Medical Mathematics Outside of Math Departments
I had not expected to find myself in a cancer biology lab on campus, looking at a micrograph image of an immune T cell attacking a cancer cell. The image showed a partial circular shape (the T cell) adjoined to a larger circular shape (the cancer cell). “Doesn’t this look like the soap bubble problem you told me about?” asked a postdoc I had recently met. “Yes,” I answered. “Are you interested in modeling their shapes?” “No,” said the postdoc’s advisor, “but we’d like to talk to you about some other types of modeling.” Thus began my transition from differential geometry to systems medicine. I switched from studying surfaces that minimize area under constraints to studying drug regimens that maximize efficacy and minimize toxicity under constraints.
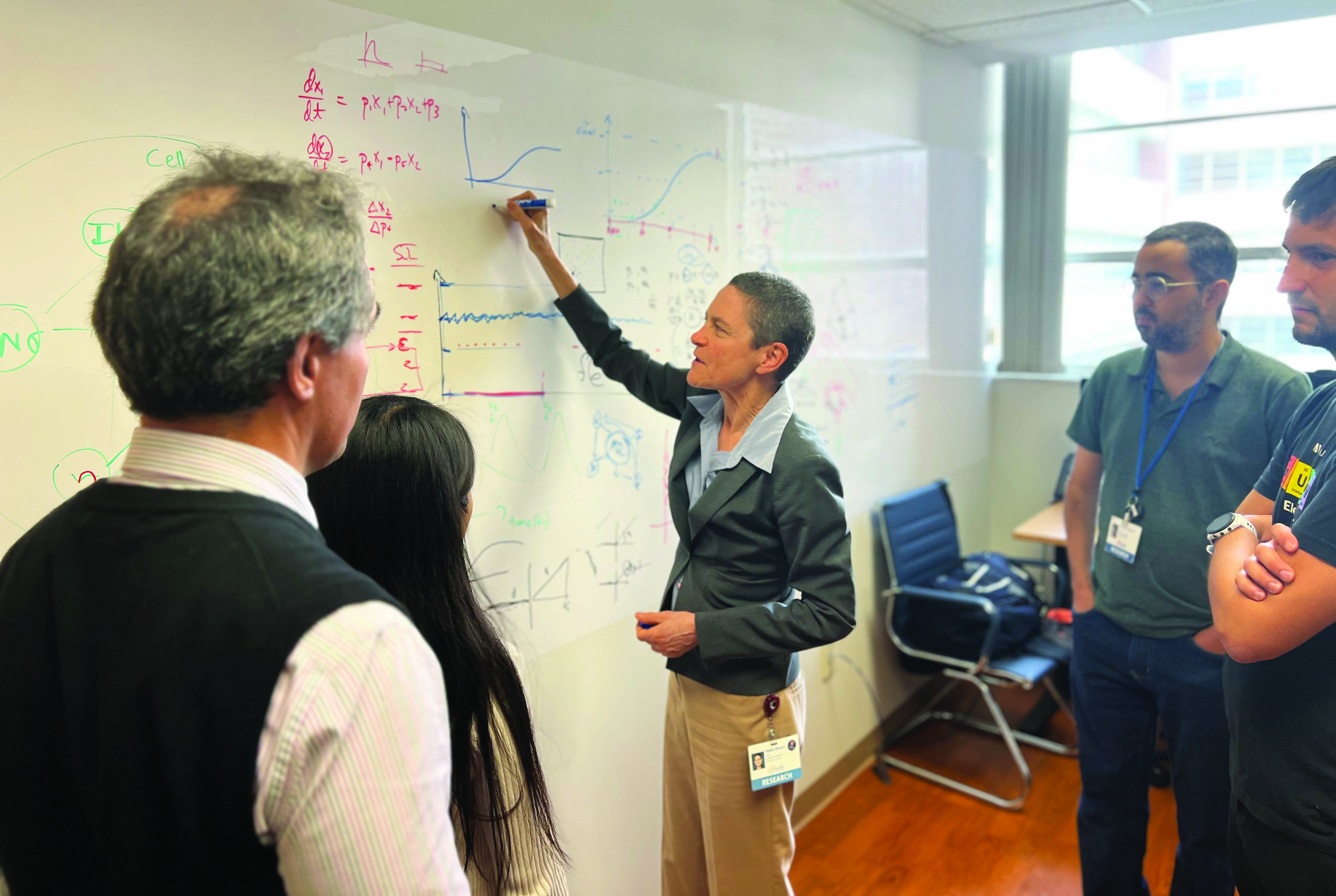
Years later, I was discussing my work on disease modeling and regimen optimization with someone else, who mentioned that their company really needed this type of expertise. I quickly received an interview and then a job offer from Genentech. Leaving academia was a difficult decision, but I took the leap and embarked on a 15-year career as a math modeler in the biotechnology/pharmaceutical (biopharma) industry. I returned to academia in 2021 and joined a group of mathematicians in the Laboratory for Systems Medicine in the College of Medicine at the University of Florida.
