Multiscale Models Shed Light on Tuberculosis
As demonstrated by the ongoing COVID-19 pandemic, a thorough understanding of infectious diseases requires data and models on multiple interconnected levels. Epidemiology addresses population-level issues, transmission models describe individuals within their environments, and a variety of biomedical approaches help researchers comprehend the way in which pathogens infiltrate the body — and the body’s ability to fight back.
Tuberculosis (TB) is one of the deadliest infectious diseases in the world. It accounts for roughly 1.5 million deaths per year and causes the most HIV-related casualties. While decision-makers know in principle how to slow the spread of certain illnesses, TB is more stubborn than most. “TB is unique compared to many other diseases and the way we treat them,” Denise Kirschner, a mathematical biologist at the University of Michigan Medical School, said. During her plenary talk at the hybrid 2022 SIAM Conference on the Life Sciences (LS22), which took place concurrently with the 2022 SIAM Annual Meeting in Pittsburgh, Pa., this July, Kirschner described the major challenges that surround TB’s characterization.
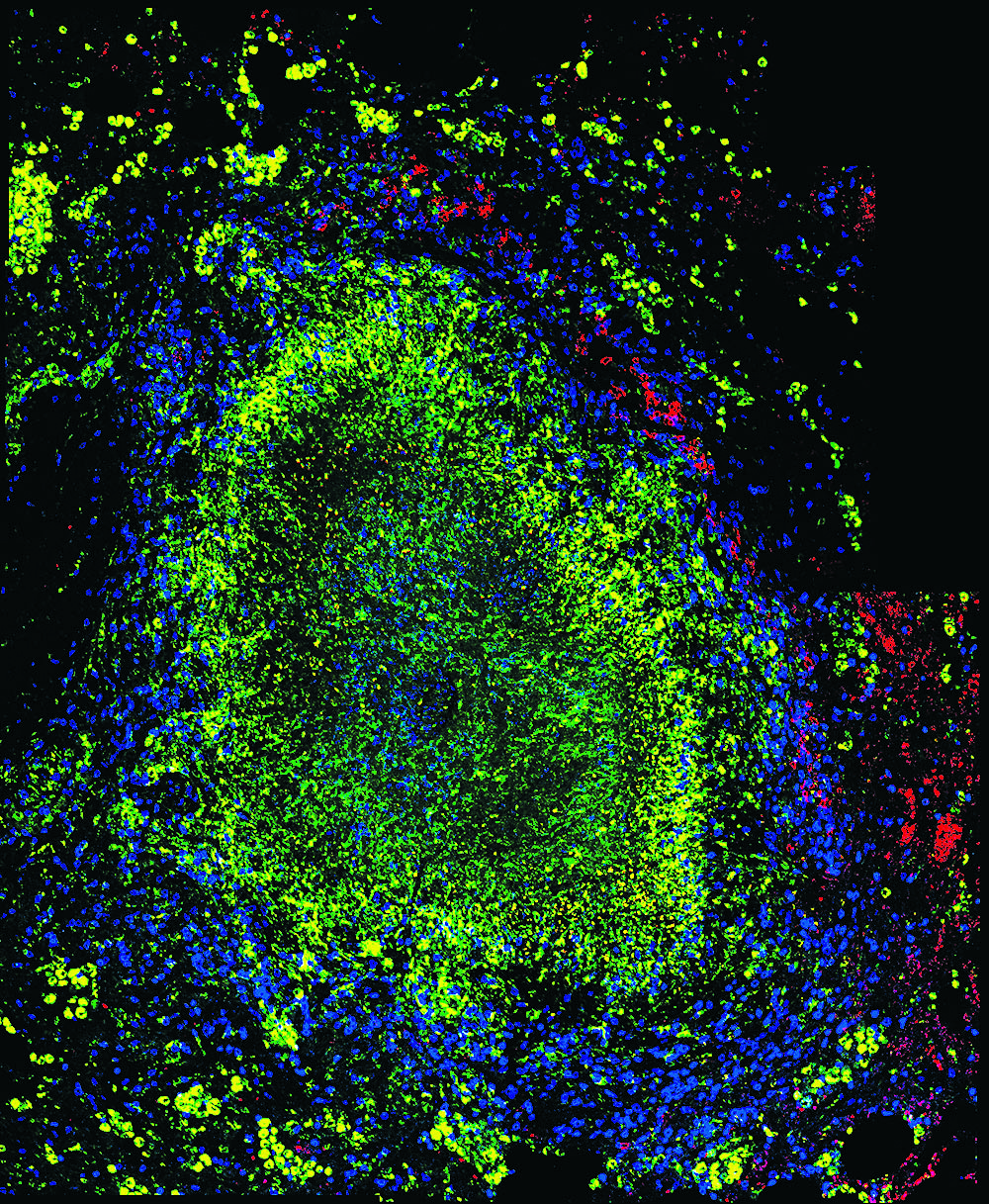
“Bacteria induce the formation of structures in the lungs known as granulomas,” she said. “These structures are important, and how cells move around within the granuloma structure is important.” Granulomas are roughly spherical clusters of immune cells that form around invading bacteria, most likely as an immunological response (see Figure 1). While the human body builds these structures in response to certain other types of infection as well, their presence in the case of TB partly explains why many people who are exposed to Mycobacterium tuberculosis bacteria remain asymptomatic.
