Modeling the Impact of Rainfall Variability on Vegetation in Drylands
The global population continues to face the detrimental effects of food insecurity and climate change, with an estimated 1.3 billion people having experienced food insecurity in 2022 [4]. At the 2023 United Nations Climate Change Conference (COP28)—which took place last December in Dubai, the United Arab Emirates (UAE)—more than 150 countries endorsed the COP28 UAE Declaration on Sustainable Agriculture, Resilient Food Systems, and Climate Action. This global commitment aims to better align countries’ efforts to manage food systems and agriculture and adapt to the changing climate.
Given the urgency of this issue, existing mathematical models investigate the impact of changing rainfall levels on some of the driest regions on Earth. The Horn of Africa is a particularly well-studied location, as the distinct self-organized spatial patterns in this region are easily visible via satellite images. In fact, applied mathematician Mary Silber of the University of Chicago has employed mathematical models of terrain topography to investigate the shape of vegetation patterns and their slow dynamics in drylands [1].
Previous modeling studies use mean annual rainfall levels as a bifurcation parameter to explore vegetation patterns on decadal (and longer) timescales. However, this approach does not resolve other features of rainfall, such as the intensity and timing of storms and their variability on short timescales. “These are fast processes—maybe hours—over short times, and we’re simulating things on a yearlong timescale,” Punit Gandhi of Virginia Commonwealth University (a frequent collaborator of Silber) said.
![<strong>Figure 1.</strong> Outcomes at the end of a 100-year simulation for periodic rainfall on a one-kilometer domain that is sloped uphill towards the right. The mean annual precipitation is 16 centimeters (cm) and the mean storm depth is \(H_0=1\) cm. <strong>1a.</strong> Time series during the last two years of the simulation. <strong>1b.</strong> Spatial profiles that are derived from the simulation’s final year. The vertical axis of the top panel refers to the farthest distance that surface water travels during a rainstorm before it infiltrates the ground at point \(X\). The lower two panels are profiles for soil water and biomass. <strong>1c.</strong> The left panel is a time series of total annual rainfall (in blue), with a 1 cm contribution from each rainstorm (in orange). The middle and right panels respectively portray the five-band spacing of annually averaged soil water and biomass. The heat maps indicate less to more water or biomass, with colors ranging from yellow (at 0) to dark blue (at 8) or dark green (at 1). Figure courtesy of [3].](/media/jtqlx3yk/figure1.jpg)
To better associate mathematical models with the natural timescale of these processes, Silber, Gandhi, and Lily Liu (who was an undergraduate at the University of Chicago during this project and is currently a Ph.D. student at New York University) built upon an existing fast-slow switching model that contains fast hydrological processes and slow biomass dynamics [2]. The model’s fast subsystem is represented with partial differential equations:
\[\frac{\partial H}{\partial T}=P(T)-I(H,W;B(X))+\frac{\partial}{\partial X}(V(H;B(X))H), \tag1\]
\[\frac{\partial W}{\partial T}=I(H,W;B(X)), \tag2\]
where \(H\) is the surface water height, \(W\) is the soil water column height, and \(B\) is the biomass density. Here, \(H(X,T)\) evolves on a short timescale of rain events, \(B(X,T)\) evolves on a long timescale between rain events, and \(W(X,T)\) corresponds to several processes that act on both fast and slow timescales. The first, second, and third terms on the right side of \((1)\) respectively denote precipitation, soil infiltration, and advection. The infiltration rate \(I\) is modeled as a function of surface water height, soil water column height, and biomass density. The important positive feedbacks between biomass and soil moisture distribution come from \(I\) (which is higher when biomass is present) and the flow speed function \(V\) (which is slower when vegetation is present, allowing more time for infiltration).
Biomass and soil water evolve on a slow timescale that is associated with plant growth; they are governed by
\[\frac{\partial W}{\partial T}= -(L+\Gamma B)W+D_W\frac{\partial^2W}{\partial X^2}, \tag3\]
\[\frac{\partial B}{\partial T}=C\bigg(1-\frac{B}{K_B}\bigg)\Gamma BW - MB +D_B\frac{\partial^2B}{\partial X^2}. \tag4\]
This subsystem evolves the soil water \(W(X,T)\) and is initialized by the post-storm distribution \(W(X)\) and biomass density \(B(X,T)\). The two terms on the right side of \((3)\) denote evapotranspiration and diffusion, the parameter \(L\) represents the evaporation rate, and \(\Gamma B\) signifies the transpiration rate. In \((4)\), the three right terms respectively denote growth, death, and dispersal. The death rate \(M\) is constant and the dispersal is modeled via linear diffusion. Transpiration dictates the biomass growth rate, whose efficiency is set by \(C\).
