SIAM Task Force Anticipates Future Directions of Computational Science
The multidisciplinary field of computational science—which lies at the intersection of mathematics, computing, and various application domains—plays a pivotal role in scientific discovery, industrial innovation, and national security. Its rapidly evolving landscape inspired the 2024 SIAM Task Force Report on the Future of Computational Science, which charts an ambitious growth trajectory for the coming decades. This comprehensive document synthesizes the collective wisdom of a diverse array of Task Force members with firsthand experience in academia, industry, and national laboratories.
The Task Force Report proposes a framework for the holistic discussion of emerging challenges and opportunities at the junction of traditional computational science, artificial intelligence (AI), and high-performance computing (HPC). Relevant challenges include the need for new hardware and software advances, as well as strategic investments to recruit and train diverse talent within the computational science workforce. The report also identifies three areas in which computational science can actively enhance both science and society: (i) Investments in software tools that bolster the impact of exascale computing, (ii) support for data science infrastructures that enable the scalable fusion of data from diverse sources, and (iii) improvements to the reliability and trustworthiness of AI that facilitate its integration with simulations and decision-making processes.
A Critical Juncture in Computational Science
Computational science is currently at a critical juncture that offers significant opportunities for advancement alongside formidable challenges that threaten to impede progress. The advent of exascale computing—a milestone that is heralded by the U.S. Department of Energy’s Exascale Computing Project (ECP)—marks a transformative moment for the field (see Figure 1). This leap in computational capability is poised to revolutionize scientific inquiry by enabling simulations of unprecedented fidelity, facilitating real-time data analytics at massive scales, and promoting the exploration of complex phenomena that were previously out of reach.
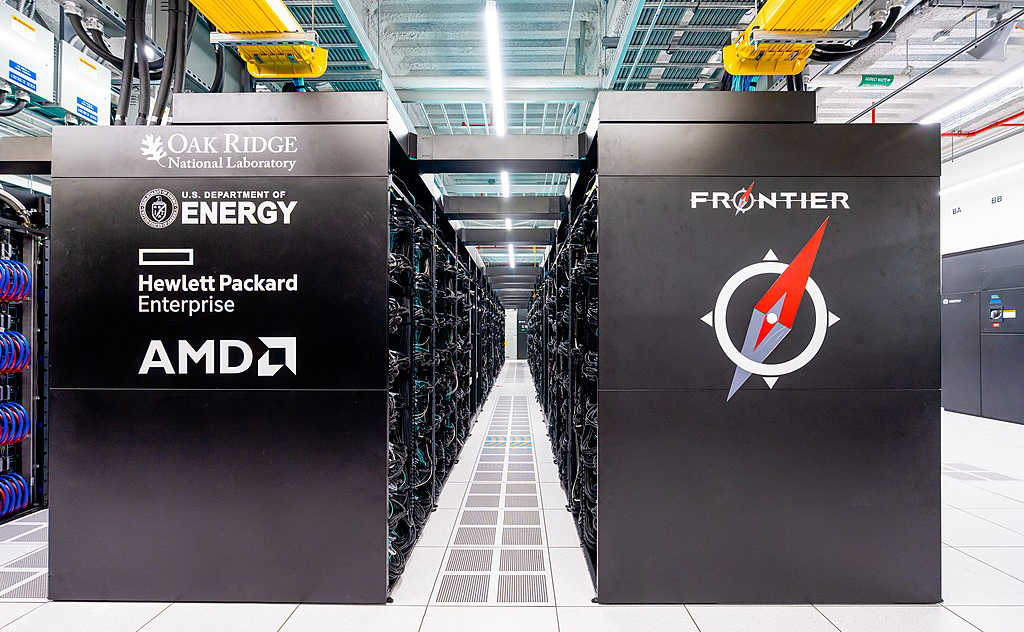
SIAM’s report highlights two noteworthy developments that can accelerate scientific discovery through exascale computing: (i) The emergence of digital twins and (ii) the synergy between AI and computer simulations. The ECP encourages such progress through its extensive provision of exascale-ready software — including tools, libraries, and a diverse suite of applications. However, the community must ensure the sustainability and ongoing enhancement of these tools. Without continuous investment, computational science applications may struggle to adapt to the exascale computing environment and fail to maximize its potential. The report thus advocates for further investments in mathematics, computer science, application science, and system software to support research and development activities that fully leverage the potential of exascale computing.
However, the path forward is riddled with obstacles; an especially pressing challenge is the evolving landscape of HPC. The end of traditional scaling laws—such as Moore’s law, which historically drove increases in computing performance—necessitates a paradigm shift towards heterogeneous computing architectures. These architectures promise to sustain the momentum of computational advancements by incorporating specialized hardware and potentially disruptive technologies like quantum processors. But they also introduce enormous complexities that pertain to software compatibility, algorithm optimization, and infrastructure adaptation that require immense care and forward-thinking strategies.
The Data Deluge
In tandem with computational advancements, the Task Force Report highlights data science’s transformative potential to propel scientific breakthroughs. Although the surge of data from scientific experiments, sensor networks, and simulations offers exciting new lines of inquiry, this deluge also poses formidable challenges in data management, analysis, and integration. To navigate such a complex data landscape, the report advocates for substantial investments in data science infrastructure and proposes a multi-pronged approach that promotes the development of sophisticated tools and algorithms for the scientific community. In particular, data fusion techniques can effectively synthesize information from disparate sources to enable a more holistic understanding of scientific phenomena. And real-time analytics can process and interpret data streams on the fly — an increasingly important capability in fields that require rapid decision-making, such as environmental monitoring and emergency response.
