Robotics-assisted Stroke Rehabilitation Device Uses Machine Learning to Classify Residual Severity
Every 40 seconds, someone in the U.S. has a stroke. Stroke is a serious medical emergency and a leading cause of adult disability worldwide. It can affect individuals at any stage of life, although risk does increases with age. During an ischemic stroke, a blood vessel obstruction hinders blood flow to the brain, preventing the flow of oxygen and causing brain cells to die. In contrast, a hemorrhagic stroke occurs when a blood vessel bursts and triggers a brain bleed. “Stroke is essentially a heart attack in your brain,” Russell Jeter of Georgia State University said. “It’s really bad, and a lot of people die from it.”
Those who do survive typically exhibit certain post-stroke symptoms—in the form of visual, cognitive, sensory, speech, and motor impairments—that range in severity. Some symptoms are extreme—a complete lack of motor function in upper or lower extremities, for instance—while others are fairly mild. But regardless of symptom severity, stroke recovery is an ongoing, lifelong process. “You never really get completely better,” Jeter said. “You’re always living with some level of impairment.”
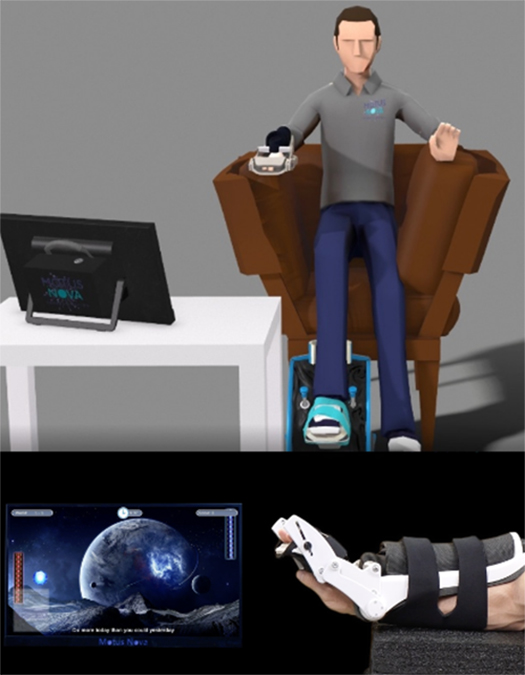
Most stroke patients attend physical and occupational therapy sessions that involve stretches and repetitive exercises for both gross and fine motor control; some also perform cognitive drills to improve memory, vision, and speech. Unfortunately, treatment can be both expensive and difficult to navigate. During the 2024 SIAM Conference on Mathematics of Data Science, which is currently taking place in Atlanta, Ga., Jeter introduced a unique in-home stroke rehabilitation robotic device that improves range of motion and autonomously classifies a patient’s level of stroke residual severity. The device, which is a product of Motus Nova, is the result of a collaborative project between both academic and industry researchers.
Jeter began with the concept of neuroplasticity: the brain’s ability to change, adapt, and construct new neural pathways in response to trauma or disease. This unique capability plays a key role in stroke rehabilitation. “If they are damaged, your neurons have mechanisms of rebuilding functions and connections,” he said. Because stroke typically impacts only one side of the body, patients must actively use their affected limbs in order to retrain the brain and regain functionality.
In the 1990s, behavioral neuroscientist Edward Taub started to examine constraint-induced movement therapy as a possible treatment for victims of stroke. This intensive form of rehabilitation—which involves restraining a patient’s working arm for a prolonged period of time and forcing them to utilize the affected one—saw much success and achieved major progress in the area of neuroplasticity. Post-stroke physical therapy broadly adapts this concept on a lesser scale with repetitive exercises.
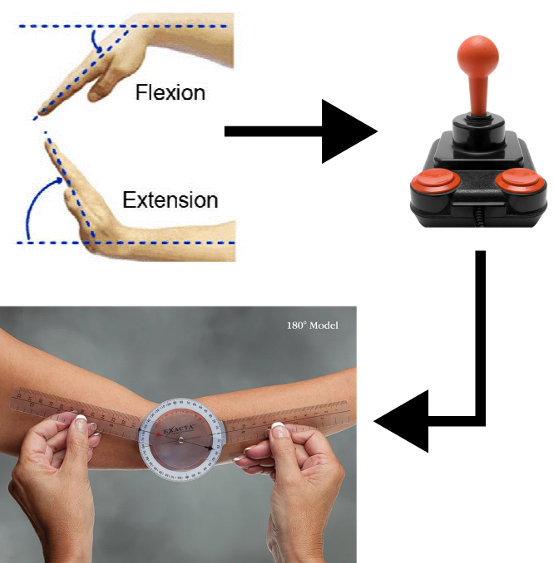
Inspired by existing recovery techniques, Motus Nova developed a robotics-assisted therapy setup—with two separate products for the hand and foot—for independent use (see Figure 1). “You do the repetitive motions of physical therapy, forcing you to do gross or fine motor control exercises,” Jeter said. “But we developed the technology to do this in the home.” Patients who use the Motus Hand sit in front of a computer, strap the exoskeleton over their wrist, and perform high-dose repetitive movements by playing video games. The apparatus converts a sensor in the wrist joint to a joystick controller input (see Figure 2). It also contains an air pump that allows the device to apply assistance and stretch the joints of people with very low levels of functionality.
