Mathematics and Merck: An Impactful Collaboration
While mathematics has contributed to the pharmaceutical industry for decades, the need for quantitative approaches has grown explosively in recent years. A mathematical career in pharma offers exciting opportunities to solve some of the most complicated and pressing issues in human health. As active scientists in the Quantitative Pharmacology & Pharmacometrics department at Merck, we employ a variety of mathematical techniques, including model creation and development; ordinary differential equations (ODEs), especially in nonlinear mixed-effect analyses; partial differential equations (PDEs); experimental design; statistics; data wrangling and visualization; artificial intelligence (AI); numerical methods; and image analysis.
Mathematical models and simulation results use the available (and often limited) internally and externally generated data to predict drug safety and efficacy, and we use these predictions to influence decisions regarding program continuation/cancellation, clinical (and other) trial/experiment design, and dosage amount and frequency. Our efforts and their impact require interdisciplinary collaboration, and we share our learnings through scientific, peer-reviewed publications and presentations.
Examples of our impactful work at the intersection of mathematics and pharma include the following:
- Early trials for pembrolizumab—an immunotherapy drug that is approved for treating several forms of cancer—assessed the relationship between dose and efficacy. We used a model-based approach to design trials and analyze data to better understand the nonlinearity that occurs at low doses [4].
- The diversity of human physiology can complicate data interpretation. We created a visualization tool that plots multi-covariate models with the complex underlying data in a manner that is understandable for both modelers and non-modelers (clinicians, biologists, and others) [8]. This tool has made it possible to influence key decisions on programs that aim to prevent respiratory syncytial virus (RSV) and COVID-19.
- Given the rapid rise of RSV and COVID-19 infections, we used model-based meta-analyses to help determine doses used in clinical trials. We also used generalized linear and nonlinear models to inform decisions that helped redirect efforts toward drug/vaccine development endeavors that are more likely to improve public health [7, 9].
- Our PDE simulations of electric fields led to a novel in vitro experiment for probing voltage-gated ion channels, thus enabling a quick and efficient assessment of the activity of small molecules against these important targets [2].
- During the development of the nonavalent human papillomavirus (HPV) vaccine, a large set of coupled, nonlinear ODEs predicted the impact of such a vaccine on the HPV epidemic. This model enabled the prediction of the vaccine’s benefits for males in the U.S. and provided evidence to support the official recommendation that men receive the vaccine. It also helped to enable the vaccine’s use in other countries and shaped vaccination strategies, such as the age groups to vaccinate for efficient public health impact [5].
- The pharmaceutical industry is actively developing antibody therapies for the treatment of various central nervous system diseases; however, the limited transport of these antibodies into the brain makes dose predictions difficult. Our minimal, mechanism-based ODE model includes a brain compartment to better understand concentrations of antibodies in the brain and predict human doses from animal study data [1].
- We created a nonlinear, mixed-effect ODE pharmacokinetic model of vericiguat (a drug that people take after hospitalization for heart failure) that was based on data from 2,000 patients. Our model found that disease-related factors—such as left ventricular ejection fraction or New York Heart Association Functional Classification—did not impact the pharmacokinetics. This result supported the recommendation that all patients should receive the same dose [10].
- Our machine learning efforts help scientists understand the biology that is implied by very large data sets. Insight into the interactions of the components (cells, chemicals, etc.) of biological systems is critical to figuring out, for example, if reducing the activity of a specific protein will have the desired effect, or if the system is likely to compensate by “going down another road” in the “network” of interactions. Our DEKER-NET algorithm improves our ability to deduce these networks by directly identifying (without resorting to arbitrary thresholds) an interpretable network with relatively few components and connections (“edges”) [6].
- Our Probability of Disease Bayesian Analysis (PoDBAY) method estimates a vaccine’s efficacy based on the immune response after vaccination. The resulting models have aided in the design and acceleration of trials, assisted in the selection of vaccine dose levels, and enabled a better way to quantify potential differences (in protection) between populations [3].
This list has only a few examples of the areas in which we contribute. Other applications for mathematics within pharma include:
- Computational models of the fluid dynamics, reactions, and transport in engineering bioreactors
- Bioinformatics that increase our understanding of humans’ immune response to protein sequences and facilitate even faster discovery and development of vaccines and monoclonal antibodies
- Methods to extract more high-quality information from medical images (and to do so even faster).
