model discovery and personalized prediction in Alzheimer's disease. npj Digit. Med., 5, 137.
About the Author
Lina Sorg
Managing editor, SIAM News
Lina Sorg is the managing editor of SIAM News.
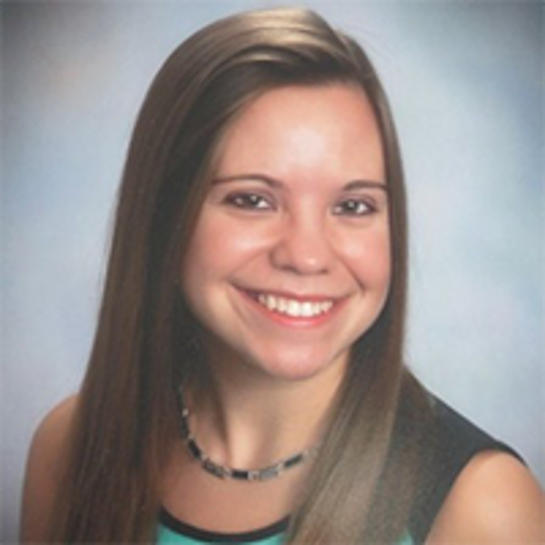
Stay Up-to-Date with Email Alerts
Sign up for our monthly newsletter and emails about other topics of your choosing.