Persistent Homology Analysis of Biomolecular Data
Technological advances in the past few decades have fueled the exponential growth of “omic” data in biology. Understanding the rules of life from existing omic data sets, which offer unprecedented opportunities for mathematicians, remains an important mission of the field. Biomolecular structure-function relationship is a major rule of life, and recognizing this relationship is the holy grail of biophysics and a central issue in experimental biology.
Geometric modeling is vital to the comprehension of biomolecular structure-function relationships. It also bridges the gap between biological data and theoretical models, such as quantum mechanics, molecular mechanics, statistical mechanics, thermodynamics, and multiscale models. However, geometry-based models are frequently inundated with too much structural detail and thus often computationally intractable. Topology provides the ultimate abstraction of geometric complexity by concerning only the connectivity of different components in a space, and characterizing independent entities, rings, and higher-dimensional faces of the space in terms of topological invariants or Betti numbers. To study topological invariants in a discrete data set—like atoms in a biomolecule—algebraic topology utilizes simplicial complexes under various settings, such as the Vietoris-Rips complex, Čech complex, or alpha complex. Specifically, a 0-simplex is a vertex, a 1-simplex an edge, a 2-simplex a triangle, and a 3-simplex a tetrahedron, as illustrated in Figure 1. Algebraic groups built on these simplicial complexes are used in simplicial homology to systematically compute Betti numbers for a given data set [7].
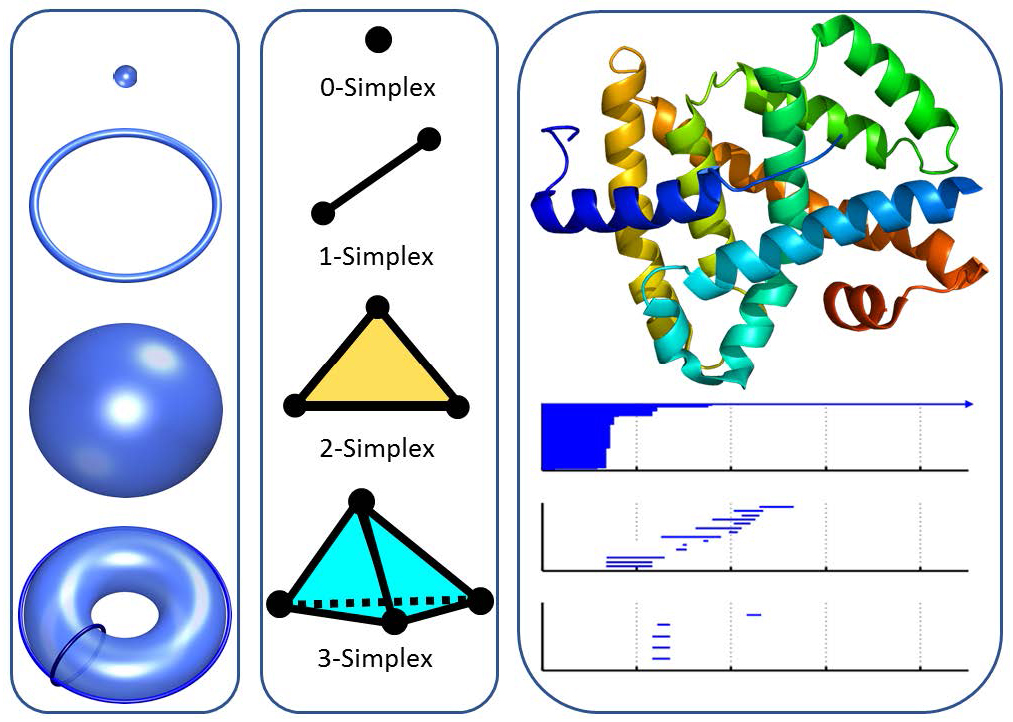
Nevertheless, traditional topology and homology are truly free of metrics or coordinates and thus keep too little geometric information to be practically useful for biomolecules. Persistent homology, a new branch of algebraic topology, embeds multiscale geometric information into topological invariants to achieve an interplay between geometry and topology [14]. It creates a variety of topological spaces of a given object by varying a filtration parameter, such as the radius of balls or the level set of a real-valued function. As a result, persistent homology can capture topological features continuously over a range of spatial scales, and the resulting analysis is often visualized by barcodes [6] or persistence diagrams [5]. As such, the changes of topological invariants over scales are recorded by the “birth,” “death,” and “persistence” of barcodes over filtration. Persistent homology has been applied to a variety of domains, including image/signal analysis, chaotic dynamics, sensor networks, complex networks, shape recognition, and computational biology [13].
