May Prize Spotlight
Congratulations to the following 2024 recipients who will be recognized at the 2024 SIAM Conference on Imaging Science (IS24), taking place May 28-31, 2024, and the 2024 SIAM Conference on Applied Linear Algebra (LA24), taking place May 13-17, 2024.
- Nicolas Boulle and Alex Townsend - SIAM Activity Group on Linear Algebra Best Paper Prize
- Yuxin Chen, Yuejie Chi, Cong Ma, and Kaizheng Wang - SIAM Activity Group on Imaging Sciences Best Paper Prize
- John C. Urschel - SIAM Activity Group on Linear Algebra Early Career Prize
Nicolas Boulle and Alex Townsend
Nicolas Boulle and Alex Townsend are the recipients of the 2024 SIAM Activity Group on Linear Algebra Best Paper Prize. The team was awarded the prize for their paper, "Learning Elliptic Partial Differential Equations with Randomized Linear Algebra," which was published in Foundations of Computational Mathematics, Vol. 23 (2), pp. 709-739 (2023). The committee recognized their work for extending classical randomized numerical linear algebra techniques to infinite dimensions and demonstrating their application in learning solution operators for elliptic PDEs within algorithms.
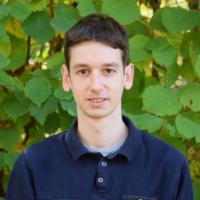
They will be recognized at the 2024 SIAM Conference on Applied Linear Algebra (LA24), taking place May 13-17, 2024, in Paris, France. Boulle will present a talk on Thursday, May 16, at 5:10 p.m. CEST.
Nicolas Boulle is a research fellow in mathematics at the University of Cambridge. He obtained a Ph.D. in numerical analysis in 2022, from the University of Oxford. His research focuses on the intersection between numerical analysis and deep learning, with a specific emphasis on learning physical models from data, particularly in the context of partial differential equations (PDEs) learning. He was awarded a Leslie Fox Prize in 2021, for his theoretical work on PDE learning. Learn more about Dr. Boulle.
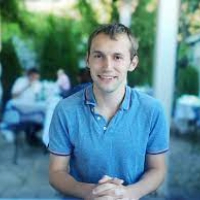
Alex Townsend is an associate professor in the mathematics department at Cornell University. His research is in applied mathematics and focuses on spectral methods, low-rank techniques, fast transforms, and theoretical aspects of deep learning. Prior to joining Cornell, Alex was an applied math instructor at Massachusetts Institute of Technology (MIT) (2014-2016) and pursued a Doctor of Philosophy at the University of Oxford (2010-2014). He was awarded the SIAM Computational Science and Engineering best paper prize, a 2022 Weiss Junior Fellowship, a 2022 Simons Fellowship, a 2021 National Science Foundation CAREER award, a 2019 SIAM Review SIGEST Paper Award, the 2018 Early Career Prize in applicable linear algebra, and the 2015 Leslie Fox Prize. Learn more about Dr. Townsend.
The authors collaborated on their answers to our questions.
Q: Why are you all excited to receive the award?
A: It is an energizing experience, and we are honored to receive the 2024 SIAM Activity Group on Linear Algebra Best Paper Prize for our work on recovering Green’s functions associated with elliptic PDEs. We are excited that this recognition may further popularize randomized linear algebra techniques in the emerging field of operator learning.
Q: Could you tell us about the research that won your team the award?
A: Our publication presents a theoretically rigorous scheme for recovering the solution operator with elliptic partial differential equations using forcing terms and solution data pairs. Solution operators are important to recover in operator learning for developing surrogate models and reduced-order models. By utilizing hierarchical low-rank structures found in Green's function, along with an infinite-dimensional singular value decomposition, we devised a randomized recovery algorithm that constructs an approximation of the Green's function with high accuracy and extremely high probability.
Our excitement about this paper stems from the fact that existing computational mathematics and linear algebra knowledge has a lot to add to the trendy field of operator learning. Usually, solution operators are recovered using deep neural networks in a supervised learning manner. Our work shows that, unlike many tasks in deep learning, solution operator learning can be remarkably data efficient, requiring surprisingly small amounts of data to develop surrogate models. In terms of practice, our work presents an opportunity to gain better insights into the quality of training datasets and can aid in the design of more effective neural network structures for learning solution operators.
Q: What does your team's work mean to the public?
A: By enabling more efficient and accurate learning of solution operators from observed data, operator learning hopes to provide new insights into the physical laws that govern systems in environmental science, engineering, and medicine. Operator learning holds great promise for improving our understanding of the physical world. The theoretical understanding of this field must be elevated to ensure robustness and trustworthiness. Ultimately, operator learning stands amongst the front runners in a paradigm shift in scientific inquiry and technological innovation, where data-driven discoveries pave the way for solving some of the most complex and critical challenges, not just thinking.
Q: What does being a member of SIAM mean to your team?
A: Being part of the SIAM community holds immense value for us, as it connects us with a vibrant network of peers and thought leaders who are equally passionate about applying mathematics to solve real-world problems. We are particularly encouraged by the active participation of world experts in computational linear algebra. Computational linear algebra is a vibrant field that sits at the center of applied mathematics. SIAM gives us opportunities for engagement and collaboration with our broad and the diverse applied mathematics community.