Many different types of math and statistics jobs are available at Merck and other pharmaceutical companies. All positions that use math to influence healthcare-related decisions require a desire to learn quickly and collaborate across disciplines. Most researchers acquire domain-specific knowledge on the job while further enhancing their oral and written communication skills. There are several types of entry-level positions for applied mathematicians at Merck. For example, the associate scientist (B.S./B.A.) and scientist (M.S.) roles involve wrangling and analyzing data and developing data analysis/visualization tools. Senior scientists (Ph.D.) complete those tasks and serve as technical experts who lead projects by collaboratively building and simulating models and interpreting and communicating the results. To search for pharma jobs at Merck and other companies, look for terms like “pharmacometrics,” “quantitative systems pharmacology” (also known as “QSP”), “image analysis,” “computational biology,” “statistician,” “modeling/modeler,” “population pharmacokinetic (popPK) models,” and “disease progression models.”
SIAM members have access to even more job postings, recent journal articles, and additional learning opportunities. In fact, two of the authors of this write-up have found jobs through SIAM events!
References
[1] Bloomingdale, P., Bakshi, S., Maass, C., van Maanen, E., Pichardo-Almarza, C., Yadav, D.B., … Mehrotra, N. (2021). Minimal brain PBPK model to support the preclinical and clinical development of antibody therapeutics for CNS diseases. J. Pharmacokinet. Pharmacodyn., 48(6), 861-871.
[2] Bugianesi, R.M., Augustine, P.R., Azer, K., Dufresne, C., Herrinton, J., Kath, G.S., … Slaughter, R.S. (2006). A cell-sparing electric field stimulation technique for high-throughput screening of voltage-gated ion channels. ASSAY Drug Dev. Technol., 4(1), 21-35.
[3] Dudášová, J., Laube, R., Valiathan, C., Wiener, M.C., Gheyas, G., Fišer, P., … Sachs, J.R. (2021). A method to estimate probability of disease and vaccine efficacy from clinical trial immunogenicity data. NPJ Vaccines, 6, 133.
[4] Elassaiss-Schaap, J., Rossenu, S., Lindauer, A., Kang, S.P., de Greef, R., Sachs, J.R., & de Alwis, D.P. (2017). Using model-based “learn and confirm” to reveal the pharmacokinetics-pharmacodynamics relationship of pembrolizumab in the KEYNOTE-001 trial. CPT Pharmacometrics Syst. Pharmacol., 6(1), 21-28.
[5] Elbasha, E.H. (2006). Impact of prophylactic vaccination against human papillomavirus infection. In A.B. Gumel, C. Castillo-Chavez, R.E. Mickens, & D.P. Clemence (Eds.), Mathematical studies on human disease dynamics: Emerging paradigms and challenges (pp. 113-127). Contemporary mathematics (Vol. 410). Providence, RI: American Mathematical Society.
[6] Hayes, S.M.S., Sachs, J.R., & Cho, C.R. (2022). From complex data to biological insight: ‘DEKER’ feature selection and network inference. J. Pharmacokinet. Pharmacodyn., 49(1), 81-99.
[7] Kandala, B., Plock, N., Chawla, A., Largajolli, A., Robey, S., Watson, K., … Sachs, J.R. (2022). Accelerating model-informed decisions for COVID-19 vaccine candidates using a model-based meta-analysis approach. eBioMedicine, 84, 104264.
[8] Lommerse, J., Plock, N., Cheung, S.Y.A., & Sachs, J.R. (2021). V2ACHER: Visualization of complex trial data in pharmacometrics analyses with covariates. CPT Pharmacometrics Syst. Pharmacol., 10(9), 1092-1106.
[9] Mass, B.M., Lommerse, J., Plock, N., Railkar, R.A., Cheung, S.Y.A., Caro, L., … Sachs, J.R. (2021). Forward and reverse translational approaches to predict efficacy of neutralizing respiratory syncytial virus (RSV) antibody prophylaxis. eBioMedicine, 73, 103651.
