Moving Memoir by an AI Pioneer
The Worlds I See: Curiosity, Exploration, and Discovery at the Dawn of AI. By Fei-Fei Li. Flatiron Books, New York, NY, November 2023. 336 pages, $29.99.
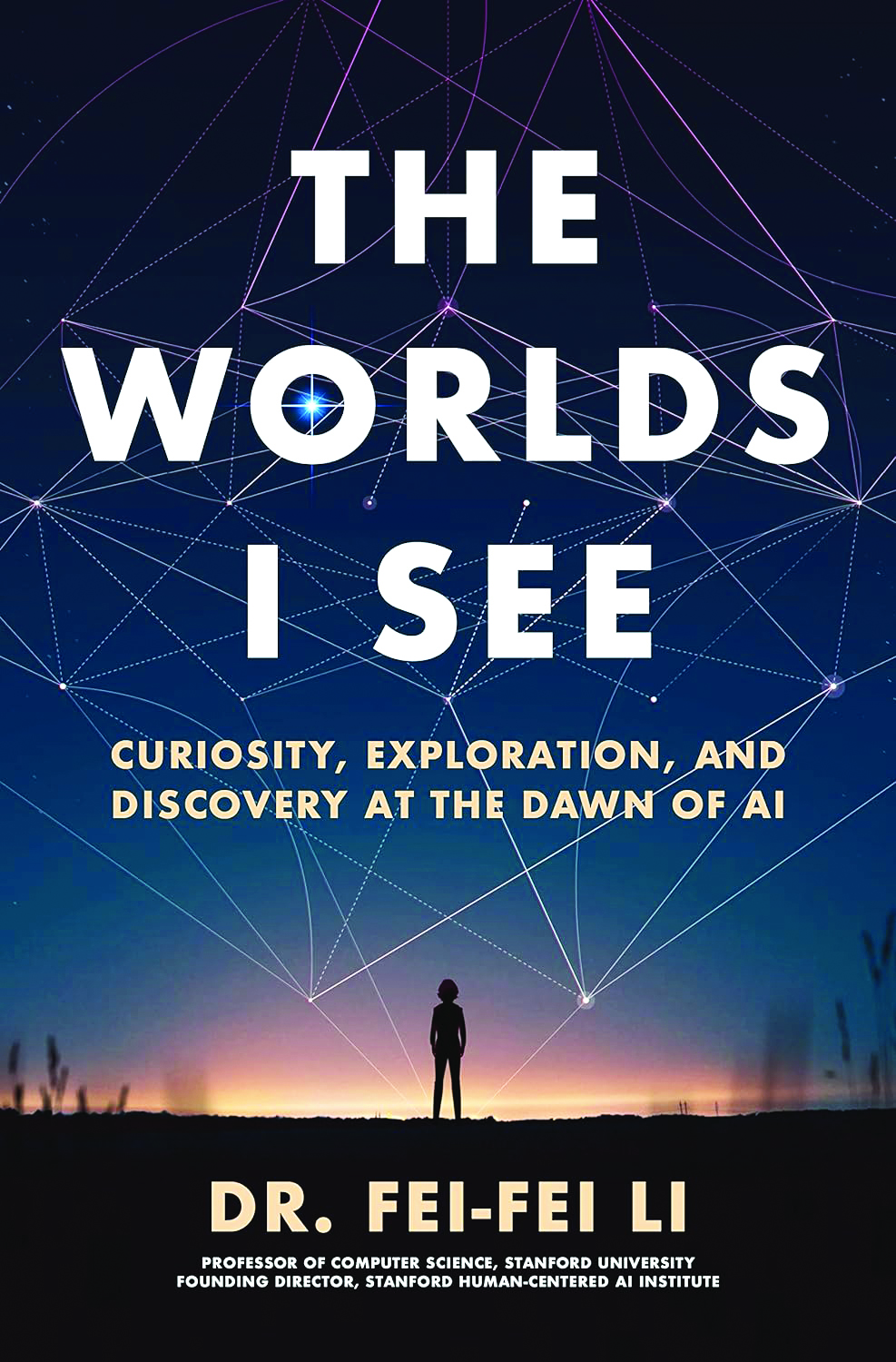
The extraordinary advances in artificial intelligence (AI) technology over the last decade are largely due to three factors: (i) Improvements in machine learning algorithms, primarily deep learning but also reinforcement learning and transformer architectures; (ii) improvements in computer hardware, particularly graphics processing units; and (iii) the availability of enormous datasets to train AI systems. Fei-Fei Li, a professor of computer science at Stanford University, is a pioneer in the creation of large, high-quality datasets for AI—particularly for computer vision—and the art of building them via crowdsourcing. The Worlds I See: Curiosity, Exploration, and Discovery at the Dawn of AI is Li’s memoir of her life and labors.
