New Inverse Reinforcement Learning Technique Examines Cancer Cell Behaviors
Similar types of cells typically behave heterogeneously in the same environment, despite their resemblances. A certain amount of heterogeneity in cell dynamics is beneficial because it drives the patterns that generate normal tissues and organs with high levels of functionality. Abnormal heterogeneity, however, is a hallmark of cancer and other diseases (see Figure 1). During the 2024 SIAM Conference on Mathematics of Data Science, which is currently taking place in Atlanta, Ga., Xun Huan of the University of Michigan introduced a novel form of inverse reinforcement learning (IRL) that utilizes the Fokker-Planck equation (FPE) to understand the rationale behind heterogenous cancer cell behaviors.
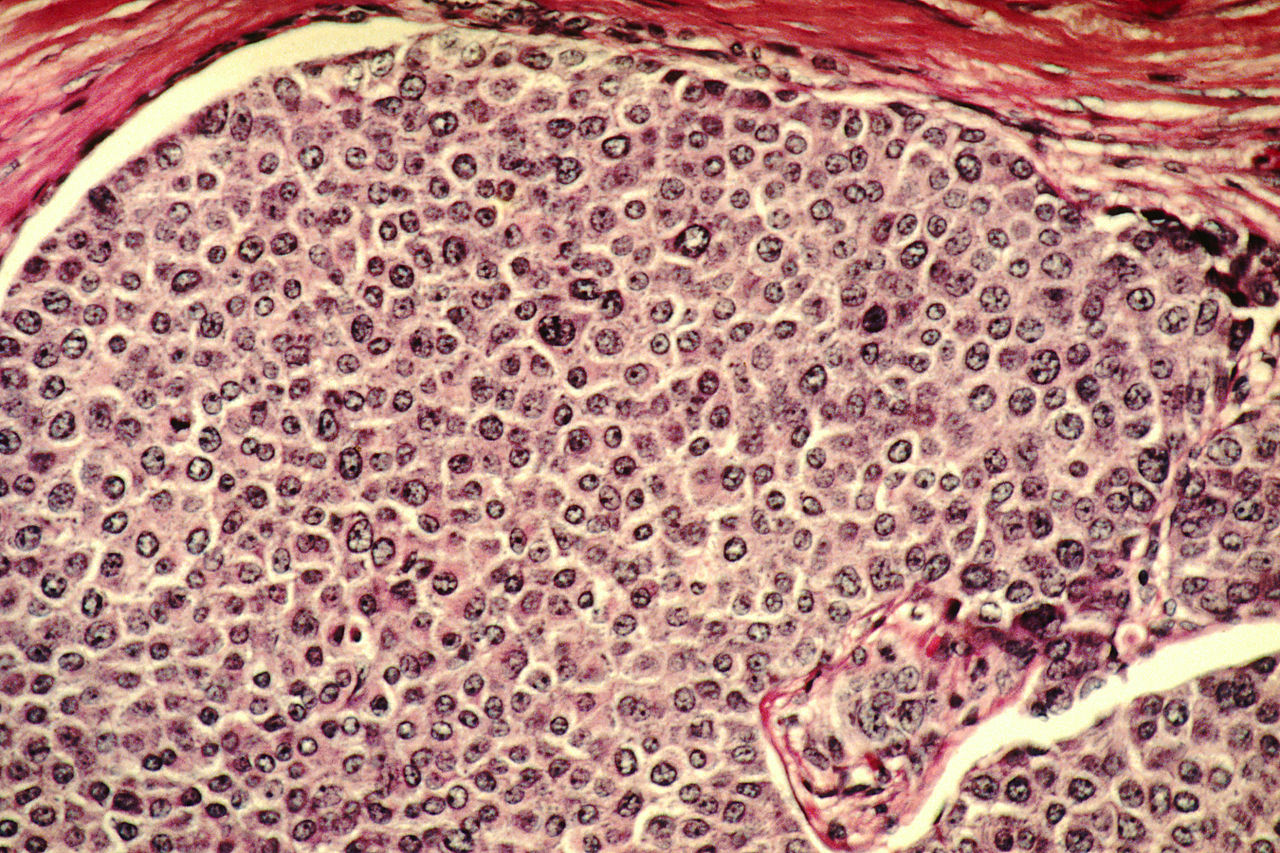
Previous studies have examined cell behaviors in numerous ways. An especially common approach is the physics-based model, wherein cell dynamics obey the physics of transport problems. Such models utilize an assortment of different equation types — such as reaction-diffusion partial differential equations (PDEs), which represent cell dynamics as a density evolution. Although physics models are certainly powerful, they are inherently more descriptive in nature; as such, practitioners cannot interpret the rationale of cell behaviors from the PDEs.
