Pursuing Computational Goals as an LLF-SIAM Undergraduate Fellow
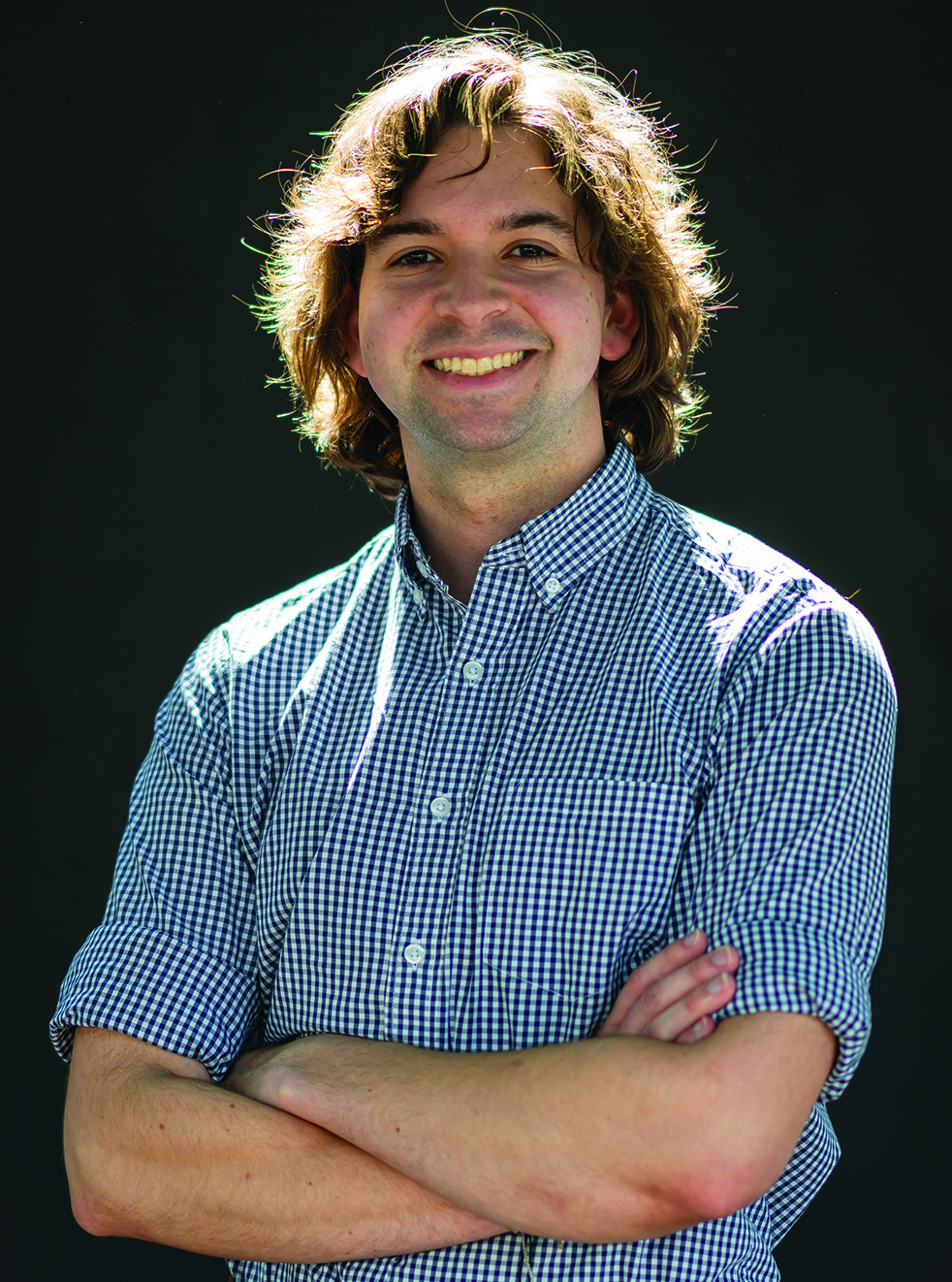
Computational science is seemingly emerging as a crucial subfield in nearly every scientific domain. A large number of researchers—from biologists to physicists—are beginning to describe themselves as “computational.” Scientists with experimental or theoretical backgrounds may therefore wonder if they have the requisite skill sets to pursue these new lines of inquiry. Several years ago, I embarked on the path towards becoming a computational scientist and resolved to work at the intersection of machine learning and physical science.
Last summer, I was afforded the opportunity to intern at Lawrence Livermore National Laboratory (LLNL) as the 2023 inaugural LLF-SIAM Undergraduate Fellow. SIAM partnered with the Livermore Lab Foundation (LLF) to support an undergraduate student internship at LLNL’s world-class research facility that offers one lucky participant the chance to advance their applied mathematics knowledge and address relevant scientific and technological challenges. Bruce Hendrickson, Principal Associate Director for Computing at LLNL, made the initial connection between LLF and SIAM. Here, I will describe both my transition to computational research and the Fellowship’s role in bringing clarity to my path.
In my experience, students or professionals who are considering computational work frequently have a background in the natural sciences. Often, they seek to develop a sense of intuition for incorporating computational and data science into their respective fields. Experience in the natural sciences does allow for a relatively seamless transition to computation because of the major overlap in prerequisite knowledge between the two areas. In contrast, computer scientists who wish to apply their expertise to other scientific fields may encounter more difficulties due to their lack of familiarity with the targeted domain.