Jeter identified three levels of classification for stroke residual severity: (i) No range of motion, (ii) low range of motion, and (iii) high range of motion. He then described the process of data collection in the form of a simple classification problem (see Figure 3). The apparatus collects roughly 30 data points per second (to correlate with 30 frames per second in associated video games). Jeter and his colleagues gathered this high-resolution time series data from 33 patients, processed and transformed it via sensors and joystick inputs, and saved the resulting angle data in a time series database. Because the raw data is naturally messy with many high-frequency oscillations, the team filtered the outliers, conducted smoothing, and ultimately generated a list of summary statistics (see Figure 2). “We try to drop the time series data entirely because our goal is to develop something that clinicians in inpatient or outpatient facilities can use,” Jeter said.
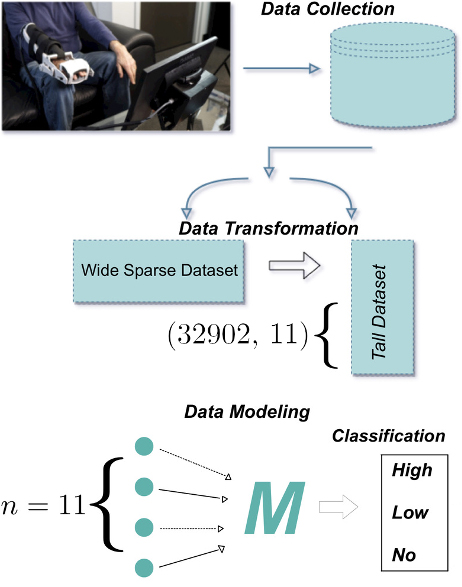
During this time, a clinician also assessed everyone in the cohort at regular intervals and ultimately classified each patient into one of the three aforementioned categories; these labels helped to inform the autonomous classification process. After conducting dimensionality reduction tests, applying principal component analysis, and assessing the correlation between individual data points, Jeter and his collaborators found that a decision tree method called light gradient boosting provided the most reliable autonomous detection of stroke residual severity. This model—which constructs several weak learner models with a weighted average to a final classifier—achieved a 96 percent accuracy on the 10-fold cross validation on an 80/20 random split of the data; its classification of patients with a low range of motion was especially successful.
To conclude, Jeter’s classifier accurately assesses stroke residual severity level and provides targeted therapy to patients as they work to regain function. “If you can determine someone’s range of motion in real time by looking at their therapy data, you can react to it in real time,” Jeter said. “If you know someone has low movement, you’re not going to provide them with fine motor control exercise.” This type of autonomous in-home technology can also be employed in inpatient or outpatient settings to reduce the burden on clinicians and allow them the freedom to see more patients.
About the Author
Lina Sorg
Managing editor, SIAM News
Lina Sorg is the managing editor of SIAM News.
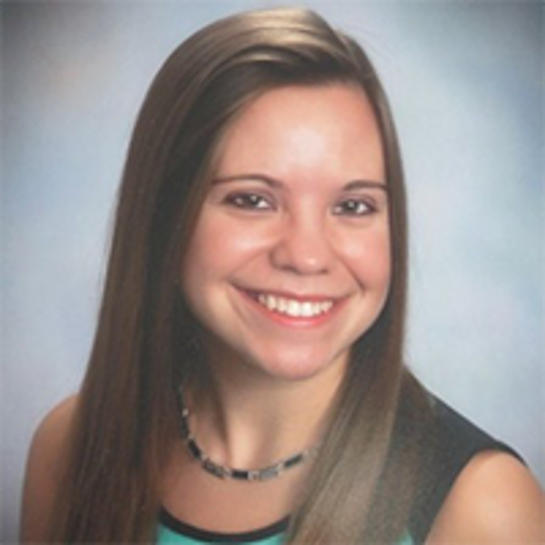
Related Reading
Stay Up-to-Date with Email Alerts
Sign up for our monthly newsletter and emails about other topics of your choosing.