Predicting Mood Episodes Based on Sleep and Circadian Rhythm Features
Individuals with mood disorders experience periods of pronounced symptoms called mood episodes. These episodes are closely related to sleep-wake patterns [3]. For example, sleep disturbances like insomnia or hypersomnia often accompany depressive episodes [7], while a reduced need for sleep is a predominant feature of manic episodes [1]. Given this connection, numerous studies have attempted to use sleep data to predict mood episodes [4, 9]. However, existing methods often require both sleep patterns and additional data, such as step counts, heart rate, phone usage, and GPS-based mobility. These stipulations increase data collection costs and inhibit routine use, thus reducing the practicality of current prediction models.
We overcame these limitations to predict mood episodes with only sleep-wake pattern data (see Figure 1a). Specifically, we derived 32 indices that pertain to sleep patterns—including sleep efficiency and sleep and wake duration during the sleep period—to effectively quantify the various sleep structures (see Figure 1b). In addition, we employed indices of circadian rhythm: the internal 24-hour biological clock that regulates activities like sleep and wakefulness.
![<strong>Figure 1.</strong> Framework to predict mood episodes from sleep pattern information using a machine learning (ML) classification algorithm. <strong>1a.</strong> 168 mood disorder patients provided sleep-wake pattern data from wearable devices. <strong>1b.</strong> We processed the sleep-wake pattern data to derive indices that capture quantity-related features of the patterns and estimated circadian rhythm. <strong>1c.</strong> By inputting these indices into the ML classification algorithm, we were able to predict daily depressive and manic episodes. Figure courtesy of [5].](/media/baqhsilb/figure1.jpg)
It has historically been challenging to measure circadian rhythm [6]. Researchers have developed various methods in response, including a mathematical-modeling-based approach that can accurately estimate circadian rhythm [8]. This model simulates the human circadian pacemaker by incorporating sleep information in the form of a light profile [2]. We applied the model to sleep-wake binary time series to estimate the circadian rhythm, thereby deriving four additional indices related to circadian rhythm — ultimately leading to a total of 36 sleep and circadian rhythm features (see Figure 1b).
![<strong>Figure 2.</strong> Our models can accurately predict mood episodes in patients with mood disorders. Here, we show receiver operating characteristic curves of the extreme gradient boosting models in the prediction of depressive episodes <strong>(2a)</strong>, manic episodes <strong>(2b)</strong>, and hypomanic episodes <strong>(2c)</strong>. The trained models predicted the depressive, manic, and hypomanic episodes with area under the curve (AUC) values of 0.80, 0.98, and 0.95, respectively. Figure courtesy of [5].](/media/dswdnlyk/figure2.jpg)
Next, we applied these 36 features to an extreme gradient boosting algorithm to predict depressive, manic, and hypomanic episodes (see Figure 1c). This approach enabled us to analyze data from 168 mood disorder patients and accurately forecast the next day’s depressive, manic, and hypomanic episodes via present-day sleep-wake pattern information, with respective area under the curve values of 0.80, 0.98, and 0.95 (see Figure 2).
![<strong>Figure 3.</strong> Shapley additive explanation (SHAP) values of the circadian phase. Each dot represents a daily sample, with red and blue colors respectively indicating high and low values of the circadian phase (i.e., delayed and advanced circadian phase estimates). A higher SHAP value indicates a greater probability of mood episode occurrence. Patients face a higher risk of experiencing depressive episodes <strong>(3a)</strong> when their estimated circadian phase is delayed more than usual. Conversely, patients tend to experience manic episodes <strong>(3b)</strong> when they exhibit circadian phase estimates that are more advanced than their own standards. Figure adapted from [5].](/media/liybv3ur/figure3.jpg)
During this process, we identified daily variations in circadian rhythm as a critical predictor of mood episodes. A delayed circadian rhythm increases the risk of depressive episodes, whereas an excessively advanced rhythm heightens the likelihood of manic episodes (see Figure 3). An individual who usually goes to sleep at 11 p.m. and wakes up at 7 a.m. would thus face an increased risk of depression if their sleep schedule shifted to later times, say, going to sleep at 11:30 p.m. and waking up at 9 a.m.
We hope that this study will enhance treatment efficiency for patients with mood disorders. In clinical settings, more effective treatments require objective mood episode data to supplement subjective psychological assessments. Our study provides practitioners with a means of obtaining objective mood episode indicators (i.e., the probability of mood episodes) using only sleep-wake pattern data [5]. Furthermore, the noninvasive, passive nature of data collection through wearable devices makes this approach particularly advantageous for daily use, enabling the seamless integration of mood episode tracking in everyday life.
