Principles and Trends in Mathematical Imaging
The fascinating and emerging field of imaging science is situated at the interface of physics, electrical engineering, computer science, and mathematics. Its broad applications reach from photography to biomedical, seismic, and astronomical imaging. Mathematical imaging pertains to the development and analysis of mathematical models and methods that process imaging data in efficient, explainable ways. Effectively coping with the application at hand requires tools from diverse fields of mathematics that often interact in interesting manners, conversely influencing the mathematical theory in these fields. Harmonic analysis, partial differential equations (PDEs) and related variational methods, stochastics, and differential geometry are just some of the fields that are relevant to imaging science.
In the early 19th century, mathematician Joseph Fourier realized that one can represent every periodic function as a superposition of sines and cosines. This basic idea became a cornerstone of signal and image processing. As a result, the principle of analyzing and modifying functions by approximating them as linear combinations of appropriate elements from a dictionary was generalized in harmonic analysis and approximation theory. Scientists have thus begun widely utilizing wavelets and their sophisticated directional counterparts, such as curvelets and shearlets, in the multiresolution analysis of images. Indeed, the language of wavelets originated from several dialects, including square integrable group representations, bandpass filters, and windowed Fourier transforms.
Quite recently, researchers have successfully implemented nonlinear eigenfunction systems—based on variational methods like total variation regularization—for imaging tasks. This process has revealed even more challenging questions in nonlinear operator theory and computation. Furthermore, appropriate sparsity assumptions on images have made sub-Nyquist imaging systems possible, ultimately resulting in the emergence of compressive imaging systems, sparse dictionary learning techniques, and super-resolution algorithms.
Experts have long used PDEs in image restoration, particularly for edge- and coherence-enhancing nonlinear diffusion of grayscale values. The development of operator splitting methods in large-scale optimization in the 1960s essentially advanced the evolution to related variational image reconstruction methods. In the 1980s, researchers applied operator splitting methods to solve monotone inclusion equations in convex analysis; nearly 20 years later, they successfully adopted these approaches in both imaging and machine learning.
Because real data is always noisy and often results from a random measurement process—as with photonic imaging—the field of statistics is another important area of mathematical imaging. Statistics helps formalize the solutions of many image processing and analysis tasks as estimation problems for which one can provide confidence bounds and quantify uncertainty. The use of Bayesian models is therefore significant in image restoration applications.
![<strong>Figure 1.</strong> Graph-based classification of chest X-rays into different pathologies. Based on only a very small number of labeled X-rays per class, researchers aim to classify the rest. To do so, they map the X-rays into a high-dimensional feature space where known labels are propagated to the unlabeled X-rays by the graph Laplacian. Figure courtesy of [1].](/media/pjrpfung/graphxnetv2.jpg)
Researchers have also incorporated nonlocal image models, graph-based models, and mixture models into imaging techniques (see Figure 1). Optimal transport-based techniques are highly interesting for certain imaging tasks, including barycenter computation. These procedures again originated from an old mathematical problem—Gaspard Monge’s 18th-century effort to transport mass at minimal costs and Leonid Kantorovich’s relaxed formulation in the 1930s—and now have an established measure-theoretic foundation upon which imaging scientists can build. Novel developments are related to multimarginal optimal transport and generalized Schrödinger bridges.
Many of the predominant mathematical problems in imaging are best formulated as inverse problems. Inverse problems involve the reconstruction of an unknown physical quantity from indirect measurements and may arise in tomographic imaging (like magnetic resonance imaging, computed tomography and positron emission tomography scans, and optical tomography), wave imaging (like ultrasound or seismic imaging), and hybrid imaging (like photoacoustic tomography). Most of these types of inverse problems are ill-posed and thus require appropriate mathematical treatment to recover meaningful solutions. Mathematical concepts from functional analysis, statistics, and numerical analysis play an important role.
New imaging techniques and hardware—i.e., novel developments in photoacoustic tomography, optical tomography, and lensless imaging—have also shaped mathematical imaging. Recent advances in quantum imaging suggest the possibility of overcoming “Rayleigh’s curse,” which is a statistical limit on resolution, via specially-designed quantum optical systems. Diffusion tensor imaging in medical scenarios and electron backscatter tomography in materials science provide “images” that are manifold-valued, thus rendering differential geometry tools essential. Dynamical imaging—the treatment of videos and multimodal images—pertains to questions in optical flow (see Figure 2), image metamorphosis, and registration. The concept of metamorphosis particularly endows the space of images with a nonlinear Riemannian structure, which one can use in applications like diffeomorphism estimation by minimizing the path energies of corresponding geodesics.
