Political Centrism and Extremism: A Mathematical Analysis
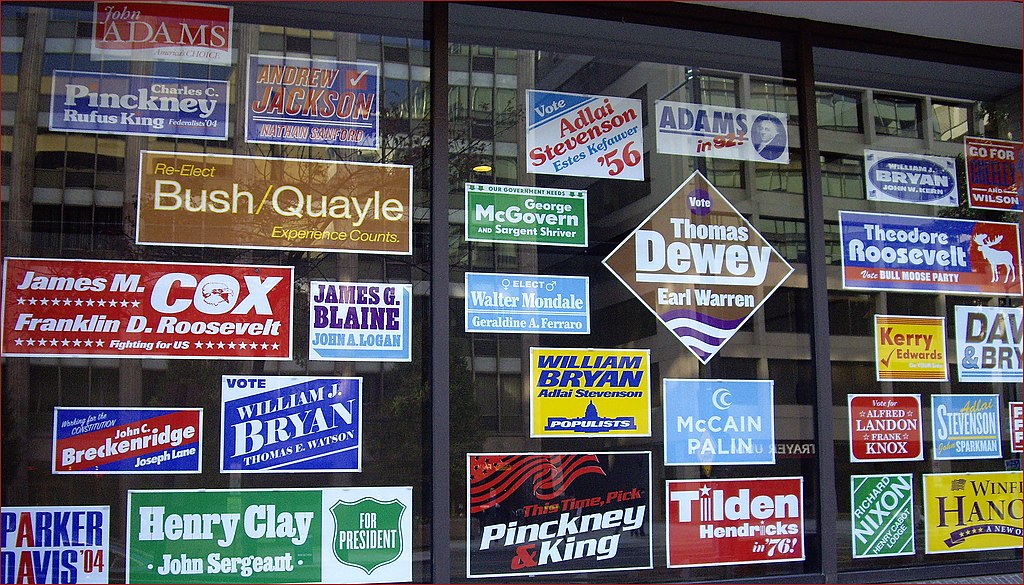
Politicians adopt various strategies to appeal to voters and win elections (see Figure 1). In a two-party system, these strategies are often driven by extensive polling and careful positioning of one candidate relative to their opponent. Nevertheless, the strategy that helps a candidate win one election might cause them to lose another. Our recent work explores a simplified mathematical model of this political process and uncovers unexpected phenomena and mechanisms that we then compare to real-life political strategies and outcomes [2, 3].
In the U.S., some politicians (e.g., Bill Clinton) have won elections by moving to the political center, while others (e.g., Donald Trump) have succeeded by securing the vote among their bases. We refer to these two strategies as centrism and extremism, but also recognize that not all off-center positions can reasonably be considered “extreme.”
Some voters always vote for the candidate whose views most closely match their own — regardless of how close they actually are. They vote for the least undesirable candidate even if all contenders are undesirable to them; we call these voters loyal. Others will abstain if no candidate closely represents their views; we call these voters disloyal (without intending any moral judgment).
The median voter theorem confirms the reasonable guess that centrism is an effective tactic when most voters are loyal [1]. We would also expect extremism to be a good strategy for a candidate with a strong off-center base of disloyal voters. Is there anything remarkable about the optimal strategy’s transition from centrism to extremism given a change in parameters, such as voter loyalty? Can we view this transition as a bifurcation in a dynamical system? If so, what kind of bifurcation and what does it say about politics more generally? Finally, are the answers to such questions reflected in real-life politics?
