Singular Perturbations in Noisy Dynamical Systems
Idealized models are commonplace in scientific theory, though often they are not quite accurate. In some cases, the reality of an almost-but-not-quite-ideal model differs radically from the unattainable ideal model, like in such essential phenomena as chemical reactions. The source of the difference may be the effects of noise, which is frequently modeled as a random perturbation of a deterministic dynamical system.
In his John von Neumann Lecture at the 2017 SIAM Annual Meeting, held last summer in Pittsburgh, Pa., Bernard Matkowsky, John Evans Professor of Engineering Sciences and Applied Mathematics at Northwestern University, surveyed “Singular Perturbations in Noisy Dynamical Systems.” As one example, he showed how these tools can quantify the radical difference between the behavior of a particle in a potential well and one that is also subject to collisions with smaller, lighter particles, as in Brownian motion.
In the absence of collisions, the particle is trapped in the potential well. However, collisions with the particles comprising the medium through which the Brownian particle travels will eventually force it from the well, even if the strength of each individual collision is very small. The tools of matched asymptotic expansions (MAEs), and extensions thereof, can predict the expected time to exit and the probabilities of exit locations on the boundary (rim) of the well. The analysis explores the variation of such physical outcomes with the strength of an individual collision.
The dramatic difference between the ideal model and the one with small noise effects included is symptomatic of a singular perturbation. The cumulative effect of random collisions can overcome even the powerful pull of a potential well that is constraining the particle to stay in the well, though each collision results only in an extremely small movement of the particle.
Small terms with big effects call for a singular perturbation analysis. Matkowsky’s primary tools are MAEs and extensions thereof. Matching connects the so-called outer solution to the rapidly varying inner, or boundary-layer, solution. The asymptotics involve the limit as a measure of the strength of each collision—typically denoted by a small parameter \(\varepsilon\) —goes to zero. The two expansions are finite-term approximations in \(\varepsilon\).
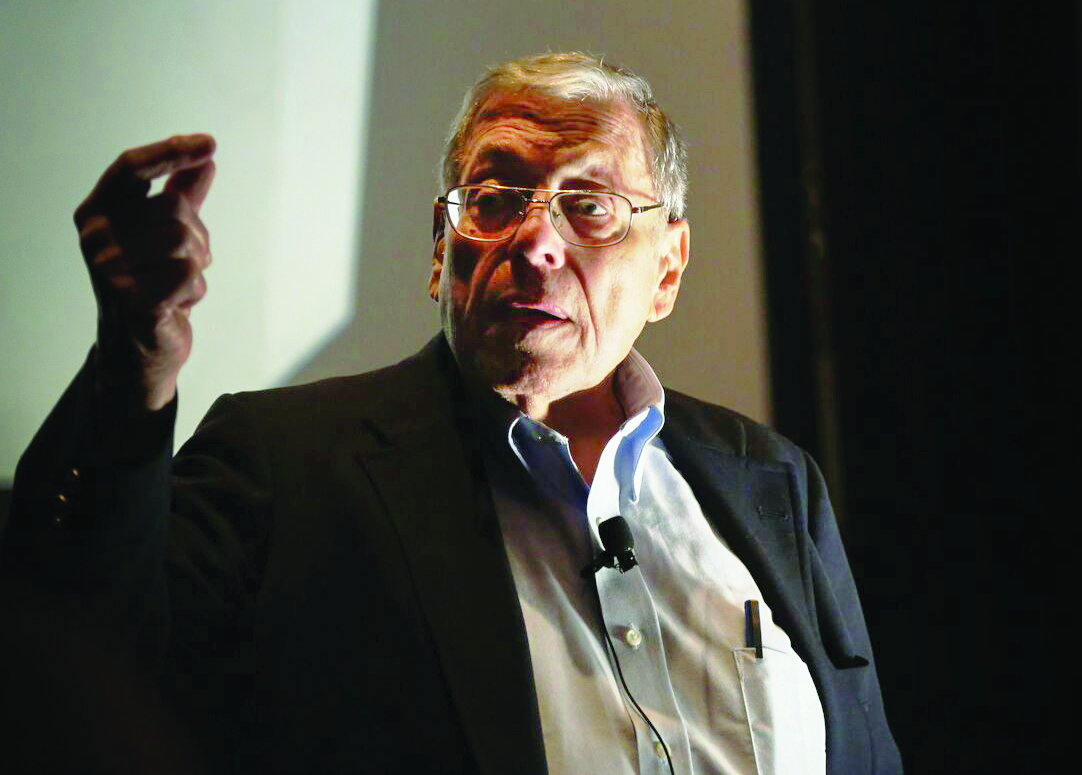
Matkowsky and his colleagues have modified or augmented MAEs to answer two central questions: (1) “What is the mean time for a particle to escape from a given starting point?” and its spatial counterpart, (2) “What is the mean probability of escape locations on the boundary given the particle’s starting point?” Matkowsky’s models are typically stochastic differential equations, mathematical representations of deterministic dynamical systems perturbed by small white noise.
