Statistical Methods for Adaptive Interventions and Precision Medicine
The study of new medical interventions—or new sequences of existing interventions—is inextricably linked with the field of statistics, which extracts meaning from noise and provides researchers with a principled approach for understanding potentially large volumes of data. In recent years, the use of statistics for precision medicine—an area of medical treatment that tailors medical care via patient covariates that may be demographic, clinical, or biological—has seen tremendous development. Precision approaches also have applications in other areas, such as educational and behavioral interventions.
Adaptive Treatment Strategies in Practice: Planning Trials and Analyzing Data for Personalized Medicine, edited by Michael R. Kosorok and Erica E.M. Moodie, was published by SIAM in 2015. The volume addresses both introductory and advanced topics on statistical methods for the estimation of precision medicine (also called dynamic treatment regimes or adaptive treatment strategies). It is split into two parts and considers issues that pertain to study design as well as data analysis. The book is accessible to a wide audience of statisticians and computer scientists with a focus on machine learning, although many chapters are also appropriate for epidemiologists and medical researchers with only modest statistical backgrounds.
The following text is a short excerpt from chapter two, entitled “DTRs and SMARTs: Definitions, Designs, and Applications.” It has been modified slightly for clarity.
To address some of the shortcomings of analyzing observational data and provide prospective evidence of dynamic treatment regime (DTR) effects, much of the existing literature on clinical trial design examines DTRs [5-10]. These studies refer to the sequential multiple assignment randomized trial (SMART), wherein individuals are randomized multiple times and follow specific DTRs (see Figure 1). The intention of this type of design is to develop DTRs, estimate the outcomes for each regime in the trial, and select the most promising DTR to compare to standard of care in a follow-up randomized control trial [7]. This objective addresses the dimensionality challenge of not just answering the question “What treatment when?” but also determining how to best use tailoring variables and information before selecting treatment. Since it is unlikely that one will have optimized all of these components prior to conducting a SMART, the goal is to conduct a series of trials that build upon one another, thereby developing and refining promising DTRs and leading to a confirmatory trial that is similar to the multiphase experimental approach [1-4]. While this scenario is ideal, the time and cost of trials may limit the intent and lead practitioners to treat SMARTs as confirmatory trials.
Just as a randomized control trial is a fixed design that generally compares two or more treatments, a SMART is a fixed design that compares or constructs two or more treatment regimes. A SMART is thus a trial and a DTR is a guideline that is carried out by a physician. SMARTs aim to construct effective DTRs. The same individuals that begin a SMART are followed throughout multiple randomizations until the end of the trial, with fixed randomization probabilities and other trial operational characteristics. Therefore, SMARTs can address questions about the best treatment at certain points in time; the best sequences of treatments (or best modes of treatment delivery) depending on intermediate outcomes; the best intermediate outcomes to direct treatment; and the best ways to individualize sequences of treatments based on biological, diagnostic, and/or other patient information. As with any trial, there should be one primary objective. However, SMARTs may lead to more secondary and exploratory aims due to the tailoring of DTRs. In order for SMARTs to be feasible, the intermediate outcome must be available for assessment within a relatively short time period — likely not more than one year. This is mainly due to the scope and relevance of conducting a trial. Thus, for some diseases with long assessment periods (treatment of some breast or prostate cancers, for example), a SMART is not an appropriate choice. However, SMARTs may still be relevant when treating other comorbidities or mental health issues that are related to these types of diseases.