TB is notoriously challenging to treat, and granulomas are partly responsible for this difficulty because most of the pathogens are isolated from the rest of the body. An infected lung might have between 10 and 30 granulomas, which generally do not seem to interact directly with each other. Medical data on patients and experiments on monkeys have revealed several distinct types of granulomas, including stable clusters wherein the surrounding cells contain bacterial growth, and broken granulomas that spill bacteria into the rest of the lung.
“Only one granuloma has to go south to cause active disease,” Kirschner said. “We have to understand what ‘go south’ means, and usually it means the granuloma is unable to control bacterial growth. Our goal is to really determine which factors within the granuloma lead to overgrowth, control, or clearance of the bacteria.”
Granular Approach to Granulomas
TB’s complexity requires that Kirschner and her collaborators simultaneously build models on multiple scales that range from molecular and cellular interactions to whole-lung and whole-patient analyses. The current state of the art involves agent-based models that simulate the way in which independent “agents” organize into larger associations. In the context of Kirschner’s TB research, these agents can take the form of granulomas, individual cells (bacteria and T-cells), and even regulatory proteins known as cytokines and chemokines. “We are interested in starting at the scale of most interest, where the data is most plentiful,” she said. “In this case, that scale happened to be the granuloma. We let the biology drive how deep we drill down and what the biological questions are.”
In other words, the unique nature of granulomas means that they are located at the metaphorical center of the picture; their formation, growth, propagation, and fate hold the key to modeling and hopefully treating TB. Many of the multiscale simulations that Kirschner and her team developed begin at the granuloma, then extend in both directions to encompass smaller- and larger-scale phenomena. The lung comprises the overall environment of these models, and the group tests the modeling outcomes against biological data from either TB patients or monkeys — which provide the closest known analog to humans.
To complicate matters, M. tuberculosis bacteria can also infect immune system cells. As such, complete multiscale models should include blood vessels and the lymph nodes that produce T cells to fight the infection. Simply put, TB simulations must span wide spatial and temporal resolutions and employ sophisticated tuning to capture the right details in every regime.
The earliest agent-based granuloma model describes bacteria, immune cell populations, and protein responses on a two-dimensional (2D) lattice [2]. This simulation also breaks time into discrete chunks via a time scale that is based on reasonable biological constraints of cell division and protein decay rates. The resulting model contains four basic entities—bacteria that reside outside of cells, T cells, macrophages, and chemokine levels—with 27 parameters that researchers either obtain from the simulation or measure experimentally. The generic form of the stepwise equations within each lattice box is
\[\omega_k(t+1)=\omega_k(t)+f_k(\omega_k, \omega_j),\]
where \(\omega_k\) is one of the entities and \(f_k\) is a nonlinear term that describes the interactions between entities.
Despite the number of simplifying assumptions, this model spontaneously generates granulomas unprompted. But cell motion in three dimensions is far more complicated than in two dimensions, and hence extending this model leads to prohibitively long computer runtimes. Though 2D models are still useful in some contexts—“Should we feel guilty about this?” Kirschner quipped during her presentation—scientists must look for other possible angles to the problem if they wish to involve biological experiments.