In terms of literary quality, I don’t think I’ve ever read a better-written scientific biography or memoir than The Worlds I See (some credit is presumably due to Li’s writing partner, Alex Mitchell). Li’s portraits of her parents and Bob Sabella—her high school math teacher and close friend until his untimely death—are warmhearted, insightful, moving, and vivid. Equally compelling is her account of the difficulties that she and her family faced immediately after emigrating to the U.S.
The scientific exposition in The Worlds I See is always clear and engaging, though not especially deep; in fact, the book is intended for a general readership. A long digression on zoologist Andrew Parker’s theory of the centrality of vision in animal evolution during the Precambrian era becomes almost lyrical:
Photosensitivity was a turning point in the history of life on Earth. By simply letting the light in—to any degree, no matter how dim or formless—our evolutionary predecessors recognized, for the first time, that there was something beyond themselves. And, more urgently, they saw that they were engaged in a struggle to survive, with more than one possible outcome. They were awakening to a harsh environment in which threats and opportunities alike abounded, competition for resources was increasing, and their own actions meant the difference between eating and being eaten.
Li wonderfully conveys the atmosphere of scientific study and research and the intense satisfactions, disappointments, thrills, and frustrations that accompany it. She writes without false modesty or self-aggrandizement. She is forthright about her abilities, hard work, and accomplishments but simultaneously upfront regarding her own good fortune and debts to predecessors, teachers, colleagues, and students.
Li was born in Beijing in 1976 and grew up in Chengdu, China. When she was 12, her family emigrated to the U.S. and settled in Parsippany, N.J., where they lived in a cramped apartment on her parents’ small incomes as shop assistants. While completing high school and learning English as a second language, Li combined a modest income from a restaurant job with housekeeping and dog walking. Fortunately, she found a mentor and friend in her math teacher, Bob Sabella. In her final year of high school, she was accepted to Princeton University with a near-full scholarship. Although Li felt socially isolated at Princeton—in part because of her classmates’ wealth—she enjoyed the academics and majored in physics. Her first experience with scientific research occurred during a summer program at the University of California, Berkeley, where she studied the neuroscience of vision in cats.
While she was in college, Li’s parents bought a dry cleaning shop. Li served as a translator between her parents and the customers and worked in the shop on weekends and during breaks. She sometimes considered taking a well-paid job as a “quant” at a financial firm to alleviate some of her family’s financial strain, but her mother insisted that she continue her education and pursue her dream of becoming a scientist.
After earning her undergraduate degree, Li pursued her Ph.D. at the California Institute of Technology. She conducted psychological experiments on human vision and built computational models under the direction of Pietro Perona and Christof Koch. While working on her thesis in 2004, Li hand-constructed a dataset that contained more than 9,000 images from 101 categories; at the time, it was the largest image dataset ever built. Upon graduation, she obtained a sequence of faculty positions at the University of Illinois, Princeton, and then Stanford.
In 2006, Li conceived the idea of an image dataset on a much vaster scale: 30,000 categories with a total of several million images. She began to pursue this project with then-Ph.D. student Jia Deng (now an associate professor at Princeton University). It was both a risky and visionary undertaking — the AI community did not foresee the potential impact of large datasets, and “big data” was not yet a buzzword. As such, most of Li’s colleagues discouraged the effort. In fact, it initially seemed impossible; a few months into the project, Deng estimated that it would take 19 years to complete. However, a breakthrough came when the duo realized that they could proceed much more quickly by crowdsourcing the work via the Amazon Mechanical Turk platform.