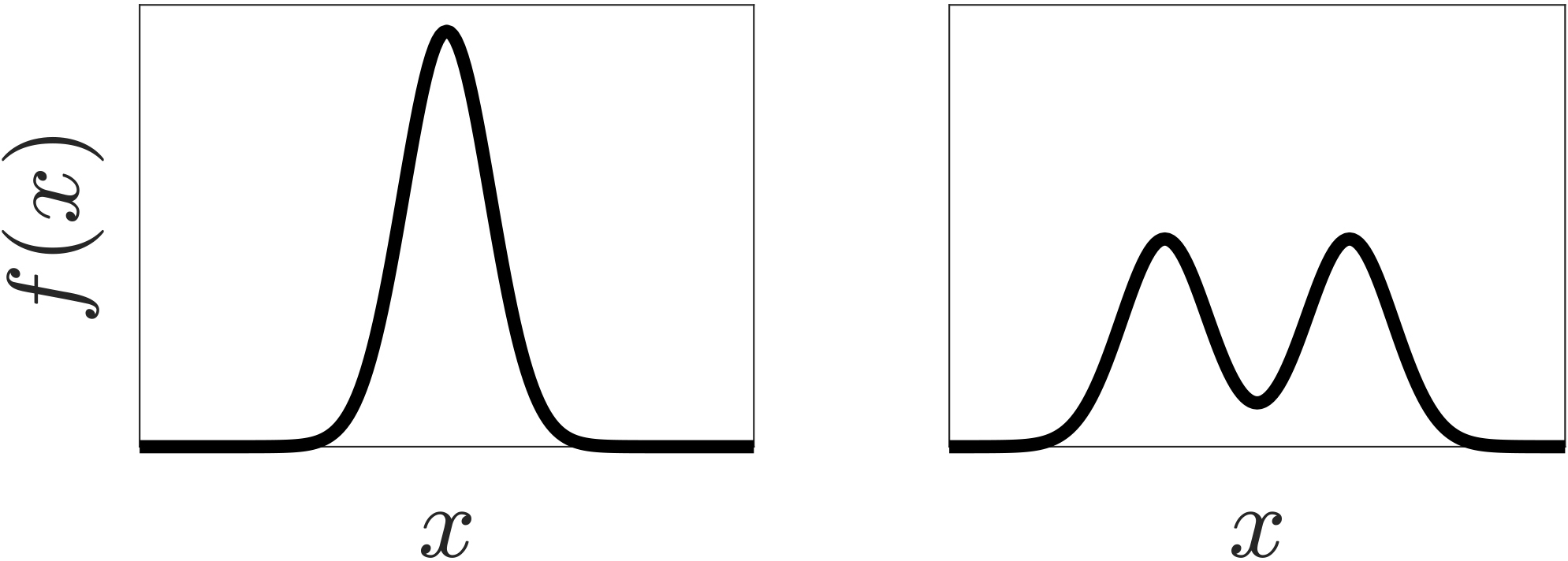
To create a simple setting, we pretend that any voter’s or candidate’s political positions can be reduced to a single number \(x\), where negative \(x\) corresponds to “left-wing” views and positive \(x\) corresponds to “right-wing” views. We further envision a continuum of voters with views that can be described by a probability density function \(f\) (see Figure 2). Without loss of generality, we assume that the median of \(f\) is \(0\).
Now consider two competing candidates \(L\) and \(R\) whose views lie at positions \(\ell\) and \(r\), with \(\ell < r\). To start, we assume that voter loyalty is maximal — i.e., everyone votes for the candidate who is closest to their own position. The vote shares of \(L\) and \(R\) will thus be
\[S_L = \int_{-\infty}^{(\ell+r)/2} f(x) ~\! dx ~~~~\mbox{and} ~~~~ S_R = \int_{(\ell+r)/2}^\infty f(x) ~\! dx.\]
Candidate \(L\) wins if \(S_L> \frac{1}{2}\) and candidate \(R\) wins if \(S_R > \frac{1}{2}\). Since the median of \(f\) is \(0\), we can equivalently say that \(L\) wins if \(\frac{\ell+r}{2} > 0\) and \(R\) wins if \(\frac{\ell+r}{2} < 0\). This result means that the candidate who is closest to the median will win, in agreement with the median voter theorem [1].
