Preparing Virginia’s Students for New Post-secondary Pathways in Data Science
Inspired by a groundbreaking 2018 study entitled Catalyzing Change in High School Mathematics from the National Council of Teachers of Mathematics [1], the Commonwealth of Virginia is considering a redesign of its Mathematics Standards of Learning (SOL) to promote deeper learning. A diverse group of mathematicians, mathematics educators from higher education, and K-12 leaders across Virginia convened to share ideas that inspired critical conversations about the way in which K-12 mathematics education prepares all students for an evolving post-secondary landscape.
From 2019 to 2021, business and industry stakeholders discussed possible ways to modernize mathematics education in Virginia public schools. Conversations addressed (i) What essential content should be included in the Mathematics SOL, (ii) where content across grade levels could better focus on college and career readiness, and (iii) how the introduction of additional course options for students might create distinct mathematics pathways. A common emerging thread was the need for mathematics content that focused on data science and would better equip students for postsecondary options. The proposed Data Science SOL—meant to support a locally designed, high-school-level data science course—were presented to the Virginia Board of Education in November 2021 and are currently under review. These prospective standards mark the start of a modernization of the Virginia Mathematics SOL, which are scheduled for revision in 2023.
While higher education institutions around the world have been steadily incorporating some version of data science into a wide range of undergraduate curricula (from business to healthcare and even the social sciences), K-12 education lags behind in terms of both pedagogical changes and overall implementation. In most of the U.S., K-12 mathematical standards have not evolved significantly in the last 100 years, and school systems continue to run their mathematics courses as disparate blocks. In contrast, countries that have shown more success in science, technology, engineering, and mathematics education tend to teach three continuous years of integrated math wherein concepts of algebra, geometry, probability, statistics, and data analysis are presented in unison. This strategy helps students develop deeper conceptual knowledge and make stronger connections with the material.
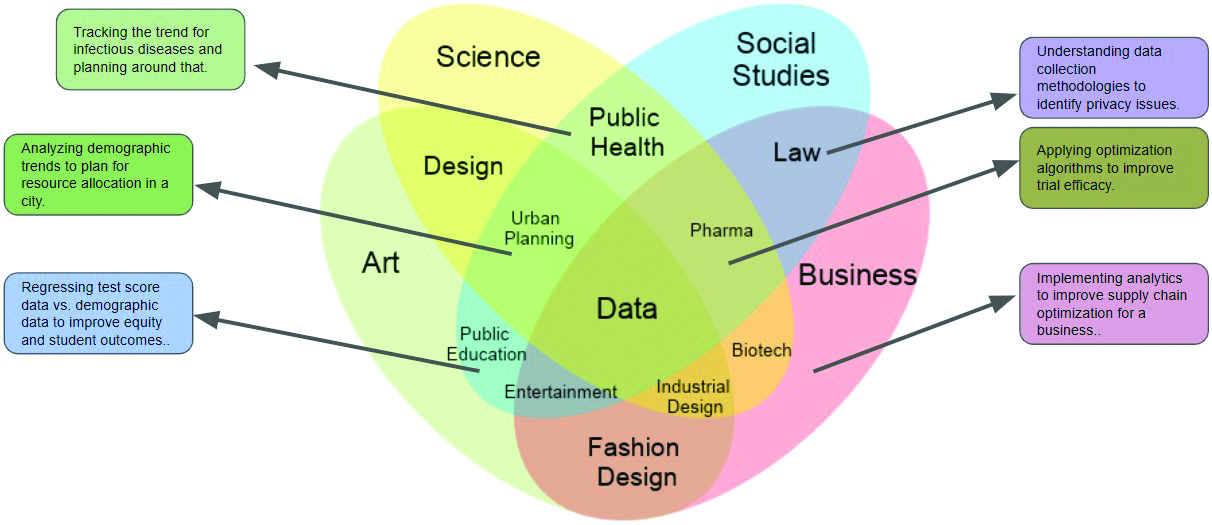
By virtue of design, data science combines elements of mathematics, statistics, and computer science to solve real-world problems (see Figure 1). Therefore, introducing students to data science at an early age allows them to incorporate inter- and intradisciplinary approaches in the problem-solving processes. A good data science curriculum should include foundational competencies like statistical and computational thinking, mathematics, communication skills, and ethics. While mathematics, statistics, and computer science tend to share certain similarities with data science—they all aim to extract knowledge from data, for example—significant differences exist in the modeling processes, size of the data, type of problem, practitioners’ backgrounds, and language in question.