A more recent, promising approach treats granulomas themselves as the agents on a discretized model of the lung [3]. Louis Joslyn, a former graduate student in Kirschner’s group, worked with Kirschner’s colleagues to model growth, reproduction, and decay of bacteria, various cell types, and proteins with 15 coupled nonlinear ordinary differential equations for each block of the lattice:
\[\frac{d\omega_k}{dt}=F_k(\omega_k, \omega_j),\]
with nonlinear interaction terms \(F_k\). The 15 entities \(\omega_k\) include cell division, cell death, bacterial growth, and protein decay, among other factors (see Figure 2).
Early results of this technique have allowed Kirschner and her collaborators to link specific biomarkers in the blood of infected subjects with early immune responses [1]. She is thus hopeful that the model might improve future therapeutic outcomes.
![<strong>Figure 2.</strong> Simulated lungs with tuberculosis (TB) granulomas that were created using an agent-based model. The lungs on the left have a latent asymptomatic infection, while the lungs on the right are experiencing full-blown TB symptoms because the granulomas have decayed and spilled their bacteria. Figure courtesy of [1].](/media/ssiegflf/figure1.jpg)
From Models to Treatment
Roughly 25 to 33 percent of the global population carries M. tuberculosis bacteria (mostly without symptoms), and poorer countries bear a heavier burden from the disease. M. tuberculosis also grows very slowly by bacterial standards, producing a new generation every eight to 24 hours. This relatively sedate growth rate means that researchers can only detect growth in the laboratory after one to six weeks, depending on the method of observation.
To further complicate matters, the widely used TB vaccine does not effectively prevent transmission or infection. In addition, antibiotic treatment is a lengthy and expensive process. Due to the duration of treatment and unpleasant side effects, many patients do not complete the entire course of antibiotics; in response, some strains of TB have become drug resistant. “We have to use multiple drugs for long periods of time because it’s really hard to penetrate granulomas,” Kirschner said. “We need to shorten the treatment, make the drugs less toxic so there are not as many side effects, and maybe reduce the number of drugs that one takes to make them more cost effective.”
During her presentation at LS22, Kirschner noted that researchers essentially identified the current four-drug, nine-month regimen through trial and error. Mathematical models of infected lungs could help scientists determine why the four antibiotics do not work individually but are somehow effective in combination.
Kirschner reiterated that biology and medicine are the primary drivers of her mathematical models. “They’re very iterative for many reasons,” she said. “As we learn that certain things are important, we may go back and fine-grain the model in places where we coarse-grained it earlier. And biologists may discover the absence of something they now know is really crucial, so we want to add that in.”
After all, while studying TB in the abstract is interesting and useful, the ultimate goal is to save lives and improve treatment outcomes. Catching infections early, controlling bacteria before they burst out of granulomas, and developing new therapies are ambitious goals, but the stakes are high. Kirschner hopes that biology-based whole-organ—or even whole-body—disease models will lead to new frontiers in vaccination and drug development for TB.
References
[1] Joslyn, L.R., Linderman, J.J., & Kirschner, D.E. (2022). A virtual host model of Mycobacterium tuberculosis infection identifies early immune events as predictive of infection outcomes. J. Theor. Biol., 539, 111042.
[2] Segovia-Juarez, J.L., Ganguli, S., & Kirschner, D. (2004). Identifying control mechanisms of granuloma formation during M. tuberculosis infection using an agent-based model. J. Theor. Biol., 231(3), 357-376.
[3] Wessler, T., Joslyn, L.R., Borish, H.J., Gideon, H.P., Flynn, J.L., Kirschner, D.E., & Linderman, J.J. (2020). A computational model tracks whole-lung Mycobacterium tuberculosis infection and predicts factors that inhibit dissemination. PLOS Comput. Biol., 16(5), e1007280.
About the Author
Matthew R. Francis
Freelance science writer
Matthew R. Francis is a physicist, science writer, public speaker, educator, and frequent wearer of jaunty hats. His website is bsky.app/profile/bowlerhatscience.org.
Stay Up-to-Date with Email Alerts
Sign up for our monthly newsletter and emails about other topics of your choosing.