To capture voter loyalty, we now assume that only a fraction \(g(z)\) of voters still vote if the candidate closest to them is at distance \(z\), where \(g(z)\) is a decreasing function of \(z \geq 0\) with \(g(0)=1\). As an example, consider \(g(z) = e^{- \frac{z}{\gamma}}\) with \(\gamma > 0\). Larger values of \(\gamma\) correspond to greater voter loyalty. The two candidates’ vote shares become
\[S_L = \int_{-\infty}^{(\ell+r)/2} f(x) e^{-\frac{|\ell-x|}{\gamma}} ~\! dx ~~~~\mbox{and} ~~~~ S_R = \int_{(\ell+r)/2}^\infty f(x)e^{-\frac{|r-x|}{\gamma}} ~\! dx.\]
We assume that the candidates will adjust their positions with time, so \(\ell=\ell(t)\) and \(r=r(t)\), governed by equations of the form
\[\frac{d \ell}{dt} = \alpha \frac{\partial S_L}{\partial \ell}, ~~~~ \frac{d r}{dt} = \beta \frac{\partial S_R}{\partial r}.\tag1\]
Here, \(\alpha\) and \(\beta\) are positive constants that measure the eagerness with which \(L\) and \(R\) adjust their positions to gain votes. We next study the changes in this system’s solutions as \(\gamma\) varies [2]. Under some circumstances—with a polarized electorate and a significant difference between \(\alpha\) and \(\beta\)—the transition from centrism to extremism involves a saddle-node bifurcation, and optimal candidate positions can jump discontinuously as a result. In Figure 3, we assume that \(\beta=0\) and \(\alpha=1\); \(r\) thus remains fixed while \(\ell\) moves, governed by \(\frac{d \ell}{dt} = \frac{\partial S_L}{\partial \ell}\). For \(\gamma=2\), there is a stable fixed point near \(-1\). As \(\gamma\) rises to \(4\), the fixed point moves closer to \(−0.5\). When \(\gamma\) rises even further, the fixed point collides with an unstable fixed point, and both are annihilated. From that moment on, it is in \(L\)'s best interest to move towards \(R\)'s position at \(r=1\). \(L\)'s optimal strategy hence changes abruptly and discontinuously as \(\gamma\) rises above a threshold value that is slightly higher than \(4\).