The Virginia Department of Education (VDOE) has taken a timely, proactive approach to address the need for data science programming. As part of the modernizing mathematics initiative during the 2023 revisions to Virginia’s SOL, the VDOE will support school divisions in piloting a flagship data science course across the state. Participants will investigate the data science cycle via a project-based learning method that allows student choice in learning experiences (see Figure 2). The proposed course focuses on five units: data and society, data and ethics, data and communication, data modeling, and data and computing. Students will utilize open-source technology tools to identify and explore problems that involve relational database concepts and data-intensive computing in order to ultimately find solutions. They will also engage in a data science problem-solving structure to interact with large data sets and formulate problems; collect, clean, and visualize data; create data-based models; and effectively communicate data-formulated solutions.
In addition, the course intends to build data literacy through “data talks” — low-threshold, high-ceiling activities that engage and motivate students in data-related topics like correlation versus causation, bias, and appropriate visualizations; a similar technique known as “number talks” builds computational fluency. Short, targeted lessons called “mini bytes” can either offer timely instruction to the whole class or serve as differentiation or scaffolding approaches for individuals on an as-needed basis. Community and business connections permit students to investigate local and real-world problems and establish mentored relationships with data science professionals.
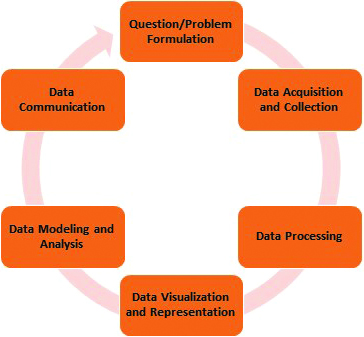
Development of the proposed data science standards involved three phases of collaboration among a diverse group of K-12 educators, industry representatives, and higher education professionals in Virginia. The phase 1 team created the course standards based on the data science cycle (see Figure 2). The phase 2 team then used feedback from the first phase as well as universal backwards design principles to identify ideas and concepts for the Data Science SOL, along with the resulting knowledge and skills. Finally, the phase 3 team generated draft unit guides, rubrics, and project templates that outlined the way in which students demonstrate evidence of learning; these materials include a self-assessment and a built-in feedback cycle from teachers, mentors, and peers.
CANVAS—Virginia’s statewide learning management system—will deliver the course’s professional learning resources during initial pilot training. A virtual version of the data science course with a dedicated virtual instructor will also be piloted via Virtual Virginia. The iterative curricular design process incorporated feedback from national and local stakeholders, and the next phase—following the Board of Education’s first review—will incorporate public feedback to further refine the standards and make the course accessible to all Virginia students.
The data science standards seek to ensure that data is collected, processed, analyzed, interpreted, visualized, and shared with a variety of stakeholders, and that information about the student experience is compiled. The experience is project-based in that students collaborate with each other and their community to investigate questions of interest through data visualization, modeling, prediction, analysis, and communication. Student teams are structurally similar to teams in the workforce, as every individual contributes their own assets to each project. These efforts are essential to the creation of a strong and diverse undergraduate and graduate workforce.