I began my journey with no background whatsoever in science, technology, engineering, and mathematics (STEM), having switched my major from fine arts to computer science (I recently graduated with bachelor’s degrees in both computer science and digital media and design from the University of Connecticut). Three key aspects contributed to my successful transition. First, I learned how to study in a manner that worked for me — a crucial part of finding success as a STEM student. Second, I recognized math’s relevance to my field of choice. Concepts in probability, multivariable calculus, and linear algebra take on new meaning when they are contextualized in a neural network. Third, research experiences helped me appreciate the symbiotic relationship between computer science and the natural sciences. By abstracting the physical world’s phenomena into data, I realized that I can use techniques such as machine learning to extract new information; conversely, physical phenomena can inspire novel algorithms in areas like genetic programming or neural networks.
My newfound understanding and appreciation for STEM led me to wonder how I could apply computer science to real-world problems in other scientific domains. While university research certainly provided a foundation, the LLF-SIAM Undergraduate Fellowship ultimately offered a broader view of this enterprise. The Fellowship targets students who are interested in applied mathematics, computational science, and/or data science, though LLF also sponsors additional Fellowships in other areas of STEM. Students are paired with an LLNL staff mentor whose research aligns with their interests and skills; they take full ownership of their assigned project components and often collaborate with multiple team members. By interning at a national laboratory, Fellows receive a paid, full-time, 10- to 12-week opportunity to work on major problems with the resources and support of a high-level research center. LLF also hosts a Fellows Week, during which the recipients of various LLF Fellowships gather to connect with each other and foster career-related skills.
I applied for the LLF-SIAM Under-graduate Fellowship to acquire additional research experience and gain a better understanding of what a career in computational science might look like for me. Additionally, I hoped to encounter researchers whose work blended computation with applications to particular scientific domains. Though I was officially a computing intern, I was paired with Diego Oyarzun Dinamarca and Aldair Gongora: staff scientists in mechanical and materials engineering. My assignment involved uncertainty quantification for heat transfer simulations within an overarching project about autonomous experimentation, which combines robotics and real-world experiments with machine learning methods like Bayesian optimization. The tasks of performing experiments and making adjustments are automated via robotics and machine learning respectively — an approach that accelerates the iterative process of experimentation to reach a particular goal.
My project sought to reduce the quantity of required experiments for certain heat transfer problems. I spent half of my time at a desk working with programmers, and the other half in a wet lab amongst chemists and engineers. This dual environment allowed me to interact with researchers and interns who had a diverse array of expertise. Some staff members were kind enough to have lunch with me and answer my questions about their work; our conversations offered insight into possible areas of focus for my career. I had also been concerned about the feasibility of working in both computer and physical science, but I met a number of individuals who successfully bridged this gap and came from a variety of backgrounds. Some of these researchers were computer scientists who were pursuing physical science projects and possessed only minimal domain knowledge, while others were physical scientists who had taken up a computational role while still focusing on their domain. Despite these differences, I realized that everyone and everything had its own niche; each approach had its own strengths and weaknesses that were better suited for certain projects and team structures than others. As I continue on my own career journey, I will need to figure out what niche I wish to fill.
My time as an LLF-SIAM Undergraduate Fellow provided clarity and answers to some of my lingering career questions. There is no one correct path that intersects computer science and the natural sciences. Instead, a spectrum of approaches—each with requirements that depend on one’s specific background—offer different types of opportunities. The mentorship and knowledge that I gained from the Fellowship were invaluable, and I hope that students with similar aspirations in the SIAM community and beyond will have equally positive experiences in the years to come.
SIAM looks forward to partnering with LLF again in 2024 to sponsor another LLF-SIAM Undergraduate Fellow, who will be selected in the coming months. If you have questions about philanthropically supporting this partnership or other undergraduate programs at SIAM, please contact Abby Addy, Director of Development and Corporate Relations, at aaddy@siam.org or (267) 648-3529.
About the Author
Everett Grethel
Academic Graduate Appointee, Lawrence Livermore National Laboratory
Everett Grethel is an Academic Graduate Appointee at Lawrence Livermore National Laboratory. He aims to develop methods that handle the unique challenges of machine learning in materials science and other physical sciences.
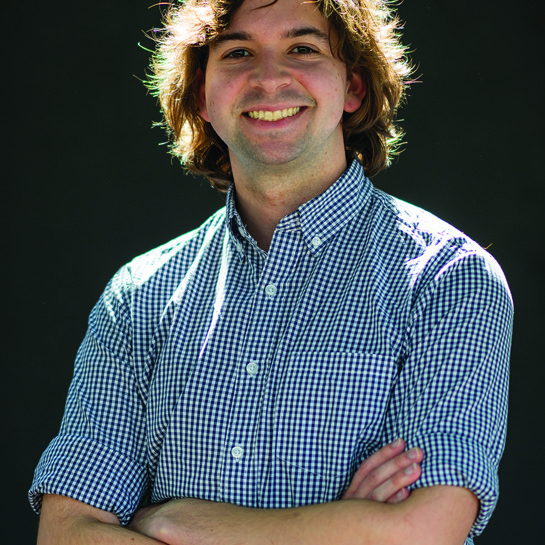
Stay Up-to-Date with Email Alerts
Sign up for our monthly newsletter and emails about other topics of your choosing.