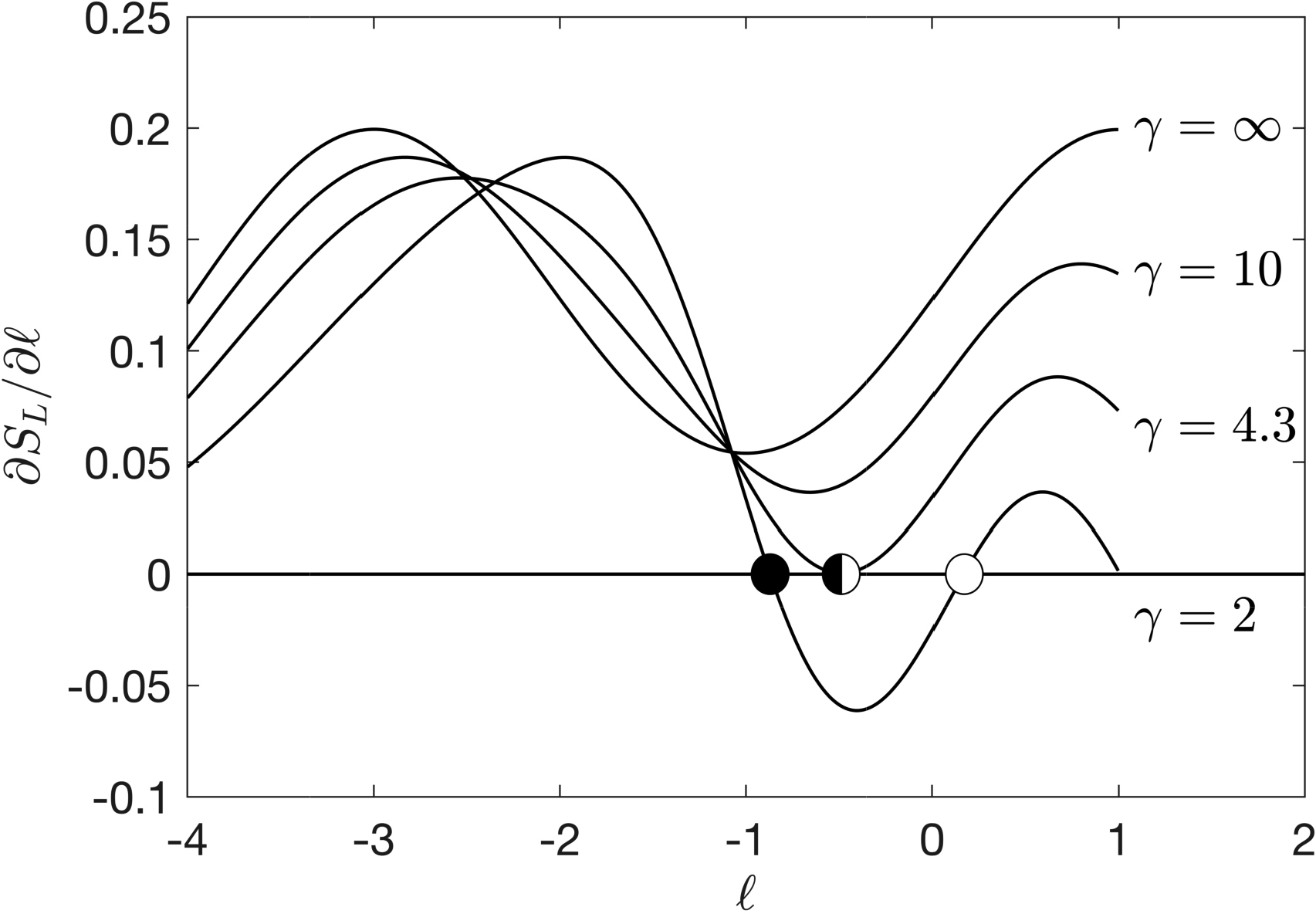
Do these types of abrupt shifts occur in real life? In 2016, Donald Trump won the U.S. presidential election by not moving towards the center and instead securing the votes of his right-of-center base. But he lost the subsequent 2020 election, perhaps because \(\gamma\) had increased; emotions were running high and many people were determined to vote. Perhaps Trump would have won if he had moved towards the center. And perhaps the abruptness of the transition caught many people by surprise, making them susceptible to claims that the election was conducted improperly.
Our model is a suitable example for an undergraduate course on ordinary differential equations [2]. We also wrote a paper that is specifically aimed at undergraduate students and their instructors [3], and created a web-based app that explores our model’s parameters.
Natasa Dragovic delivered a contributed presentation on this research at the 2023 SIAM Conference on Applications of Dynamical Systems, which took place in Portland, Ore., last year.
References
[1] Black, D. (1948). On the rationale of group decision-making. J. Polit. Econ., 56(1), 23-34.
[2] Börgers, C., Boghosian, B., Dragovic, N., & Haensch, A. (2023). A blue sky bifurcation in the dynamics of political candidates. Amer. Math. Monthly.
[3] Börgers, C., Dragovic, N., Haensch, A., Kirshtein, A., & Orr, L. (2024). ODEs and mandatory voting. CODEE J., 17, 11.
About the Authors
Christoph Börgers
Professor, Tufts University
Christoph Börgers is a professor of mathematics at Tufts University. He holds a Ph.D. from the Courant Institute of Mathematical Sciences at New York University. His current research focus is on the probabilistic analysis of interacting particles and agents.