The report also emphasizes the value of digital twins: high-fidelity virtual models of natural or engineered systems that are continuously updated with real-world data. These models bridge the gap between theoretical research and practical application and offer unparalleled opportunities for simulation, prediction, and optimization. As such, Task Force members envision a future of accelerated scientific inquiry that harnesses the power of data science, computational advancements, digital twins, and other emerging technologies to produce revolutionary discoveries and innovations across various domains.
Artificial Intelligence and Machine Learning
The Task Force Report further illuminates the significance of AI and machine learning (ML) in scientific research. Recent strides in these fields have resulted in potent tools that enhance data analysis, expedite simulations, and yield novel scientific insights. However, focused research efforts are needed to tailor these commercial advancements to the nuanced requirements of scientific applications. Sparse datasets, physical models within AI structures, and the reliability and interpretability of AI-driven scientific outcomes all warrant immediate attention.
Unlike commercial applications that often benefit from vast, densely populated datasets, the scientific community frequently grapples with sparse datasets. These datasets—which are characterized by their limited size, irregular sampling, or incompleteness—pose significant hurdles for traditional AI and ML algorithms that are accustomed to learning from large volumes of data. Beyond the issue of data sparsity, the integration of domain-specific physical laws and principles with the computational prowess of AI algorithms could enhance AI-driven predictions and outcomes. In addition, the importance of reliability and interpretability in this context cannot be overstated. Given their potential real-world implications, AI- and ML-informed decisions must be accurate, transparent, and justifiable.
A Skilled and Diverse Workforce
Finally, the Task Force Report emphasizes the need for a skilled and diverse workforce that can navigate the interdisciplinary terrains of computational science. It notes current shortcomings and advocates for innovative educational programs and initiatives that support new talent from historically underrepresented communities. Diversity within the computational science workforce is critical as both a matter of equity and a strategic imperative because it can inspire innovative solutions and cultivate a deeper, more nuanced understanding of complex scientific problems. By fostering an environment that welcomes and supports diversity, the field of computational science will experience a broader range of insights and approaches and enhance its capacity to tackle new challenges and seize emerging opportunities. Projects that reduce barriers to entry, provide mentorship, and highlight role models will all help to further this cause.
A Call to Action
To support the ultimate vision of the 2024 SIAM Task Force Report on the Future of Computational Science, all computational science stakeholders will need to make strategic investments in technology and research, foster interdisciplinary collaborations, and commit to an inclusive and innovative scientific community. The report’s recommendations serve as both a guide for leveraging the immense potential of computational science and a call for sustained U.S. leadership in this vital domain. By embracing this roadmap, the community can create a future wherein computational science continues to serve as a linchpin for scientific advancement, technological innovation, and societal progress.
Acknowledgments: The authors would like to thank Bruce Hendrickson of Lawrence Livermore National Laboratory, chair of the SIAM Task Force on the Future of Computational Science, for his contributions to this article.
About the Authors
Elie Alhajjar
Senior Scientist, RAND Corporation
Elie Alhajjar is a senior scientist in the RAND Corporation’s Engineering and Applied Sciences Department in Washington, D.C., where he leads projects at the intersection of artificial intelligence, cybersecurity, and government. He previously held simultaneous posts as an associate professor in the U.S. Military Academy at West Point and a senior research scientist at the Army Cyber Institute. Alhajjar has a Ph.D. in mathematics from George Mason University.

Tanzima Islam
Assistant Professor, Texas State University
Tanzima Islam is an assistant professor at Texas State University who specializes in maximizing scientific returns through the synergistic use of high-performance computing, artificial intelligence, and machine learning. She holds a Ph.D. from Purdue University and pursued postdoctoral research at Lawrence Livermore National Laboratory (LLNL). Islam’s numerous accolades include the Department of Energy’s Early Career Award, an R&D 100 Award, LLNL’s Science and Technology Award, and Texas State University’s Presidential Seminar Award.
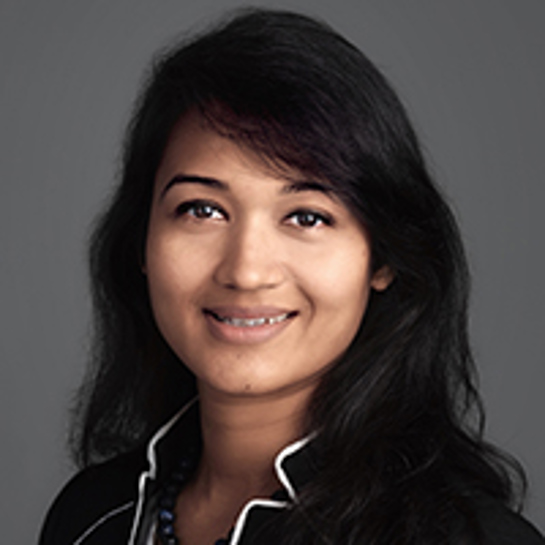