![<strong>Figure 2.</strong> Crack detection in materials during tensile tests by optical flow variational methods. Even cracks that are not visible to the human eye (gray value images) can be detected. Figure courtesy of [2].](/media/ezcfaisg/figure2.jpg)
Finally, the emergence of machine learning and impressive performance of deep neural networks (DNNs) in many imaging applications have fueled a significant amount of research in data-driven methods. Discovering the mathematical principles behind the seemingly simple concept of neural networks is a tremendous undertaking. Understanding the design, training, and performance of DNNs will again draw from nearly every area of mathematics and surely lead to even more insights and theories that feed back into these fields. Many interesting questions remain, as it appears that standard DNNs are mostly insufficient for solving imaging problems — especially in areas like medical and scientific imaging where training data is scarce. Although these data are embedded in high dimensions, they actually stay near a low-dimensional manifold; this fact calls for deep geometric learning of big data to explore and exploit the underlying geometry in many applications. Generalizing neural networks to arbitrary geometric domains like graphs and manifolds and improving the accuracy, efficiency, and interpretability of the learning process therefore remains a challenge.
New developments in machine learning have the potential to solve data analysis and processing tasks that were previously unthinkable. At the same time, researchers must confront the limitations of these techniques—such as data bias, instabilities, and computational challenges—when applying them to practical imaging problems. This contrast between promise and practice calls for novel developments in mathematical imaging that could potentially help combine mathematical modeling and analysis with data-driven components. The question of how to best do this, however, remains unanswered.
The broad and diverse range of mathematical topics in imaging science demonstrates the true richness of this area for mathematical research and explains the subject’s lure for mathematicians from various fields within and beyond academia. New trends and developments in mathematical imaging are also inspiring newfound career perspectives for early-career researchers, as the emergence of data science within imaging has created employment opportunities in many industrial sectors.
References
[1] Aviles-Rivero, A.I., Papadakis, N., Li., R., Sellars, P., Fan, Q., Tan, R.T., & Schönlieb, C.-B. (2019). GraphXNET – chest X-ray classification under extreme minimal supervision. In International conference on medical image computing and computer assisted intervention. Shenzen, China.
[2] Balle, F., Beck, T., Eifler, D., Fitschen, J.H., Schuff, S., & Steidl, G. (2019). Strain analysis by a total generalized variation regularized optical flow model. Inverse Prob. Sci. Engin., 27(4), 540-564.
About the Authors
Carola-Bibiane Schönlieb
Professor, University of Cambridge
Carola-Bibiane Schönlieb is a professor of applied mathematics at the University of Cambridge, where she is head of the Cambridge Image Analysis Group. Her current research interests focus on variational methods, partial differential equations and machine learning for image analysis, image processing and inverse imaging problems, and the mathematical foundations of machine learning. Schönlieb is also a member of the SIAM Council.
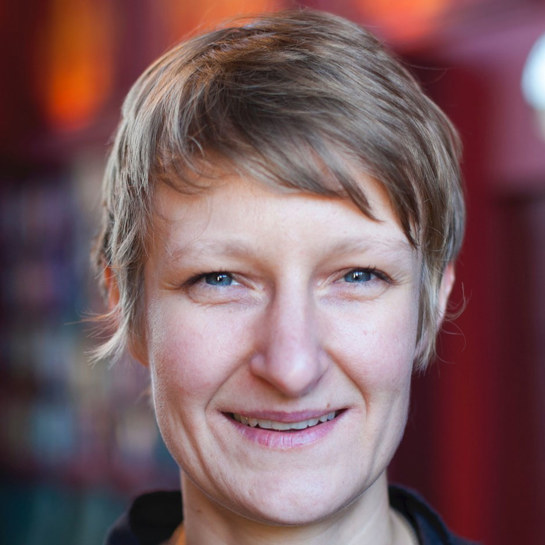
Hongkai Zhao
Professor, Duke University
Hongkai Zhao serves as vice chair of the SIAM Activity Group on Imaging Science and is a professor of mathematics at Duke University. His research interests are in computational and applied mathematics, including numerical analysis and scientific computing, inverse problems, and image processing.
Gabriele Steidl
Professor, Technical University of Berlin
Gabriele Steidl serves as program director of the SIAM Activity Group on Imaging Science and is a professor at the Technical University of Berlin. She has previously held positions as an associate professor at the Technical University of Darmstadt and a full professor at the University of Mannheim and the University of Kaiserslautern.
Michael B. Wakin
Professor, Colorado School of Mines
Michael B. Wakin serves as secretary of the SIAM Activity Group on Imaging Science and is a professor of electrical engineering at the Colorado School of Mines. His research interests include signal and data processing via sparse, low-rank, and manifold-based models.
Stay Up-to-Date with Email Alerts
Sign up for our monthly newsletter and emails about other topics of your choosing.