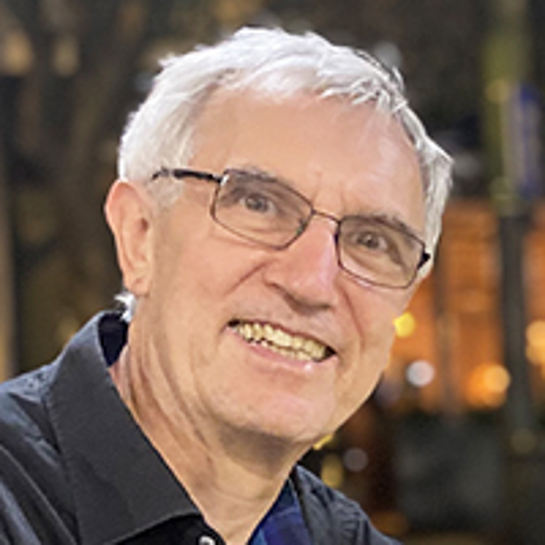
Natasa Dragovic
Assistant professor, University of St. Thomas
Natasa Dragovic is an assistant professor in the Department of Mathematics at the University of St. Thomas. She holds a Ph.D. in mathematics from the University of Texas at Austin. Dragovic’s research lies at the intersection of probability, dynamical systems, and social science, with a focus on mathematical modeling that examine changes in opinions over time.
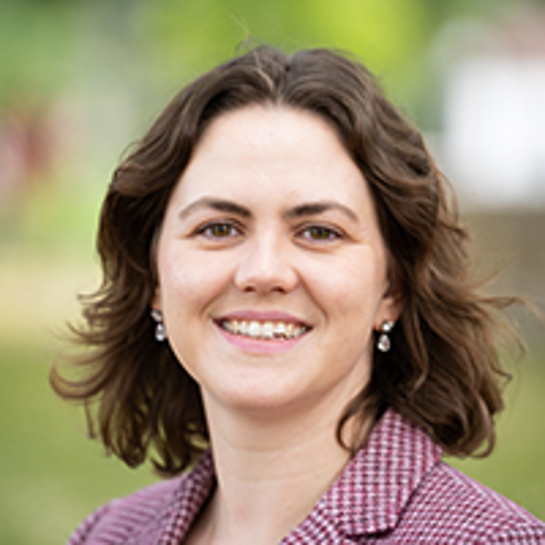
Anna Haensch
Senior Data Scientist, Data Intensive Studies Center
Anna Haensch is a senior data scientist in the Data Intensive Studies Center at Tufts University with a secondary appointment in the Department of Mathematics. She holds a Ph.D. in mathematics from Wesleyan University. Haensch’s research lies at the intersection of mathematics and the social sciences; she explores the many ways through which data can contribute to a safer, more sustainable, and more equitable world.
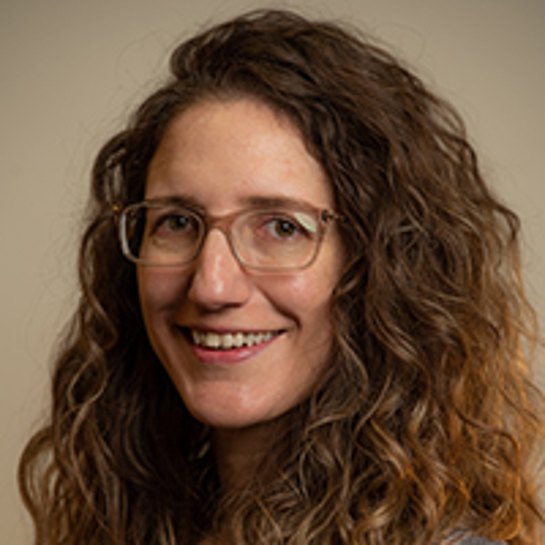
Stay Up-to-Date with Email Alerts
Sign up for our monthly newsletter and emails about other topics of your choosing.