Acknowledgments: We would like to acknowledge the coauthors of this study: Jaegwon Jeong, Chul-Hyun Cho, Ji Won Yeom, and Heon-Jeong Lee of Korea University; Yun Min Song of the Korea Advanced Institute of Science and Technology; and Taek Lee and Jung-Been Lee of Sun Moon University.
References
[1] Barbini, B., Bertelli, S., Colombo, C., & Smeraldi, E. (1996). Sleep loss, a possible factor in augmenting manic episode. Psychiatry Res., 65(2), 121-125.
[2] Forger, D.B., Jewett, M.E., & Kronauer, R.E. (1999). A simpler model of the human circadian pacemaker. J. Biol. Rhythms, 14(6), 532-537.
[3] Germain, A., & Kupfer, D.J. (2008). Circadian rhythm disturbances in depression. Hum. Psychopharmacol., 23(7), 571-585.
[4] Lee, H.-J., Cho, C.-H., Lee, T., Jeong, J., Yeom, J.W., Kim, S., … Kim, L. (2023). Prediction of impending mood episode recurrence using real-time digital phenotypes in major depression and bipolar disorders in South Korea: A prospective nationwide cohort study. Psychol. Med., 53(12), 5636-5644.
[5] Lim, D., Jeong, J., Song, Y.M., Cho, C.-H., Yeom, J.W., Lee, T., … Kim, J.K. (2024). Accurately predicting mood episodes in mood disorder patients using wearable sleep and circadian rhythm features. NPJ Digit. Med., 7(1), 324.
[6] Rzepka-Migut, B., & Paprocka, J. (2020). Melatonin-measurement methods and the factors modifying the results. A systematic review of the literature. Int. J. Environ. Res. Public Health, 17(6), 1916.
[7] Soehner, A.M., Kaplan, K.A., & Harvey, A.G. (2014). Prevalence and clinical correlates of co-occurring insomnia and hypersomnia symptoms in depression. J. Affect. Disord., 167, 93-97.
[8] Stone, J.E., Postnova, S., Sletten, T.L., Rajaratnam, S.M.W., & Phillips, A.J.K. (2020). Computational approaches for individual circadian phase prediction in field settings. Curr. Opin. Syst. Biol., 22, 39-51.
[9] Sükei, E., Norbury, A., Perez-Rodriguez, M.M., Olmos, P.M., & Artés, A. (2021). Predicting emotional states using behavioral markers derived from passively sensed data: Data-driven machine learning approach. JMIR mHealth uHealth, 9(3), e24465.
About the Authors
Dongju Lim
Ph.D. candidate, Korea Advanced Institute of Science and Technology
Dongju Lim is a Ph.D. candidate in the Department of Mathematical Sciences at the Korea Advanced Institute of Science and Technology, where he focuses on the mathematical-model-based analysis and application of human sleep-wake pattern data from wearable devices.
Jae Kyoung Kim
Associate professor, Korea Advanced Institute of Science and Technology
Jae Kyoung Kim is an associate professor in the Department of Mathematical Sciences at the Korea Advanced Institute of Science and Technology and chief investigator in the Institute for Basic Science’s Biomedical Mathematics Group. He holds a Ph.D. in applied and interdisciplinary mathematics from the University of Michigan and was a postdoctoral fellow at the Mathematical Biosciences Institute of Ohio State University. Kim’s research combines nonlinear dynamics, the theory of stochastic processes, and scientific computing to address critical biological and medical problems, including sleep disorders. He has received the Human Frontier Science Program’s Young Investigator Award, the Korean Society for Industrial and Applied Mathematics’ Young Researcher Award, the Korean Mathematical Society’s Sangsan Prize for Young Mathematicians, and the Choi Seok-jung Award.
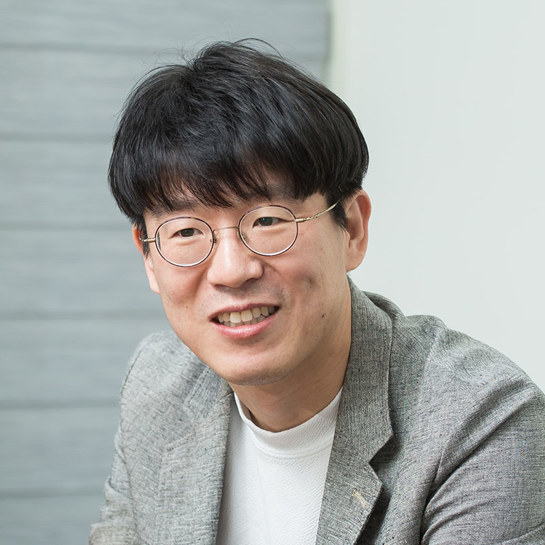