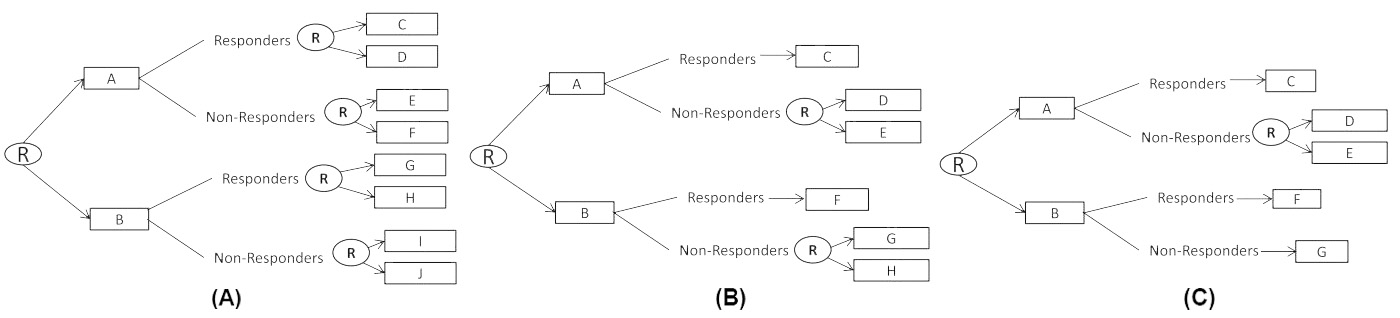
The most common SMART design includes two stages: an initial stage of randomization to one of two or more first-stage treatments, and a period of follow-up. At some point in time—or over a period of time—specialists assess the response to initial treatment and patient characteristics to subsequently re-randomize individuals for second-stage treatment. Depending on the intermediate outcome status, one may or may not be re-randomized to a treatment option. Upfront consent of sequential randomizations is recommended so that individuals are randomized to subsequent treatment once they are eligible. This allows for data usage until randomization for balance between the treatment assignments of responders and nonresponders. Conceptionally, there is no difference between upfront or sequential randomization, and both can be handled accordingly through analysis; however, sequential randomization may allow for more balanced randomization and the identification of other potential tailoring
variables at each step.
Enjoy this passage? Visit the SIAM Bookstore to learn more about Adaptive Treatment Strategies in Practice: Planning Trials and Analyzing Data for Personalized Medicine and browse other available SIAM titles.
References
[1] Box, G.E.P., Hunter, W.G., & Hunter, J.S. (1978). Statistics for experimenters: An introduction to design, data analysis, and model building. New York, NY: Wiley.
[2] Chakraborty, B. (2009). A study of non-regularity in dynamic treatment regimes and some design considerations for multicomponent interventions [Ph.D. thesis, Department of Statistics, University of Michigan]. University of Michigan Library.
[3] Collins, L.M., Chakraborty, B., Murphy, S.A., & Strecher, V.J. (2009). Comparison of a phased experimental approach and a single randomized clinical trial for developing multicomponent behavioral interventions. Clin. Trials, 6(1), 5-15.
[4] Collins, L.M., Murphy, S.A., Nair, V.N., & Strecher, V.J. (2005). A strategy for optimizing and evaluating behavioral interventions. Ann. Behav. Med., 30(1), 65-73.
[5] Lavori, P.W., & Dawson, R. (2000). A design for testing clinical strategies: Biased adaptive within-subject randomization. J. Roy. Stat. Soc. A, 163(1), 29-38.
[6] Lavori, P.W., & Dawson, R. (2004). Dynamic treatment regimes: Practical design considerations. Clin. Trials, 1(1), 9-20.
[7] Murphy, S.A. (2005). An experimental design for the development of adaptive treatment strategies. Stat. Med., 24(10), 1455-1481.
[8] Thall, P.F., Millikan, R.E., & Sung, H.G. (2000). Evaluating multiple treatment courses in clinical trials. Stat. Med., 19(8), 1011-1128.
[9] Thall, P.F., Sung, H.G., & Estey, E.H. (2002). Selecting therapeutic strategies based on efficacy and death in multicourse clinical trials. J. Am. Stat. Assoc., 97(457), 29-39.
[10] Thall, P.F., Wooten, L.H., Logothetis, C.J., Millikan, R.E., & Tannir, N.M. (2007). Bayesian and frequentist two-stage treatment strategies based on sequential failure times subject to interval censoring. Stat. Med., 26(26), 4687-4702.
About the Authors
Michael R. Kosorok
W.R. Kenan, Jr. Distinguished Professor of Biostatistics, University of North Carolina, Chapel Hill
Michael R. Kosorok is a W.R. Kenan, Jr. Distinguished Professor of Biostatistics and a professor of statistics and operations research at the University of North Carolina at Chapel Hill. He received the 2019 Gottfried E. Noether Senior Scholar Award from the American Statistical Association (ASA) and is a Fellow of the ASA, American Association for the Advancement of Science, and Institute of Mathematical Statistics.
Erica E.M. Moodie
Professor, McGill University
Erica E.M. Moodie is a professor of biostatistics and a Canada Research Chair (Tier 1) in Statistical Methods for Precision Medicine at McGill University. She is the 2020 recipient of the CRM-SSC Prize in Statistics and an elected member of the International Statistical Institute. Moodie also holds a chercheur de merite career award from the Fonds de recherche du Québec – Santé.
Stay Up-to-Date with Email Alerts
Sign up for our monthly newsletter and emails about other topics of your choosing.