Here, I will share advice for early-career mathematicians who are considering working in the biopharma industry. I will describe some of the math modeling used in biopharma as well as skills that employers look for in job candidates. I will then address future modeling directions in industry, the process of returning to academia from industry, alternatives to industry, and the differences between academic and industrial careers. More information is available in [1].
Mechanistic Modeling in Biopharma
Although empirical modeling of drug concentrations has been used in biopharma since at least the 1970s, a type of mechanistic modeling called quantitative systems pharmacology (QSP) modeling is increasingly used today. A QSP model is a mathematical, mechanistic model — typically a system of ordinary differential equations (ODEs). However, it could instead consist of a system of partial differential equations or stochastic differential equations, an agent-based model, or other types of mathematical models that can mechanistically represent diseases and therapies in individuals. Many mathematical biologists in academic math departments use ODE systems to model susceptible-infected-recovered (SIR) individuals in a population. Unlike SIR-type models that track the spread of disease at the population level, QSP models are in-host models of disease dynamics within an individual, hypothetical patient. Such models focus on disease mechanisms, identifying potential therapeutic targets, and testing hypothetical or actual therapies. With adequate data for calibration, QSP models can also serve as medical digital twins [6].
QSP modeling contributed to Pfizer’s successful development of the antiviral therapy Paxlovid for patients with COVID-19. Modelers created a QSP model to simulate patient outcomes under various dosing scenarios and determined that patients should begin the drug within five days of symptom onset for best results [5, 8].
Skills Needed for QSP Modeling in Biopharma
Here are some of the capabilities and experiences that will make you a good candidate for QSP modeling positions in biopharma. I have listed them starting with the most important, based on my personal involvement with the interviewing and hiring processes:
- Experience in building and working with ODE models in relevant settings
- Familiarity with MATLAB or similar software (e.g., Python and R)
- Use of appropriate sensitivity analysis methods to assess model dependencies
- Ability to collaborate with scientists who are not modelers
- Strong presentation and writing skills
- Professionalism, including prioritizing and meeting timelines.
When interviewing for a job, applicants should discuss examples that demonstrate these specific capabilities or experiences.
The SIAM Job Board sometimes has job listings for QSP modelers, and the International Society of Pharmacometrics typically lists dozens of QSP opportunities. You can also check the career pages at top biopharma companies, or search sites like Indeed and LinkedIn for keywords such as “QSP,” “systems pharmacology,” or “math modeling.” Additionally, the Life Sciences and Dynamical Systems community forums on SIAM Engage can be good places to network with like-minded individuals.
The Future of Modeling in Biopharma
For mechanistic math modelers, the future in industry is bright! Due to the success of the methods, many companies are currently looking for qualified QSP modeling applicants. Regulatory agencies are driving an emphasis on model verification, validation, and uncertainty quantification, so modelers with the depth and rigor that comes from graduate math Ph.D. training are in high demand. There is an increase in the use of methods like sensitivity analysis (especially global methods such as the Morris method, active subspaces, partial rank correlation coefficients, extended Fourier amplitude sensitivity testing, Sobol indices, extended Sobol indices, and Shapley values); identifiability analysis (both structural and practical); uncertainty quantification; and data-based validation (including k-fold cross validation and external data) [4].
Once we have a reliable model, we can use it for various predictions. For example, we can apply optimal control to determine optimal combination drug regimens [7]. We can also simulate virtual patients and predict how a different dose regimen would impact a hypothetical patient population.
The size and complexity of QSP models make the simulation of virtual patients (one virtual patient \(=\) one feasible parameter set) a lengthy process. But such simulations are necessary for testing distinct scenarios (e.g., different dosing regimens, renally-impaired populations, or effects in children). The judicious selection of virtual patients to reduce the required computational time is an active area of research [2, 9]. Model reduction techniques and surrogate modeling can help speed up the simulation of virtual patients and more thoroughly explore the parameter space, thus providing greater confidence in outcomes of simulated patient cohorts.
The use of artificial intelligence (AI) is popular in many fields these days. Methods that employ AI may help estimate model parameters, but will they ever be able to develop reliable QSP models by combining data with knowledge from the literature? The jury is still out on this use of AI. Other empirical modeling methods are used in biopharma, many of which involve regression fitting of a specified curve to a data set. Peter Bonate’s book provides a mathematically-focused overview of such empirical modeling methods in drug development [3].