For nano- and biomolecules, persistent homology enables a quantitative topological analysis—which reveals biomolecular “topology-function relationships”—via topological fingerprints (TFs) [9, 11]. Contrary to popular belief, short-lived topological events are not noise, but rather part of TFs; they play a valuable role in the quantitative topological analysis of protein folding stability [9] and fullerene curvature energy [8]. Differential geometry has been utilized to derive partial differential equation-based persistence for biomolecules [8]. Multidimensional persistence induced by a multiresolution analysis [12] is particularly useful for resolving ill-posed inverse problems in cryo-electron microscopy structure determination [10].
TFs provide biomolecules with a systematic and unique representation that cannot be literally cast into traditional physical interpretation. Fortunately, this representation is ideally suited for machine learning (particularly deep learning), which captures nonlinear and high-order interactions among features in sufficiently large and intrinsically complex data sets. One of the first integrations of machine learning and TFs offered an encouraging classification of tens of thousands of proteins involving hundreds of tasks [4]. However, persistent homology neglects chemical and biological information during topological simplification and is thus not as competitive as geometry or physics-based representation in quantitative predictions. Element-specific persistent homology, or multicomponent persistent homology built on colored biomolecular networks, has been introduced to retain chemical and biological information during topological abstraction [2]. This approach enciphers biological properties—such as hydrogen bonds, van der Waals interactions, hydrophilicity, and hydrophobicity—into topological invariants, rendering a potentially revolutionary representation for biomolecules [1, 3].
Rational drug design is an imperative life science problem that ultimately tests our understanding of biological systems. Designing efficient drugs to cure disease is one of the most challenging tasks in the biological sciences. Multicomponent persistent homology plays a crucial role in hot-spot prediction, drug-binding pose analysis, binding affinity prediction, structure optimization, toxicity analysis, and pharmacokinetic simulation. For example, the integration of machine learning with multiscale weighted colored graphs and multicomponent persistent homology provided the best free energy ranking for Set 1 (Stage 2) in D3R Grand Challenge 2, a worldwide competition in computer-aided drug design.
References
[1] Cang, Z.X., & Wei, G.W. (2017). Analysis and prediction of protein folding energy changes upon mutation by element specific persistent homology. Bioinform., doi: 10.1093/bioinformatics/btx460.
[2] Cang, Z.X., & Wei, G.W. (2017). Integration of element specific persistent homology and machine learning for protein-ligand binding affinity prediction. Inter. J. Numer. Meth. Biomed. Eng., doi:10.1002/cnm.2914.
[3] Cang, Z.X., & Wei, G.W. (2017). TopologyNet: Topology based deep convolutional and multi-task neural networks for biomolecular property predictions. Plos Comp. Bio., 13(7), e1005690.
[4] Cang, Z.X., Mu, L., Wu, K., Opron, K., Xia, K., & Wei, G.W. (2015). A topological approach to protein classification. Mol. Based Math. Bio., 3, 140-162.
[5] Edelsbrunner, H., & Harer, J. (2008). Persistent homology — a survey. Cont. Math., 453, 257-282.
[6] Ghrist, R. (2008). Barcodes: The persistent topology of data. Bull. Am. Math. Soc., 45, 61-75.
[7] Kaczynski, T., Mischaikow, K., & Mrozek, M. (2004). Computational Homology. In Applied Mathematical Sciences (Vol. 157). New York, NY: Springer-Verlag.
[8] Wang, B., & Wei, G.W. (2016). Object-oriented persistent homology. J. Comp. Phys. 305, 276-299.
[9] Xia, K.L., & Wei, G.W. (2014). Persistent homology analysis of protein structure, flexibility and folding. Inter. J. Num. Meth. Biomed. Eng., 30, 814-844.
[10] Xia, K.L., & Wei, G.W. (2015). Persistent topology for cryo-EM data analysis. Inter. J. Num. Meth. Biomed. Eng., 31, e02719.
[11] Xia, K.L., Feng, X., Tong, Y.Y., & Wei, G.W. (2015). Persistent homology for the quantitative prediction of fullerene stability. J. Comp. Chem., 36, 408-422.
[12] Xia, K.L., Zhao, Z.X., & Wei, G.W. (2015). Multiresolution topological simplification. J. Comp. Bio., 22, 1-5.
[13] Yao, Y., Sun, J., Huang, X.H., Bowman, G.R., Singh, G., Lesnick, M.,…Carlsson, G. (2009). Topological methods for exploring low-density states in biomolecular folding pathways. J. Chem. Phys., 130, 144115.
[14] Zomorodian, A., & Carlsson, G. (2005). Computing persistent homology. Dis. Comp. Geo., 33, 249-274.
About the Author
Guo-Wei Wei
MSU Foundation Professor, Michigan State University
Guo-Wei Wei is an MSU Foundation Professor at Michigan State University. His research explores the mathematical foundations of bioscience and data science.
Stay Up-to-Date with Email Alerts
Sign up for our monthly newsletter and emails about other topics of your choosing.