Interested in submitting a nomination for the SIAM Activity Group on Linear Algebra Best Paper Prize? Mark your calendar - the next call for nominations opens May 1, 2026.
Yuxin Chen, Yuejie Chi, Cong Ma, and Kaizheng Wang
Yuxin Chen, Yuejie Chi, Cong Ma, and Kaizheng Wang are the recipients of the 2024 SIAM Activity Group on Imaging Sciences Best Paper Prize. The team received the prize for their paper “Implicit Regularization in Nonconvex Statistical Estimation: Gradient Descent Converges Linearly for Phase Retrieval, Matrix Completion, and Blind Deconvolution’’, which was published in Foundations of Computational Mathematics, Vol. 20 (3), pp. 451-632 (2020). The committee awarded them for the paper in recognition of the deep understanding and analysis of the interaction between optimization algorithms and the geometry of the landscape in which they operate.
They will be recognized at the 2024 SIAM Conference on Imaging Science (IS24), taking place May 28-31, 2024 in Atlanta, Georgia, United States. Ma will present a talk on Wednesday, May 29, at 1:00 p.m. ET.
The SIAM Activity Group on Imaging Science awards this prize every two years to the author(s) of the most outstanding paper on mathematical and computational aspects of imaging published within the four calendar years preceding the year prior to the award year.
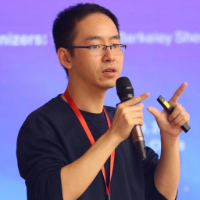
Yuxin Chen is currently an associate professor in the department of statistics and data science at the University of Pennsylvania. Before joining the University of Pennsylvania, he was an assistant professor of electrical and computer engineering at Princeton University. He completed his Ph.D. in electrical engineering at Stanford University, and was also a postdoc scholar at Stanford Statistics. His current research interests include high-dimensional statistics, nonconvex optimization, information theory, and reinforcement learning. He has received the Alfred P. Sloan Research Fellowship, the International Consortium of Chinese Mathematicians Best Paper Award, the Air Force Office of Scientific Research and ARO Young Investigator Awards, the Google Research Scholar Award, and was selected as a finalist for the Best Paper Prize for Young Researchers in Continuous Optimization. Dr. Chen has also received the Princeton Graduate Mentoring Award. Learn more about Dr. Chen.
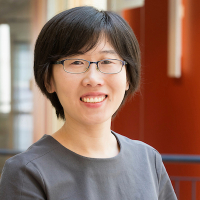
Yuejie Chi is the Sense of Wonder Group Endowed Professor of Electrical and Computer Engineering in AI Systems at Carnegie Mellon University, with courtesy appointments in the machine learning department and CyLab. She received her Ph.D. and M.A. from Princeton University, and B. Eng. (Hon.) from Tsinghua University, all in electrical engineering. Her research interests lie in the theoretical and algorithmic foundations of data science, signal processing, machine learning, and inverse problems, with applications in sensing, imaging, decision making, and AI systems. Among others, Dr. Chi received the Presidential Early Career Award for Scientists and Engineers, the SIAM Activity Group on Imaging Science Best Paper Prize, Institute of Electrical and Electronics Engineers (IEEE) Signal Processing Society Young Author Best Paper Award, and the Inaugural Signal Processing Society Early Career Technical Achievement Award for contributions to high-dimensional structured signal processing. She is a 2023 IEEE Fellow for contributions to statistical signal processing with low-dimensional structures. Learn more about Dr. Chi.
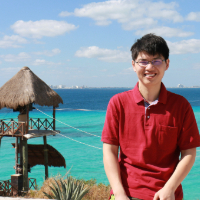
Cong Ma is an assistant professor in the department of statistics at the University of Chicago. He obtained his Ph.D. from Princeton University in 2020 and did a one-year postdoc at University of California, Berkeley. Dr. Ma is broadly interested in mathematics of data science, with a focus on reinforcement learning, transfer learning, high-dimensional statistics, and nonconvex optimization. Learn more about Dr. Ma.
Kaizheng Wang is an assistant professor of industrial engineering and operations research, and a member of the Data Science Institute at Columbia University. Wang received his Ph.D. in operations research from Princeton University in 2020. Working at the intersection of statistics, machine learning, and optimization, he has received the second place award in the 2023 INFORMS Blue Summit Supplies Data Challenge Competition. Learn more about Dr. Wang.
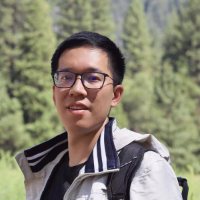
The authors collaborated on their answers to our questions.
Q: Why are you all excited to receive the award?
A: We are honored to receive the SIAM Activity Group on Imaging Sciences Best Paper Prize, as it represents recognition of the hard work and innovative ideas that went into our research. Winning this prize is a testament to the impact our work has had on the field and motivates us to continue pushing the boundaries of what is possible in this exciting area of research.