Returning to Academia
How easy is it to move from industry to academia? In my experience, most schools generally ignore the valuable knowledge that candidates could bring from their industry backgrounds and instead evaluate you as if you were an academic. This means that you need peer-reviewed research manuscripts. My industry groups generally did not allow me to publish anything related to my work projects. But thanks to the time I put in during weekends and evenings, I generated a small but steady stream of publications during my 15 years in industry. I had received a National Science Foundation grant and two teaching awards while in academia, and I spent a few of my industry years teaching biopharma modeling methods and software. I also had a long track record of mentoring and working to increase diversity in multiple settings. So when applying to return to academia, I was able to write the three statements that are required for many academic positions—research, teaching, and diversity—but still had to explain gaps in my research and teaching.
Due to these particular criteria, it can be challenging to transition to academia if you have been in industry for a long time. If you want to keep such a transition as an option, you should look for jobs with groups that are enthusiastic about publishing their work. Additionally, you can give guest lectures to students and ask them to complete evaluation forms. It may also be beneficial to make a decision about returning to academia early in your career, since a gap of a few years is not as much of an issue as 15 years.
Alternatives to Industry
What if you want an academic career but would prefer to focus on research rather than teaching? You’re in luck! There are now multiple groups of mathematicians outside of math departments that primarily focus on research. I am part of the Laboratory for Systems Medicine in the College of Medicine at the University of Florida, which is one such group. We currently have openings for faculty, with a responsibility breakdown of roughly 90 percent research and 10 percent teaching.
Several other university groups in the U.S.—including the Computational Medicine Program at the University of North Carolina at Chapel Hill and the Center for Computational Oncology at the University of Texas at Austin—have a similar focus on research and often have postdoctoral or faculty openings. Groups within medical centers include the Integrated Mathematical Oncology Department at Moffitt Cancer Center and the Mathematics in Medicine Program at Houston Methodist Hospital.
Table 1 compares typical characteristics for settings in which a mechanistic, in-host disease modeler might be employed.
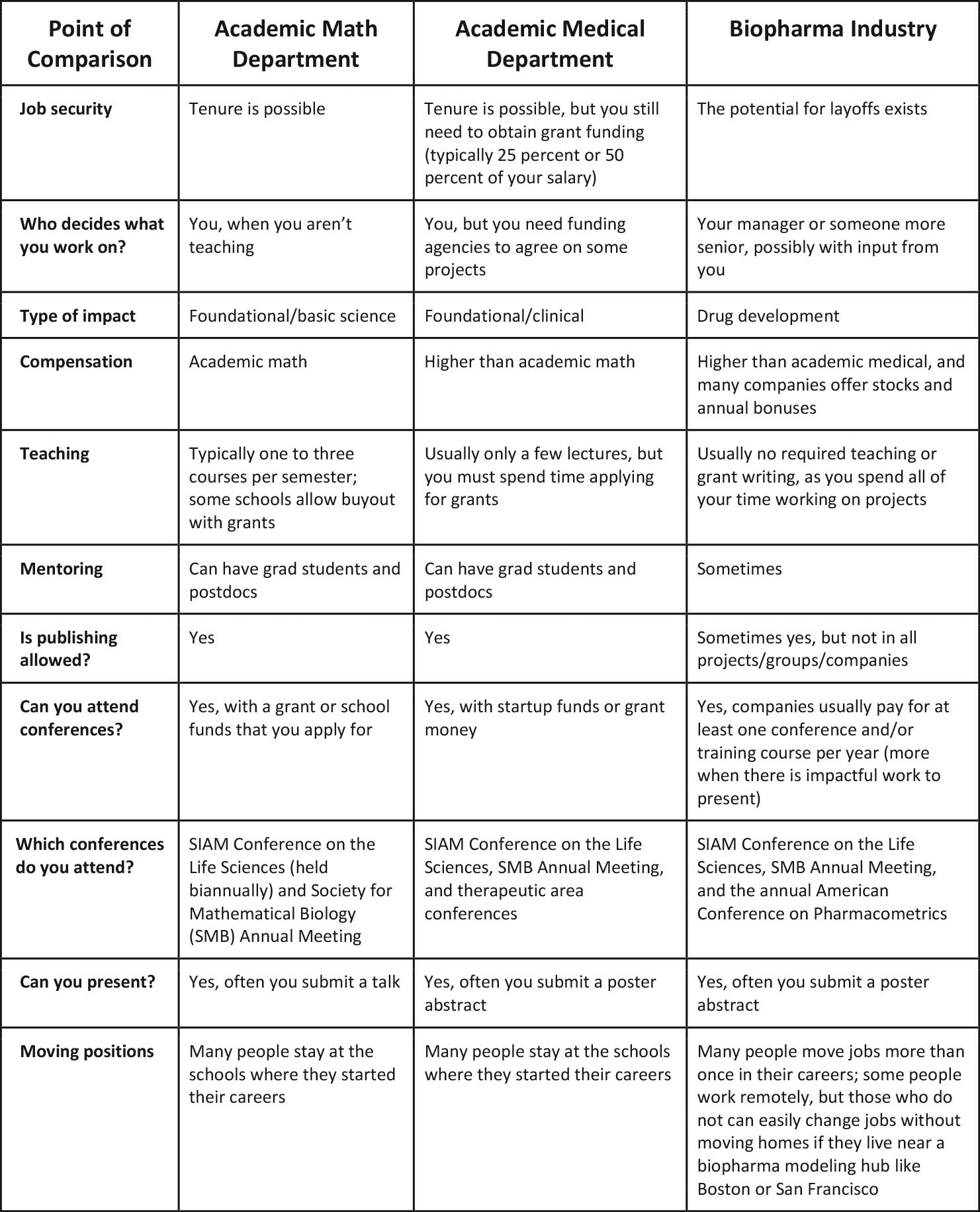
Conclusions
I have presented my own experiences and views of mathematical modeling in the biopharma industry. If you are considering a job in industry, I encourage you to talk to individuals who have worked (or are currently working) in an industry setting. You can do this by attending meetings that attract industry modelers, or career panels at SIAM conferences. You might even want to invite some of these modelers to speak in a department seminar. The SIAM Visiting Lecturer Program provides profile and contact information for applied mathematicians who work in a variety of industries.