A data science skillset is valuable, easily transferable across disciplines, and fills a critical need in most workplace settings [2]. Students who study and practice data science are more likely to see themselves in different data science roles based on their unique strengths in areas like statistics, programming, and visualization. Talitha Washington, director of the Atlanta University Center Consortium’s Data Science Initiative, recognizes the value of such experience in the context of future careers. “Data science is a rapidly emerging skill that is needed to solve problems across all subjects,” she said. “Opportunities for students to broaden their abilities to read, interpret, and communicate information from data do not replace mathematics. Rather, they expand students’ knowledge for interacting in our data-driven world.”
The variety of skills and application options in the field of data science underscores the value of a diverse team, which can employ an asset-based approach and build student agency. This tactic will also help data science education move beyond a deficit model that is driven by accountability and problems in practice, and towards an asset model that is driven by a positive approach that examines students’ strengths, interests, and cultures.
References
[1] National Council of Teachers of Mathematics. (2018). Catalyzing change in high school mathematics: Initiating critical conversations. Reston, VA: NCTM.
[2] Seshaiyer, P. (2021). Novel frameworks for upskilling the mathematics education workforce. In D. Burghes & J. Hunter (Eds.), Mathematics education for sustainable economic growth and job creation (pp. 90-107). New York, NY: Routledge.
About the Authors
Tina Mazzacane
K-12 Mathematics Coordinator, Virginia Department of Education’s Office of STEM and Innovation
Tina Mazzacane is the K-12 Mathematics Coordinator at the Virginia Department of Education’s Office of STEM and Innovation. She leads the Modernizing Mathematics in Virginia initiative.
Deborah Crawford
K-12 Supervisor, Frederick County Public Schools
Deborah Crawford is the K-12 supervisor for mathematics and world language at Frederick County Public Schools. She leads the Data Science Pathways initiative for the Modernizing Mathematics in Virginia initiative.
Lisa Bussian
Mathematics Teacher, Patriot High School
Lisa Bussian is a mathematics teacher and chair of the math department at Patriot High School in Nokesville, Va., where she teaches Advanced Algebra II and AP Computer Science Principles. She is a member of the Modernizing Mathematics in Virginia Pathways Committee.
Aanand Vasudevan
Mathematics Teacher, South Lakes High School
Aanand Vasudevan is a mathematics teacher at South Lakes High School in Reston, Va., where he teaches geometry and IB Mathematics Applications and Interpretations. He is a member of the Modernizing Mathematics in Virginia Pathways Committee.
Padmanabhan Seshaiyer
Professor, George Mason University
Padmanabhan Seshaiyer is a professor of mathematical sciences at George Mason University who previously served as chair of the SIAM Diversity Advisory Committee. He works in the broad area of computational mathematics, mathematical biology, data science, biomechanics, design thinking, and STEM education. Seshaiyer is also chair of the U.S. National Academies Commission on Mathematics Instruction and Associate Director for Applied Mathematics at the Math Alliance.
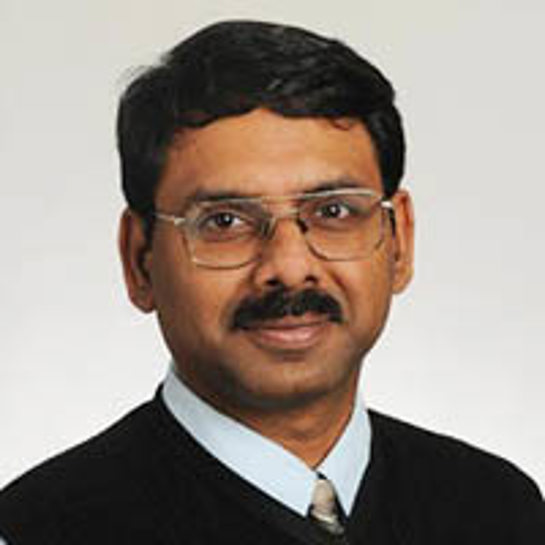
Stay Up-to-Date with Email Alerts
Sign up for our monthly newsletter and emails about other topics of your choosing.