Q: Could you tell us about the research that won your team the award?
A: Prior to our work, there was significant progress in developing provably efficient nonconvex methods for tackling statistical estimation challenges, including those arising from imaging science. However, due to the highly nonconvex landscape, the state-of-the-art algorithms/results often require proper regularization procedures (e.g. trimming, projection, or extra penalization) to guarantee fast convergence. For vanilla algorithms, however, prior theory usually suggests conservative step sizes to avoid overshooting.
Our work uncovers a striking phenomenon: even in the absence of explicit regularization, nonconvex gradient descent enforces proper regularization automatically and implicitly under a large family of statistical models. In fact, the vanilla nonconvex procedure follows a trajectory that always falls within a region with nice geometry. This "implicit regularization" feature allows the algorithm to proceed in a far more aggressive fashion without overshooting, which in turn enables faster convergence. In this work, implicit regularization is established for three key statistical estimation problems: phase retrieval, low-rank matrix completion, and blind deconvolution. To prove this, we create a versatile framework for examining the paths of iterative algorithms using a leave-one-out perturbation technique.
Q: What does your team's work mean to the public?
A: First, the problems conquered in this paper permeate a diverse array of imaging and data science applications. Several representative examples include phase retrieval, a problem concerned with reconstructing a signal from intensity-only measurements, which arises in optical imaging and X-ray crystallography; low-rank matrix completion, a problem that seeks to predict missing entries of a low-rank matrix, as commonly encountered in magnetic resonance imaging and computer vision; and, blind deconvolution, a problem that aims to recover two unknown signals from their convolution and comes up in tomography and radar imaging.
Second, we establish the effectiveness of vanilla nonconvex procedures (e.g., gradient descent with large step sizes) for all three problems. This has a significant impact on practitioners. Running simple gradient methods frees the practitioners from tuning a lot of unnecessary algorithmic parameters in practice, which may not be an easy job at all.
Q: What does being a member of SIAM mean to your team?
A: SIAM provides a fantastic community to be a part of, and we are truly grateful for the opportunity. Through its diverse array of meetings, conferences, and published books, SIAM actively fosters research discussions among applied mathematicians. Our research, including the work being recognized, has greatly benefited from engaging with SIAM's events and publications.
Interested in submitting a nomination for the SIAM Activity Group on Imaging Sciences Best Paper Prize? Mark your calendar - the next call for nominations opens May 1, 2025.
John C. Urschel
John C. Urschel is the recipient of the 2024 SIAM Activity Group on Linear Algebra Early Career Prize. Dr. Urschel received the prize for his excellent work in linear algebra, using many different techniques from mathematics, such as random matrix theory, orthogonal polynomials, group theory, and optimization.
He will be recognized at the 2024 SIAM Conference on Applied Linear Algebra (LA24), taking place May 13-17, 2024, in Paris, France. He will present a talk titled “The Growth Factor in Gaussian Elimination” on Tuesday, May 14 at 6:35 p.m. CEST.
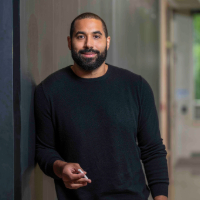
John C. Urschel is an assistant professor in the mathematics department at Massachusetts Institute of Technology (MIT) and a Junior Fellow at the Harvard Society of Fellows. Previously, he was a member of the Institute for Advanced Study. He received his Ph.D. in mathematics from MIT in 2021 under the supervision of Michel Goemans. His research is focused on fundamental problems in matrix analysis, numerical linear algebra, and spectral graph theory. Learn more about Dr. Urschel.
Q: Why are you excited to receive the award?
A: I received a great deal of support and mentorship from many people during my Ph.D., especially Michel Goemans and Alan Edelman. I was very excited to tell them both that I received this award. Michel, my Ph.D. advisor, was especially involved in the proofreading of the thesis.
Q: Could you tell us about the research that won you the award?
A: I think my specific work is quite removed from the ideas that the general non-mathematical public thinks or cares about, but my research is quite important in application. Matrix computations underpin many areas of science, and the understanding of networks through linear algebraic techniques has proven to be a powerful tool.
Q: What does your work mean to the public?
A: My research is focused on answering fundamental questions in applied linear algebra, often with implications for other areas of mathematics and computer science. My thesis focused on four topics: determinantal point processes, inverse eigenvalue problems, graph drawing, and moment-based eigenvalue algorithms. The tools used to solve these problems are quite varied in nature, including algebraic graph theory, linear algebra over finite fields, orthogonal polynomials, trace theorems, and other ideas. In each of these four stories, the hero remains the same: linear algebra, which saves the day.
Q: What does being a member of SIAM mean to you?
A. I am proud to be a member of SIAM and take part in a community that values the importance of both mathematics and computation. Simply put, SIAM is an integral part of the applied mathematics community.
Interested in submitting a nomination for the SIAM Activity Group on Linear Algebra Early Career Prize? Mark your calendar - the next call for nominations opens May 1, 2026.
Stay Up-to-Date with Email Alerts
Sign up for our monthly newsletter and emails about other topics of your choosing.