[10] Trujillo, M.E., Arrington, L., Patel, Y., Passarell, J., Wenning, L., Blaustein, R.O., … Gheyas, F. (2022). Population pharmacokinetics of vericiguat in patients with heart failure with reduced ejection fraction: An integrated analysis. Clin. Pharmacol. Ther., 112(5), 1061-1069.
About the Authors
Callie J. Miller
Quantitative pharmacometrician, Merck
Callie J. Miller is a self-described mathematically inclined bioengineer with quantitative and qualitative research experiences from pedagogy to morphogenesis, as well as professional experiences from academia to her current job as a quantitative pharmacometrician at Merck.
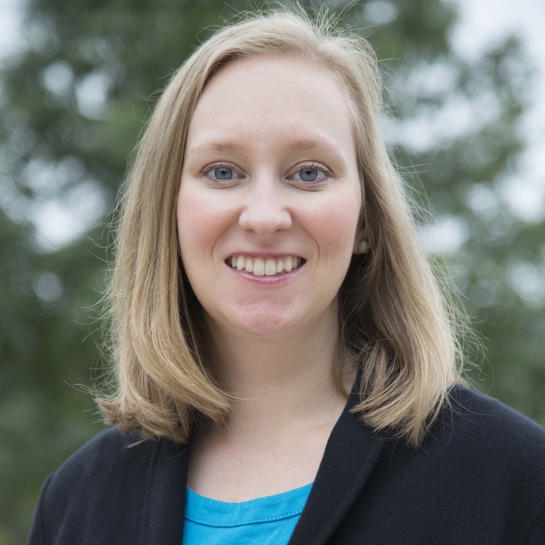
Janita P. Hogan
Applied mathematician, Merck
Janita P. Hogan is an applied mathematician by training who uses her modeling and computational skills to provide insights from preclinical and clinical data to drive decision-making for drug discovery and development at Merck.
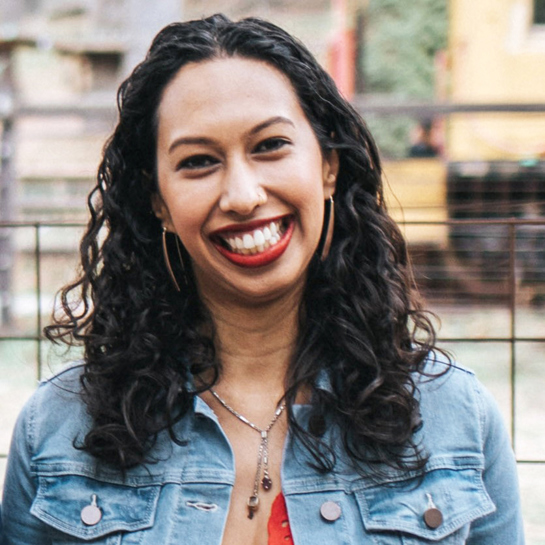
Jeffrey R. Sachs
Mathematical scientist, Merck
Jeffrey R. Sachs is a mathematical scientist who helps to accelerate and enhance vaccine discovery and development at Merck. For more information, visit his LinkedIn profile and Google Scholar page.
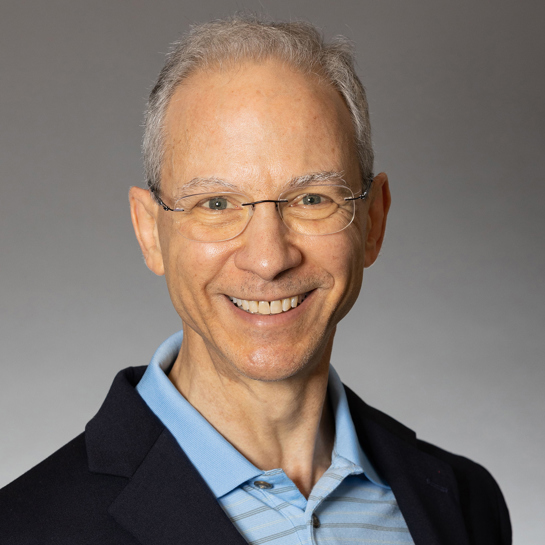
Stay Up-to-Date with Email Alerts
Sign up for our monthly newsletter and emails about other topics of your choosing.