The answer to these central questions are asymptotic expansions in terms of the small parameter \(\varepsilon\), which depends, e.g., on physical quantities such as temperature, the height of the potential barrier that must be overcome to escape, Boltzmann’s constant, etc. Hence, the results connect the observable outcomes of experiments to fundamental material properties.
A short but elegant path built on Ito calculus connects the stochastic dynamical system to two singularly perturbed elliptic boundary-value problems whose solutions capture the expectations of interest. The solution of a Poisson problem with zero boundary conditions gives the mean first passage time to the boundary, while the mean distribution of exit points is the Green’s function of a Dirichlet problem with given boundary conditions.
For ease of exposition, Matkowsky considers the problem in one dimension on the interval \((-a, b)\) with \(a\), \(b>0\), and \(x=0\), a stable equilibrium point. The well corresponds to the potential \(V = x^2/ 2\), so that the deterministic force is \(-x\). These deterministic problems are singularly perturbed since the diffusion term modeling the collisions is small compared to the potential force. Setting \(\varepsilon\) to zero reduces the differential equation from second-order to first-order, leaving more boundary conditions to satisfy than degrees of freedom in the outer solution.
One must account for diffusion in the so-called boundary layer. Since the solution varies rapidly there, Matkowsky stretches the spatial coordinate to find the so-called inner solution that satisfies both a second-order equation and the boundary condition. Finally, he matches the two solutions so that they connect smoothly. The final asymptotic approximation is the sum of the outer and inner expansions less their common parts (so that they are not counted twice).
The standard rubric of MAE fails for problems exhibiting boundary-layer resonance, such as the one under consideration, because there are not enough conditions to uniquely determine the solution (a constant remains undetermined). This process yields a one-parameter family of possible solutions, though only one can be the actual solution. Matkowsky and his colleagues have developed four approaches to rescue the MAE method and offer deeper insights into the asymptotics.
Two of the approaches impose an extra condition to select a single solution from this one-parameter family. The first chooses the solution that is a stationary point of the Euler-Lagrange variational principle associated with the given boundary-value problem. The second replaces the variational condition with an appropriate orthogonality condition. One may think of the former as related to the Ritz method and the latter as related to the Galerkin method.
A third approach constructs the boundary-layer function in a different way. Rather than an exponent, which is linear in the stretched variable, Matkowsky employs the Jeffreys-Wentzel-Kramers-Brillouin (JWKB) method, which allows the exponent to be nonlinear; in this case, one boundary-layer function can describe two distinct boundary layers. Finally, a fourth approach employs asymptotics beyond all orders, adding an exponentially small term to the outer expansion. The results of the four approaches are the same. Namely, exit occurs through the left (right) end point if \(a < b\) (\(b < a)\), and exit is equally likely to occur at either end point if \(a = b\).
With this panoply of tools, one can then explore the detailed behavior of such fundamental physical processes as chemical reactions and atomic migration in crystals, among others. The important physical observable, e.g., the reaction rate, can be modeled as the random escape of a particle from a potential well. Specifically, the rate is half the inverse of the mean escape time. The factor “one half” enters because the particle is equally likely to exit or return to the well once it reaches the boundary.
Matkowsky’s lecture is available from SIAM either as audio or as a PDF of his slides. Alternatively, one can access it on Matkowsky’s departmental website.
Since 1960, SIAM has annually recognized a John von Neumann lecturer for outstanding and distinguished contributions to the field of applied mathematical sciences, and effective communication of these ideas to the community. The award honors John von Neumann (1903-1957), one of the most prolific and articulate practitioners of applied mathematics in the 20th century. “The von Neumann Lecture is particularly meaningful to me since four of the previous awardees were my teachers and inspirations,” Matkowsky said. “These include Kurt Friedrichs, Peter Lax, Jurgen Moser, and most importantly to me, my advisor and friend Joe Keller.”
About the Author
Paul Davis
Professor Emeritus, Worcester Polytechnic Institute
Paul Davis is professor emeritus of mathematical sciences at Worcester Polytechnic Institute.
Stay Up-to-Date with Email Alerts
Sign up for our monthly newsletter and emails about other topics of your choosing.