Reflections on March Mathness
Every March, the U.S. goes mad — for March Madness. For those of you outside of the U.S., March Madness is an annual, highly anticipated single-elimination basketball tournament for National Collegiate Athletic Association Division I teams. WalletHub estimated that corporate losses due to unproductive workers during the 2023 iteration of March Madness totaled $16.3 billion [1]. They also reported that 37 percent of Americans are willing to call in sick or skip work to watch the games.
The hype begins well before a single ball bounces in the tournament itself. The initial match-ups are announced on Selection Sunday: the Sunday before the first day of the games (this year, it fell on March 12). Over the subsequent days, millions of people complete brackets that predict their proposed winners for the 63 games that comprise the tournament.
While producing a perfect bracket is of course possible, exponential growth gives rise to its improbability. There are \(2^{63}\)—or \(9,233,372,036,854,775,808\)—unique brackets for each tournament. For perspective, note that \(2^{63}\) seconds is almost 300 billion years; picking the perfect bracket out of the entire set of possibilities is therefore akin to me randomly choosing one second in 300 billion years and you accurately selecting the exact second that I chose. This near impossibility provides context to the fact that a perfect bracket has never been submitted to ESPN, CBS, or Yahoo Sports — despite the fact that fans sent more than 15 million brackets to ESPN in 2022 alone.
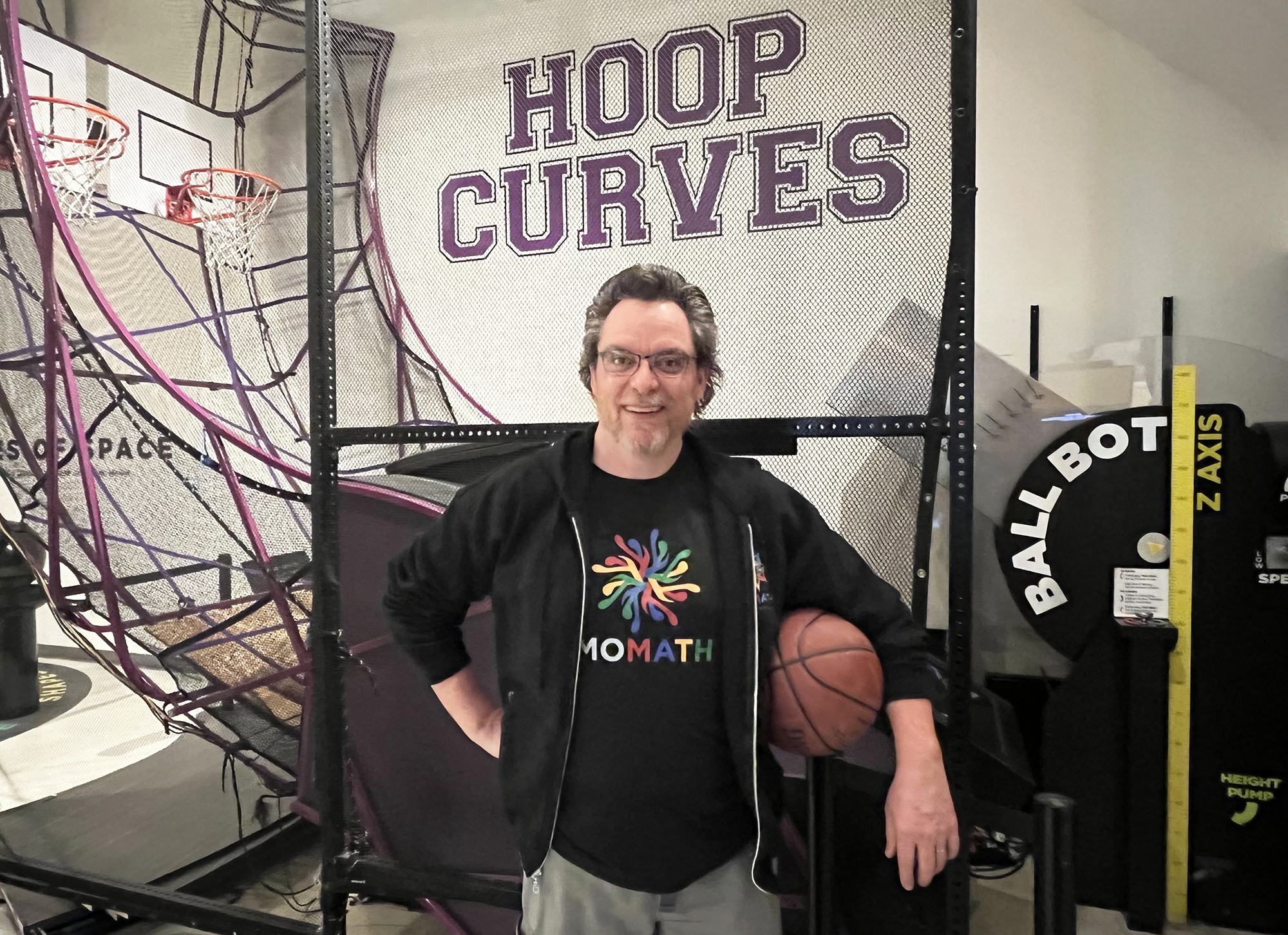
This period of bracket making encompasses a certain madness for me as well; I’m interviewed on the radio, quoted in print media, and make TV appearances every year. For this year’s tournament, The New York Times and CNN interviewed me even before Selection Sunday. So how did I end up specializing in brackets and sports analytics? In 2009, I worked with my collaborator Amy Langville of the College of Charleston and our student researchers to apply new ranking research to March Madness. Our efforts adapted the Colley and Massey methods, which officials used during the Bowl Championship Series era of college football to select teams for New Year’s Day bowl games. Both methods utilize linear systems to rank teams; their success launched me into the field that is often known as bracketology.