In a recent paper that published in the SIAM Journal of Applied Dynamical Systems last year, Gandhi, Liu, and Silber expanded this existing framework to create a mathematical model that explores rainfall pattern variability over shorter timescales and its corresponding impact on the formation of vegetation patterns in dry ecosystems [3]. The climatology of the Horn of Africa inspired this new pulsed-precipitation model, which represents rainstorms as instantaneous “kicks” to the soil water — i.e., Dirac delta function impulses that deposit a uniform layer of water on the surface.
![<strong>Figure 2.</strong> Results at the end of a 100-year simulation with stochastic rainfall, with similar parameters to Figure 1. <strong>2a.</strong> Time series during the last two years of the simulation. <strong>2b.</strong> Spatial profiles of surface water travel distance, soil water, and biomass that are derived from the simulation’s final year. <strong>2c.</strong> Time series of annual rainfall and spacetime plots of annually averaged soil water and biomass. Figure courtesy of [3].](/media/1xiayxot/figure2.jpg)
Though they retained the same reaction-diffusion model in the slow subsystem, the team made two significant changes to the fast subsystem that allowed them to determine the output soil moisture distribution \(W(X)\) in a closed-form expression. First, the term \(P(T)\) in \((1)\) now signifies rain events that instantaneously deposit a uniform water column of height \(H_0\). The timing and strength of these precipitation pulses are the random variables in the stochastic simulations. And second, the infiltration rate \(I\) is now given by
\[I(H,B(X))\equiv K_I\bigg(\frac{B(X)+fQ}{B(X)+Q}\bigg)\Theta(H). \tag5\]
The Heaviside unit step function \(\Theta(H)\) indicates that the infiltration is independent of soil saturation and is modeled as a simple on-off switch in the presence or absence of surface water, assuming that \(\Theta(0)=0\).
Using these modifications, the researchers reformulated the fast-slow model to a pulsed-precipitation framework in which the rainstorm instantaneously deposits water on the surface at a fast timescale, after which an intervening dry period evolves on a slow timescale.This new model reveals interesting insights about rainfall patterns. Figure 1a illustrates the time series for periodic rainfall during the last two years of a 100-year simulation. Figure 1b depicts spatial profiles—derived from the simulation’s final year—that compare soil water and biomass given the farthest distance that surface water travels before infiltration during a rainstorm. Figure 1c then offers a time series of total annual rainfall, along with the five-band spacing of annually averaged soil water and biomass. Similarly, Figure 2 presents the simulated results for stochastic rainfall in the form of a stochastic traveling wave solution that fluctuates based on season and rainfall variability.
![<strong>Figure 3.</strong> Snapshots of annual rainfall, soil water, and biomass at different domain sizes \(L\) for periodic and stochastic rainfall. In each scenario, the vertical axis spans 100 years and the horizontal rainfall axis spans 40 centimeters (cm). The model parameters are fixed at a mean annual precipitation of 16 cm and a mean storm depth of \(H_0=1\) cm. Figure courtesy of [3].](/media/gxybsbpd/figure3.jpg)
Figure 3 compares patterns under periodic and stochastic rainfall in a smaller domain than Figures 1 and 2. The team changed the size of the periodic domain \(L\) to enforce different band spacings and restricted \(L\) to less than 250 meters (m). When \(L\) ranges from 100-167 m or falls under 59 m, the simulations do not exhibit a traveling wave state with periodic rainfall. But for these values of \(L\) in the case of stochastic rainfall, the simulations do show a stochastic traveling wave. “It was surprising to find that under periodic rainfall, we sometimes saw crazy behavior,” Gandhi said.
Gandhi and his collaborators utilized linear stability analysis techniques to explain these differences. Using the periodic rainfall scenario with uniform vegetation in the pulsed-precipitation model, they introduced a small sinusoidal perturbation to the initial state to see whether it would grow into a full vegetation band. The researchers found that for mean annual precipitation (MAP) values that were greater than roughly 43 centimeters, the perturbation dampens and yields uniform vegetation (see Figure 4). But as the MAP decreases to lower values (which is expected in arid regions like the Horn of Africa), the analysis—in the presence of spatially periodic perturbation—reveals resonance tongues across multiple regions with growing vegetation patterns.
