Optimizing Neoantigen Cancer Vaccine Doses
According to the World Health Organization, cancer is the second leading cause of death worldwide. Different treatments options are available—including chemotherapy, surgery, and immunotherapy—depending on the type and stage of cancer. However, the immunosuppressive environment that is associated with many treatments typically impacts patients’ long-term survival.
![<strong>Figure 1.</strong> Selection of the predicted optimal peptide dose that has greater clinical benefit in overall tumor reduction than the tested vaccine dose. The blue and red curves correspond to \(J\) and \(J_T\) scores as a function of the predicted optimal doses \(\{Dose^{*,i}_p\}\). Horizontal lines indicate the \(J\) and \(J_T\) scores of the tested dose \(\hat{Dose}_p\). The red region includes suboptimal doses, while the blue region contains doses that have potential clinical benefits. Doses below the blue dashed line are optimal with uncertain clinical benefit, doses between dashed lines are optimal with lower clinical benefit, and doses in the green region are optimal with a higher benefit in tumor reduction. Figure courtesy of [10].](/media/ceuevgmd/figure1.jpg)
Given the rapid development of next-generation sequencing technologies, neoantigen cancer vaccines have emerged as personalized immunotherapies with the potential to offer additional clinical benefits to cancer patients by eliciting an antitumor immune response that can increase survival rates and long-term remission [2, 3, 7]. Unfortunately, clinical trials have struggled to find the optimal dose and regimen for personalized cancer vaccines; contributing factors include a lack of systematic approaches to test different platforms to induce immune response, small patient sample sizes that hinder the efficient characterization of immune response curves, and tumor heterogeneity [1, 7, 9]. Moreover, traditional methods of drug assessment during a phase I clinical trial in oncology—such as accelerated titration designs, canonical 3+3 designs, or similar derived designs—often provide inadequate estimates for the development of personalized drugs [5, 8]. In light of these obstacles, mechanistic mathematical modeling approaches—usually in the form of ordinary or partial differential equations—can serve as useful tools for understanding complex interactions that involve the immune system, tumors, cancer vaccines, and each patient’s health history and response to treatment.
We propose a novel approach that quantitatively determines the optimal dose composition of a personalized cancer vaccine, including peptides and adjuvants [10]. Using optimal control theory, we pose two optimization problems that are subject to a fixed vaccination schedule and an existing mechanistic immunological model [6] that describes key events associated with an immune response to a neoantigen cancer vaccine at the cellular and subcellular level. The first optimization problem \((J_1)\) focuses on efficacy by minimizing the overall number of tumor cells \((J_T)\) and total vaccine exposure \((J_V)\) throughout the course of treatment. The second optimization problem \((J_2)\) targets both efficacy and safety by attempting to identify the minimum required number of activated \(CD4^+\) and \(CD8^+\) cells \((J_{\textrm{T-cell}})\) to achieve the largest reduction in tumor cell count. We apply both optimization problems to six patients with advanced melanoma to investigate possible benefits of optimal personalized vaccine doses [4].
![<strong>Figure 2.</strong> Optimal peptide concentrations by patient. Bar plots correspond to the optimal peptide doses in terms of the number of folds of the clinical trial dose for each vaccination via \(J_1\) (blue) and \(J_2\) (green). The horizontal dashed line represents the baseline clinical trial dose for each patient. Figure courtesy of [10].](/media/5jcjdje4/figure2.jpg)
Our optimization approach requires identifying the peptide dose with the greatest clinical benefit in overall tumor reduction while also ensuring that the total vaccine dose does not significantly exceed other doses, such as those used in clinical trials. The approach first supplies a set of optimal peptide doses that allow us to approximate a global minimizer for the objective functional (either \(J_1\) or \(J_2\)). However, we noticed that this global minimizer may not provide the highest overall tumor reduction (i.e., \(J_T\) score) when compared to another vaccine dose (see Figure 1). To overcome this challenge, we selected an optimal vaccine dose from our set of optimal peptide doses that have the highest tumor reduction when compared to an alternative dose (i.e., the lowest \(J_T\) in the green region of Figure 1). We describe the selection process for this optimal dose in more detail in the literature [10].
Our optimization approach obtained the optimal peptide doses for every patient in Figure 2 in terms of the number of folds of the clinical trial dose for each vaccination [10]. All patients experience tumor size reductions that are either similar to or greater than those in the clinical trial [4]; the optimal dose for \(J_1\) (efficacy) is consistent with model predictions [6]. In the case of optimal doses for \(J_2\) (efficacy and safety), our optimal peptide dose for patients 1, 3, 4, and 5 match their clinical trial doses almost exactly. Patients 2 and 6 require higher doses for the initial vaccinations but lower subsequent doses.
![<strong>Figure 3.</strong> Tumor diameter by patient when optimizing \(J_1\) and \(J_2\). The blue and green curves represent optimal vaccinations; the dotted, solid, and dashed orange curves represent suboptimal vaccinations with 3, 1, and 0.1 folds of clinical trial dose, respectively; and the red curve represents the absence of vaccination. The numbers within each diagram represent the tumor diameter at the end of treatment (from \(J_1\)). Figure courtesy of [10].](/media/s0lgmy4v/figure3.jpg)
![<strong>Figure 4.</strong> Optimal vaccine dose performance in terms of overall and individual ratios for \(J_1\) (efficacy), as well as total vaccine dose ratio compared to clinical trial dose. Green cells indicate higher clinical benefit or a lower total vaccine dose, while red cells indicate lower clinical benefit or a higher total vaccine dose. Figure courtesy of [10].](/media/bwbne5qo/figure4.jpg)
Figure 3 depicts the tumor responses to the optimal doses and compares them with deviations from the clinical doses. The optimal dosing performed slightly better than the clinical trial dose during the vaccination period (0-139 days), but most of the tested vaccine doses showed similar effectiveness in tumor reduction during the late stage (after day 139). Figure 4 quantifies the clinical benefit in overall tumor reduction between the optimal vaccine dose and the clinical trial dose. The combination of \(J_T\)-ratio and \(D\)-ratio indicates that the optimal dose for \(J_1\) could offer higher overall clinical benefits to some of these patients, but at the price of almost doubling the clinical trial dose.