This article shares some overlap with another article by Helen Moore, entitled “Modeling Jobs in the Biopharma Industry,” which published concurrently in the “Early Career Section” of the May issue of Notices of the American Mathematical Society.
References
[1] Allen, R., & Moore, H. (2019). Perspectives on the role of mathematics in drug discovery and development. Bull. Math. Biol., 81(9), 3425-3435.
[2] Allen, R.J., Rieger, T.R., & Musante, C.J. (2016). Efficient generation and selection of virtual populations in quantitative systems pharmacology models. CPT Pharmacometrics Syst. Pharmacol., 5(3), 140-146.
[3] Bonate, P.L. (2011). Pharmacokinetic-pharmacodynamic modeling and simulation (2nd ed). New York, NY: Springer.
[4] Braakman, S., Pathmanathan, P., & Moore, H. (2022). Evaluation framework for systems models. CPT Pharmacometrics Syst. Pharmacol., 11(3), 264-289.
[5] Dai, W., Rao, R., Sher, A., Tania, N., Musante, C.J., & Allen, R. (2021). A prototype QSP model of the immune response to SARS-CoV-2 for community development. CPT Pharmacometrics Syst. Pharmacol., 10(1), 18-29.
[6] Laubenbacher, R., Niarakis, A., Helikar, T., An, G., Shapiro, B., Malik-Sheriff, R.S., … Glazier, J.A. (2022). Building digital twins of the human immune system: Toward a roadmap. NPJ Digit. Med., 5, 64.
[7] Moore, H. (2018). How to mathematically optimize drug regimens using optimal control. J. Pharmacokinet. Pharmacodyn., 45(1), 127-137.
[8] Rao, R., Musante, C.J., & Allen, R. (2021). A quantitative systems pharmacology model of the pathophysiology and treatment of COVID-19 predicts optimal timing of pharmacological interventions. NPJ Syst. Biol. Appl., 9, 13.
[9] Rieger, T.R., Allen, R.J., Bystricky, L., Chen, Y., Colopy, G.W., Banks, H.T., … Musante, C.J. (2018). Improving the generation and selection of virtual populations in quantitative systems pharmacology models. Prog. Biophys. Mol. Biol., 139, 15-22.
About the Author
Helen Moore
Associate Professor, University of Florida
Helen Moore is an associate professor of systems medicine in the College of Medicine at the University of Florida. She is on the Board of Trustees of the International Society of Pharmacometrics and is Vice Chair of the SIAM Activity Group on Life Sciences.
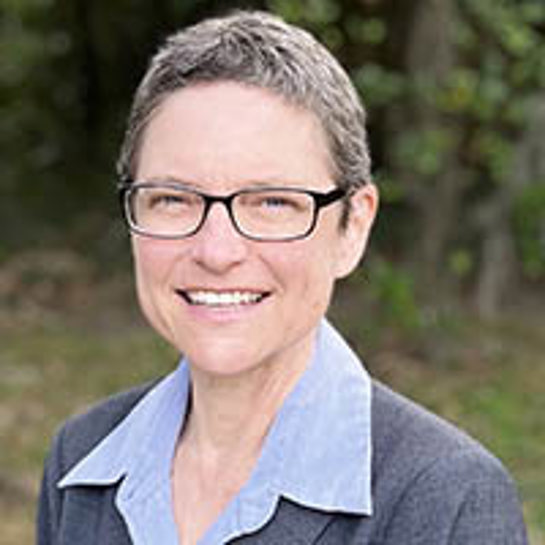
Stay Up-to-Date with Email Alerts
Sign up for our monthly newsletter and emails about other topics of your choosing.