In 2009, Li and Deng released ImageNet: an image database with 22,000 categories of 15 million total images that were divided into a published training set and an unpublished test set. Although ImageNet is currently the gold standard for the training and evaluation of AI vision systems, the research community’s initial reaction was tepid. Deng and Li’s corresponding paper was accepted at a conference as a poster presentation rather than a talk, and the initial results from systems that trained on ImageNet were not significantly different from those that trained on earlier, much smaller datasets. But the tides turned in 2012 when Alex Krizhevsky, Ilya Sutskever, and Geoffrey Hinton trained their own neural-network-based system (called AlexNet) on ImageNet, achieving dramatically better results than with any competing system. As the saying goes, the rest is history. The deep learning technology that AlexNet pioneered—empowered by enormous datasets of all kinds—has since served as the foundation for almost every noteworthy advance in AI.
Although Li does not discuss it at any length, one of the most striking aspects of ImageNet (and of some of Li’s more recent projects, such as the Visual Genome dataset) is the complexity and sophistication of her use of crowdsourcing technology. Li carefully designed and monitored the multi-step process to obtain high-quality tags from crowd workers, refining it through multiple iterations. Some other AI researchers who have utilized crowdsourcing to develop large datasets have been content to give crowd workers a problem and accept their answers at face value — an approach that does not yield results of comparable quality.
Towards the end of The Worlds I See, Li describes a series of extended visits to a hospital emergency room to investigate potential uses of AI technology. The book clearly and movingly describes Li’s deep admiration for dedicated, overworked, and hurried medical professionals; her empathy for their concerns about cameras with AI technology in their workspaces; and her willingness to learn from them rather than immediately present her own ideas of what they should want. This is a model that all types of computer experts should remember and emulate.
As a memoir, The Worlds I See could hardly be better. But as a historical narrative, it does have gaps. There is no index, no footnotes, and no bibliography, and the dates of certain events are often unclear. The text offers no guidance for the lay reader who wants to learn more, and no references—often not even titles—for the papers that Li discusses, including her own works. She praises a textbook titled Vision Science: Photons to Phenomenology, but I had to google it to learn that the author is Stephen Palmer. There are also no illustrations, which is somewhat astonishing for a book about vision.
I noticed more substantive omissions as well. For example, the text does not mention neuroscientist David Marr of the Massachusetts Institute of Technology, who was a leading figure in both cognitive and AI vision. Perhaps by the time Li entered the field in the late 1990s, Marr’s work was too outdated to be useful to her.
Closer to home—both Li’s home and mine, in different ways—is the exclusion of the first large image dataset that I ever encountered, which marked my initial intimation that large datasets might be central to the future of AI. In 2007, I attended a talk by Antonio Torralba about his forthcoming paper with Rob Fergus (now my colleague at New York University) and William Freeman, titled “80 Million Tiny Images: A Large Data Set for Nonparametric Object and Scene Recognition” [1]. The trio had created a dataset that contained 79 million \(32 \times 32\) resolution images within 75,000 categories, then demonstrated the sufficiency of nearest-neighbors search for what were fairly good levels of accuracy at the time (this was four times as many images and three times as many categories as ImageNet, which published two years later). The paper began with a dramatic assertion about the value of large datasets: “With overwhelming amounts of data, many problems can be solved without the need for sophisticated algorithms.” In the end, the tiny image dataset was much less impactful than ImageNet; the process of tagging images by category was far less precise, and the coarse images were useless for the training of high-quality vision systems. Nevertheless, it certainly deserves a mention in a history of large image datasets for AI vision systems.
Despite these minor flaws, The Worlds I See is an extraordinarily valuable and beautiful work. It offers an account of contemporary scientific research at its best and exemplifies the power of scientific memoir.
References
[1] Torralba, A., Fergus, R., & Freeman, W.T. (2008). 80 million tiny images: A large data set for nonparametric object and scene recognition. IEEE Trans. Pattern Anal. Mach. Intell., 30(11), 1958-1970.
About the Author
Ernest Davis
Professor, New York University
Ernest Davis is a professor of computer science at New York University's Courant Institute of Mathematical Sciences.