Because Huan is interested in the “why” of these behaviors, he turned to the concept of reinforcement learning. This machine learning technique generates an agent-based model wherein each cell is an agent that makes a series of decisions to ultimately find an optimal strategy/policy towards the best possible outcome. A Markov decision process (MDP)—which accounts for state space, action space, initial state probability, transition probability, and reward function—describes these decisions. “The optimal policy maximizes the expected discounted cumulative reward,” Huan said. “This reward encapsulates the motivation that drives the agent’s behavior.”
During forward reinforcement learning, agents follow the optimal policy and generate corresponding trajectories that lead to it — but IRL is a bit more complex. In an IRL framework, one must use the agent trajectories and transition function (which are already known) to infer the reward and its corresponding optimal policy (see Figure 2). Huan assumed that the agent follows a single time-invariant policy that is optimal with regard to an unknown reward function and constrained by a transition function. “The nature of the problem is very difficult,” he said. “It’s kind of an ill-conditioned problem, so it tends to be very data hungry. We need a lot of trajectories that can hopefully reach out to all of the different possible states and actions in order to invert the reward.” This reward reveals important information about the motivations of cell behavior.
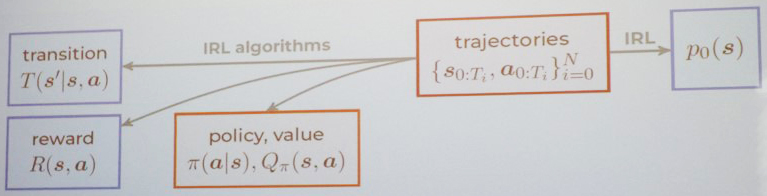
Next, Huan related the MDP to a multivariate FPE: a PDE that describes the time evolution of the probability density function. MDPs begin with one state, follow a policy, and then transition (via a random walk) to a new state as necessary. If the given policy is fixed, the state and policy evolve together over time as a Markov process (MP). And when it seems like the MP follows the dynamics of the FPE, practitioners can look for correspondents between the two.
Because the reward function is lost when the MDP reduces to an MP, Huan expanded the MP back to an MDP. His actions were guided by the following conjecture: There exists a structural isomorphism between the FPE and MDP; specifically, the potential function in the FPE is equivalent to the negative state-action value function in MDP. To expand the MP, Huan decoupled the agent’s policy from the FPE, used the inverse Bellman equation to determine the reward function, and ultimately arrived at and extracted the reward. He employed variational system identification to infer the potential function but noted that other parameter estimation/inference techniques are also suitable.
After presenting some preliminary results that investigated the relationship between velocity and signaling processes on an aggressively motile breast cancer cell line, Huan explained that his IRL framework is very much a work in progress. While his method is more computationally efficient than other IRL algorithms, it assumes just one agent and is only applicable to problems that have corresponding FPEs. In the future, Huan plans to study multi-agent systems and explore a greater variety of problems in agent-based biology.
About the Author
Lina Sorg
Managing editor, SIAM News
Lina Sorg is the managing editor of SIAM News.
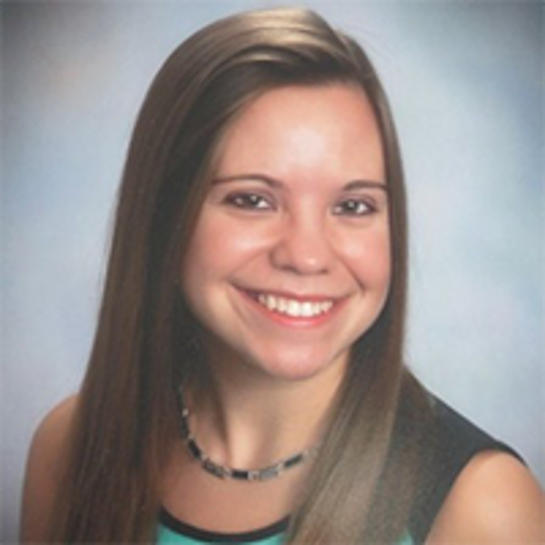
Related Reading
Stay Up-to-Date with Email Alerts
Sign up for our monthly newsletter and emails about other topics of your choosing.