The Massey method derives from a least-squares formulation. We start by defining the point differential for a single game between a winning team \(W\) and a losing team \(L\) as \(d_{WL} = \textrm{points}_W - \textrm{points}_L\). The Massey method derives its linear system from the assumption that \(r_W - r_L = d_{WL}\), where \(r_W\) and \(r_L\) are the respective Massey ratings for the winning and losing teams. Applying least squares to this overdetermined system yields the normal equations \(M\mathbf{r} = \mathbf{p}\), where \(\mathbf{r}\) is the ratings vector. Adding a restriction to specify that all of the ratings sum to zero resolves this singular system, and replacing the last row of \(\left[ \begin{array}{c|c} M & \mathbf{p} \end{array}\right]\) with \(\left[ \begin{array}{cccc|c} 1& 1& \ldots& 1 & 0 \end{array}\right]\) results in the nonsingular system. While infinitely many solutions are still possible (when the graph of the season is disconnected, for instance), a lot of systems now have a unique solution.
Another approach involves forming the linear system \(C\mathbf{r} =\mathbf{b}\) from Colley’s method, where \(C\) is the Colley matrix and \(\mathbf{r}\) is the ratings as a vector. Here, \(C = M + 2I\) and the vector \(\mathbf{b}\) contains information about each teams’ number of wins and losses: \(b_i = 1+\frac{w_i-l_i}{2}\). Because the Colley matrix \(C\) is strictly diagonally dominant, the Colley method produces a unique rating vector \(\mathbf{r}\).
The Massey and Colley methods generate two different brackets, and my research with Langville and our students allowed us to weight predictive elements. For example, weighting games by time identifies teams that are playing well as the tournament begins. Our math-based brackets solve these linear systems and assume that a better-ranked team wins any match-up. In 2009, this work produced a bracket that beat over 97 percent of the more than four million brackets that were submitted to ESPN. The following year, I taught a portion of these methods to undergraduate students at Davidson College, where I’m a professor of mathematics and computer science. One of my students created a bracket that beat over 99.9 percent of the more than five million brackets on ESPN that season.