It is important to note that our optimization approach may not always produce a dose that yields significantly better clinical outcomes in overall tumor reduction compared to the tested doses, as was the case with the optimal doses for \(J_2\). In some cases, the optimal dose may provide clinical outcomes that are similar to those of other tested doses. Nevertheless, we believe that our work can serve the clinical community’s investigation of various dosing regimens in personalized immunotherapy. It also highlights the importance of alternative dosages in achieving the primary objective of immunotherapy: triggering a potent immune response to reduce or eliminate tumors.
Wencel Valega-Mackenzie delivered a minisymposium presentation on this research at the 2023 SIAM Conference on Control and Its Applications, which took place in Philadelphia, Pa., last year.
Disclaimer: My comments represent my own best judgement and in no way bind or obligate the U.S. Food and Drug Administration.
References
[1] Liu, J., Fu, M., Wang, M., Wan, D., Wei, Y., & Wei, X. (2022). Cancer vaccines as promising immuno-therapeutics: Platforms and current progress. J. Hematol. Oncol., 15(1), 28.
[2] Melero, I., Gaudernack, G., Gerritsen, W., Huber, C., Parmiani, G., Scholl, S., … Mellstedt, H. (2014). Therapeutic vaccines for cancer: An overview of clinical trials. Nat. Rev. Clin. Oncol., 11(9), 509-524.
[3] Mellman, I., Coukos, G., & Dranoff, G. (2011). Cancer immunotherapy comes of age. Nature, 480(7378), 480-489.
[4] Ott, P.A., Hu, Z., Keskin, D.B., Shukla, S.A., Sun, J., Bozym, D.J., … Wu, C.J. (2017). An immunogenic personal neoantigen vaccine for patients with melanoma. Nature, 547(7662), 217-221.
[5] Peck, R.W. (2018). Precision medicine is not just genomics: The right dose for every patient. Annu. Rev. Pharmacol. Toxicol., 58, 105-122.
[6] Rodriguez Messan, M., Yogurtcu, O.N., McGill, J.R., Nukala, U., Sauna, Z.E., & Yang, H. (2021). Mathematical model of a personalized neoantigen cancer vaccine and the human immune system. PLoS Comput. Biol., 17(9), e1009318.
[7] Shemesh, C.S., Hsu, J.C., Hosseini, I., Shen, B.-Q., Rotte, A., Twomey, P., … Wu, B. (2021). Personalized cancer vaccines: Clinical landscape, challenges, and opportunities. Mol. Ther., 29(2), 555-570.
[8] Shord, S.S., Zhu, H., Liu, J., Rahman, A., Booth, B., & Zineh, I. (2023). US Food and Drug Administration embraces using innovation to identify optimized dosages for patients with cancer. CPT Pharmacometrics Syst. Pharmacol., 12(11), 1573-1576.
[9] Taylor, D., Meyer, C.T., Graves, D., Sen, R., Fu, J., Tran, E., … Korrer, M.J. (2022). MuSyC dosing of adjuvanted cancer vaccines optimizes antitumor responses. Front. Immunol., 13, 936129.
[10] Valega-Mackenzie, W., Rodriguez Messan, M., Yogurtcu, O.N., Nukala, U., Sauna, Z.E., & Yang, H. (2024). Dose optimization of an adjuvanted peptide-based personalized neoantigen melanoma vaccine. PLOS Comput. Biol., 20(3), e1011247.
About the Author
Wencel Valega-Mackenzie
ORISE Postdoctoral Fellow, Center for Biologics Evaluation and Research
Wencel Valega-Mackenzie is an ORISE Postdoctoral Fellow in the Division of Analytics and Benefit-Risk Assessment within the Office of Biostatistics and Pharmacovigilance in the Center for Biologics Evaluation and Research. His primary work uses mathematical and computational techniques to develop mechanistic models to better understand immune responses to therapeutic products. Valega-Mackenzie has previously developed mechanistic models in epidemiology and ecology fields to understand resource allocation and the dynamics of Zika virus.
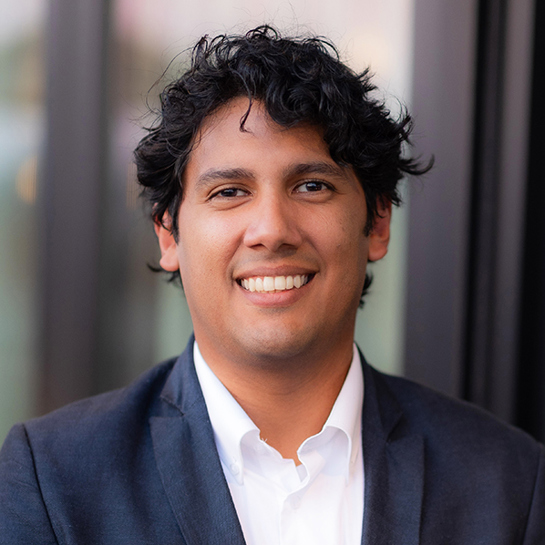
Stay Up-to-Date with Email Alerts
Sign up for our monthly newsletter and emails about other topics of your choosing.