This finding proves that the regularity of “artificial” periodic rainfall patterns could lead to spatial resonance that in turn controls the preferred spacing of the vegetation bands. Specifically, vegetation band spacing is determined by the distance that surface water travels in the time that it takes for the precipitation pulse to fully infiltrate the soil. “The important thing to note is that the surface hydrology, which happens on timescales of seconds or minutes during storms, controls this large-scale vegetation pattern that evolves over decades,” Gandhi said. Further simulations revealed that even during stochastic rainfall, the distance that water travels on the surface is a key component of pattern formation.
![<strong>Figure 4.</strong> Results from the linear stability analysis. Figure courtesy of [3].](/media/ub3hokid/figure4.jpg)
The pulsed-precipitation model reveals many interesting MAP insights, but a major question prevails: How will climate change influence these patterns? To investigate, the collaborators explored the implications of storm depth and rainy season duration on vegetation patterns. They found that longer rainy seasons—which correspond to shorter dry intervals, during which the biomass can survive without rain—could increase the vegetation’s mean survival time. Similarly, more frequent but less intense storms—which are associated with a reduction in seasonal rainfall variability—could help prevent vegetation collapse.
The disappearance of spatial resonance with stochasticity in the rainfall model presents an intriguing mathematical challenge that merits further exploration. Many math modelers study vegetation patterns in vulnerable ecosystems under a changing climate, but Silber notes that this focus alone is not enough. “We also need to be thinking about water because it’s a tightly coupled system,” she said. “You have to think about the resource and the time in which water is acting.”
While previous vegetation models have drawn tremendous inspiration from historical papers, Gandhi, Silber, and Liu’s efforts highlight the vast potential for interdisciplinary collaborations. For instance, their current work could spur investigations into a variety of research problems, such as the use of mathematical models to inform measurable quantities for remote sensing of moisture, or model validation via feedback from field-based hydrology monitoring efforts. Most importantly, however, this study highlights the intricate interplay between the timescales of fast-acting hydrological processes and slowly evolving dryland vegetation patterns. A thorough understanding of these interactions may help future researchers assess the resilience of dryland ecosystems.
References
[1] Chandrasekaran, L. (2017). Modeling vegetation patterns in vulnerable ecosystems. SIAM News, 50(2), p. 6.
[2] Gandhi, P., Bonetti, S., Iams, S., Porporato, A., & Silber, M. (2020). A fast-slow model of banded vegetation pattern formation in drylands. Phys. D: Nonlinear Phenom., 410, 132534.
[3] Gandhi, P., Liu, L., & Silber, M. (2023). A pulsed-precipitation model of dryland vegetation pattern formation. SIAM J. Appl. Dyn. Sys., 22(2), 657-693.
[4] Zereyesus, Y.A., & Cardell, L. (2022, November 8). Global food insecurity grows in 2022 amid backdrop of higher prices, Black Sea conflict. U.S. Department of Agriculture: Amber Waves. Retrieved from https://www.ers.usda.gov/amber-waves/2022/november/global-food-insecurity-grows-in-2022-amid-backdrop-of-higher-prices-black-sea-conflict.
About the Author
Lakshmi Chandrasekaran
Freelance science writer
Lakshmi Chandrasekaran holds a Ph.D. in mathematical sciences from the New Jersey Institute of Technology and a master’s degree in science journalism from Northwestern University. She is a freelance science writer whose work has appeared in MIT Technology Review, Quanta, Science News, and other outlets.
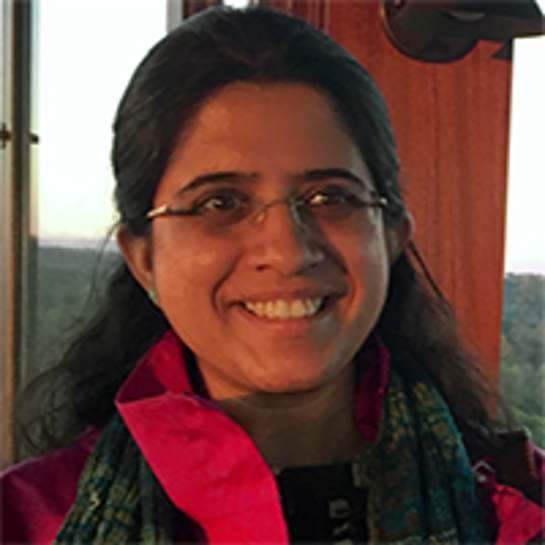
Stay Up-to-Date with Email Alerts
Sign up for our monthly newsletter and emails about other topics of your choosing.