The success of this work quickly captured the media’s attention. As a result, the madness to my March now involves participating in interviews and helping people—including the general public—create brackets. Given these responsibilities, I rarely make a full bracket myself. Media personalities typically ask me to make predictions on matters like big upsets, the “Final Four” teams in the tournament, and the national champion. Doing so takes time, analysis, and care, and I usually get help from a group of students. I’m currently serving as the 2022-23 Distinguished Visiting Professor at the National Museum of Mathematics (MoMath), so participants from my eight-week ranking course at MoMath assisted with the 2023 predictions.
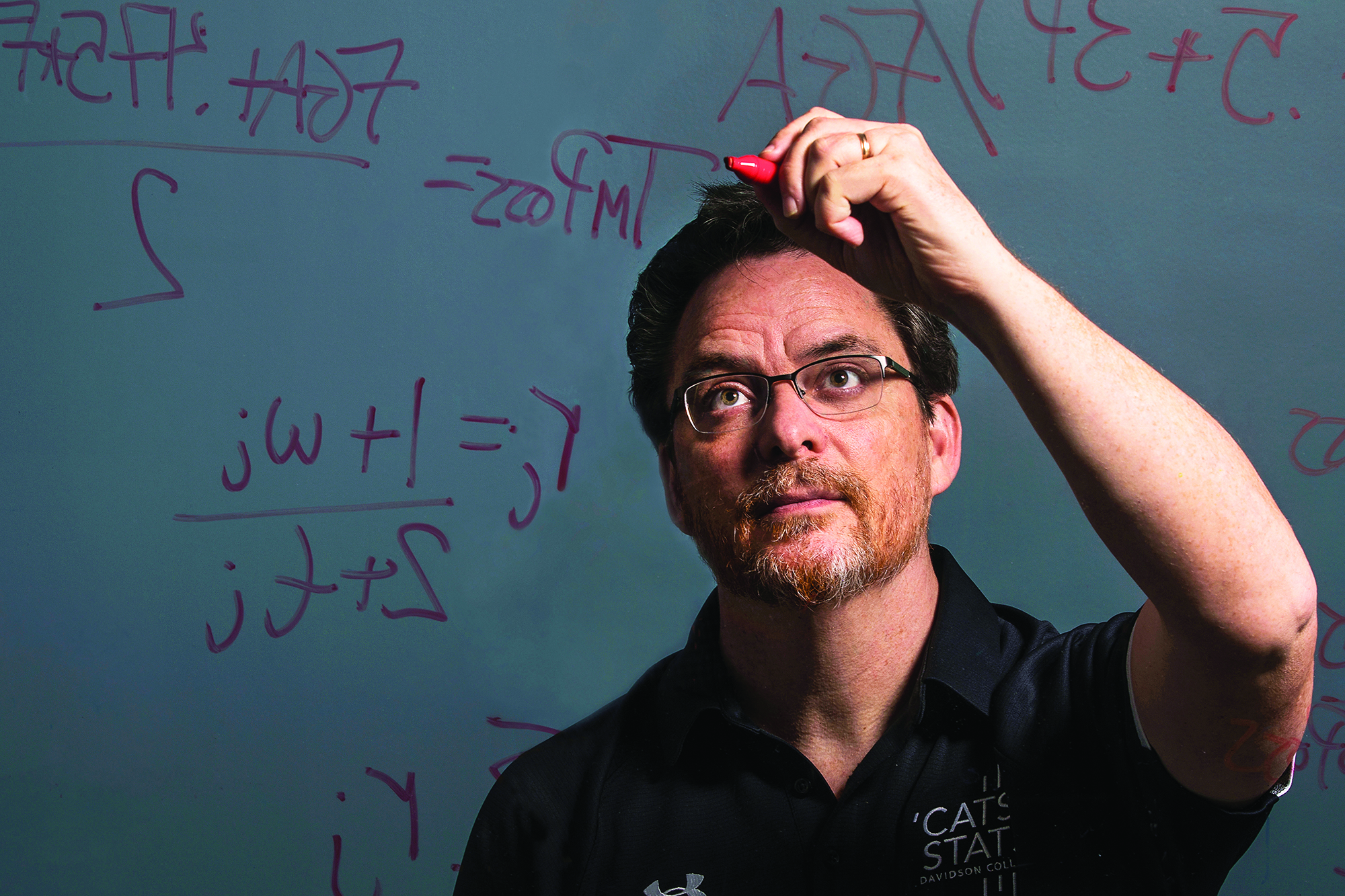
How does this work overlap with the interests of the SIAM community? First, there is outreach. I lead March Madness events across the U.S. for students, established researchers, and members of the public. Each year, as many as 600 secondary students come to Davidson College for a math and brackets workshop that prepares them for school-wide competitions. Second, there is education. I teach the bracket methods in various linear algebra courses, from general education to upper-level mathematics. If the course occurs in the spring, I introduce the techniques in time for the students to make brackets for March Madness.
Third, there is research. A number of open questions about March Madness have yet to be explored. Massey and Colley are only two possible ranking methods; we can also apply other techniques like the Elo rating system or Microsoft’s TrueSkill ranking system. Furthermore, we might wish to integrate adaptations beyond weighting recency to create more predictive brackets. My students weighted home versus away games in 2010, which proved to be more accurate than our 2009 efforts that only weighted games by date of play. And on Selection Sunday, we can consider how to better predict which methods will be most effective in a tournament. Sometimes, both Massey and Colley do very well; in other years, one method struggles more than the other. Should we employ different ranking methods if we are looking for big upsets versus solely focusing on predicting the national champion? Because March Madness comprises only 63 games per tournament, the sample size of data is quite small. Even if we analyzed every March Madness game ever played, we’d only have a total of several thousand games — not the tens or hundreds of thousands of games that are necessary to hone and train a method. Regardless, bracketology can engage students in data science and help those who are interested in sports analytics create samples of their work.
My efforts in March Madness springboarded my research into the field of sports analytics. I am now a consultant for the National Basketball Association (NBA) League Office, where I explore matters in game integrity. I’ve fielded analytics queries from ESPN and The New York Times, worked with undergraduates on questions posed by National Football League and NBA teams, and aided the U.S. Olympic and Paralympic Committee.
For most people, March Madness crops up annually. For me, it never ends. I’m always looking for novel insights, new ways to develop ranking methods in data science, and fresh opportunities to engage the public in mathematics. This work is an applied context for open questions and offers the chance for individuals to step further into new territories of understanding.
References
[1] Kiernan, J.S. (2023, March 7). 2023 March Madness stats & facts. WalletHub. Retrieved from https://wallethub.com/blog/march-madness-statistics/11016.
About the Author
Tim Chartier
Professor, Davidson College
Tim Chartier is the 2022-23 Distinguished Visiting Professor at the National Museum of Mathematics and the Joseph R. Morton Professor of Mathematics and Computer Science at Davidson College. He received an Alfred P. Sloan Research Fellowship and a national teaching award from the Mathematical Association of America. Chartier’s most recent publication is 2022’s Get in the Game: An Interactive Introduction to Sports Analytics (published by the University of Chicago Press). He has also worked with Google and Pixar on their K-12 educational initiatives.
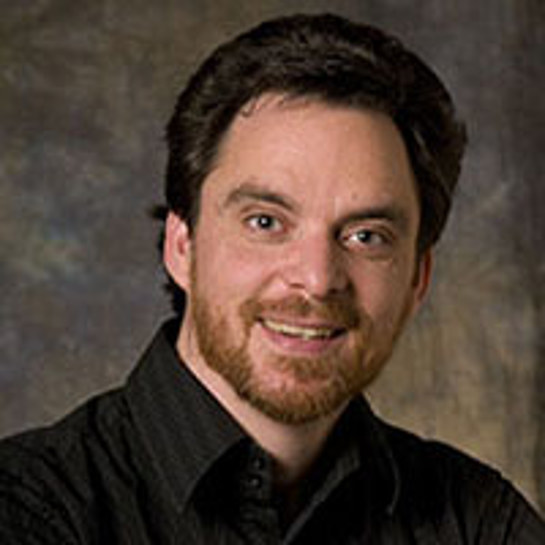
Stay Up-to-Date with Email Alerts
Sign up for our monthly newsletter and emails about other topics of your choosing.