The Perils of Automated Facial Recognition
Unmasking AI: My Mission to Protect What is Human in a World of Machines. By Joy Buolamwini. Random House, New York, NY, October 2023. 336 pages, $28.99.
Your Face Belongs to Us: A Secretive Startup’s Quest to End Privacy as We Know It. By Kashmir Hill. Random House, New York, NY, September 2023. 352 pages, $28.99.
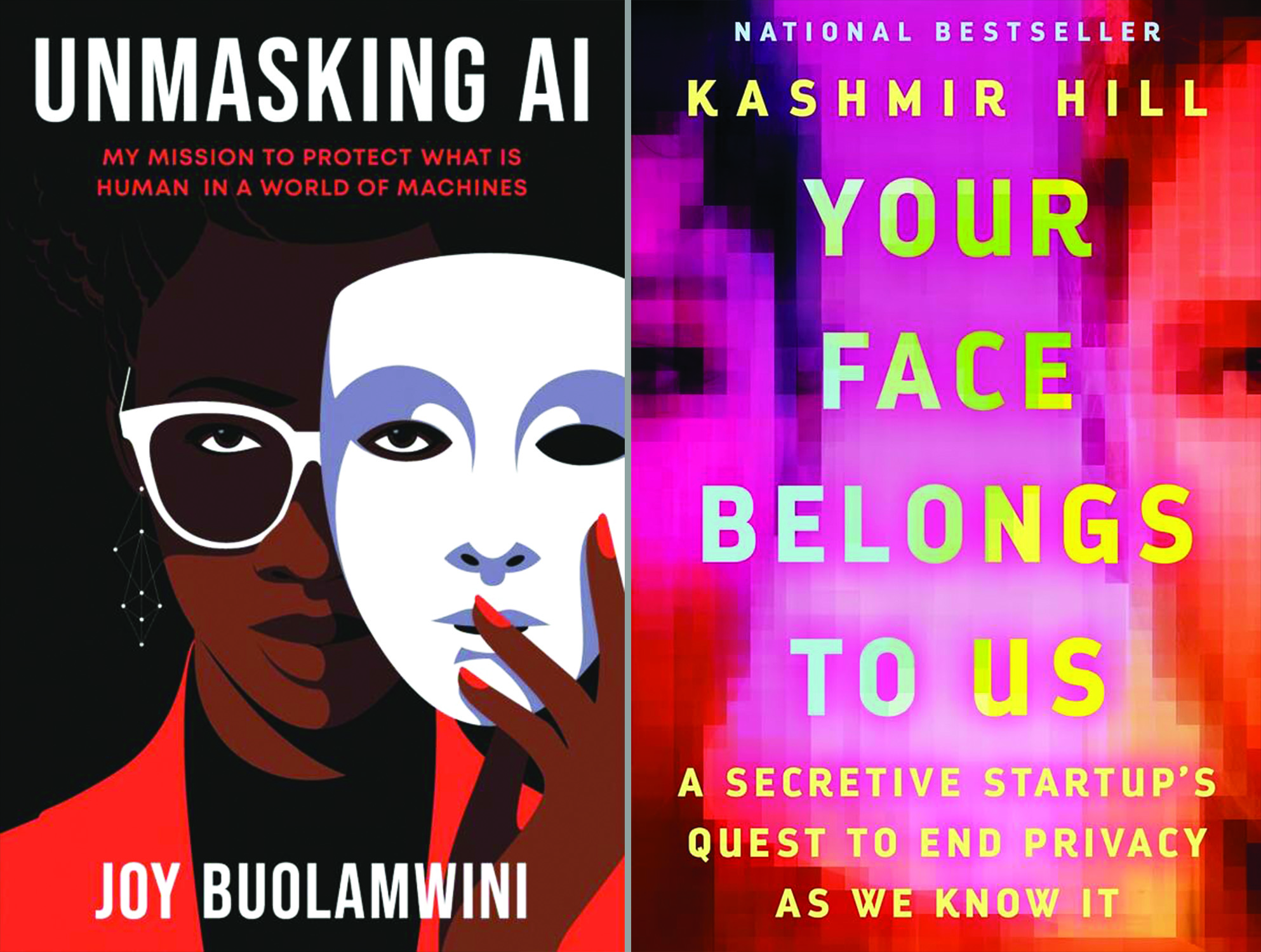
Automated facial recognition is one of the most widely deployed and technically successful forms of artificial intelligence (AI). AI systems can match faces with roughly the same level of accuracy as humans themselves. These technologies can find photos on the internet from a decades-old party that even the subject has never seen. They are able to match low-quality images and photos where the individual in question is inconspicuously in the background, part of a large group, wearing a mask, or sporting a completely different hairstyle at a much younger age.
Facial recognition is also one of the most problematic AI technologies, with very serious implications for personal privacy and inequality. The toxic combination of power, ubiquity, invasiveness, and bias has brought forth a uniquely troubling situation. Two important recent books—Unmasking AI: My Mission to Protect What is Human in a World of Machines by computer scientist Joy Buolamwini, and Your Face Belongs to Us: A Secretive Startup’s Quest to End Privacy as We Know It by New York Times reporter Kashmir Hill—raise serious concerns about the impact of facial recognition systems and the difficulty of controlling them.
§
Joy Buolamwini is best known for exposing the fact that many common facial recognition systems are much less accurate for women and people with dark skin than for white males. Unmasking AI is simultaneously an autobiography, an explanation of her scientific work, and a statement of principles that should guide AI development.
While conducting undergraduate research with a robot that had a camera that utilized facial recognition technology, Buolamwini noticed that the robot was often unable to see her face — despite its ability to identify her white classmates. She later encountered the same issue in graduate school; though she was using more advanced facial detection software, it did not process her face until she donned a white Halloween mask. Buolamwini examined this problem systematically as part of her doctoral research and found that all types of facial recognition systems had substantially higher failure rates for women, people with dark complexions, and especially dark-complexioned women.
Buolamwini has since developed industry-wide techniques to ameliorate these biases. She currently researches the detection and correction of biases in AI systems and explores the deployment of AI in ways that promote societal justice and equity. Buolamwini’s career has been marked by meteoric success, including a doctoral degree from the MIT Media Lab at the Massachusetts Institute of Technology, various TED talks, testimony to U.S. Congress, and a group meeting with U.S. President Joe Biden — all by the age of 33. Sadly, it has also been punctuated by the kinds of slights and insults that Black women in technology encounter all too often: participants at conferences who assume she is staff, security guards who block her entrance to events where she is presenting, and so on.
One particularly interesting aspect of Unmasking AI is Buolamwini’s struggle with the ethical issues that arose in her own research. Quantitative documentation of a vision program’s bias against women and people who are Black requires a benchmark collection of facial photographs that are tagged with their race and gender. Existing benchmarks’ biases towards white male faces rendered them unusable. In order to create an unbiased, high-quality assembly of benchmark photos, Buolamwini decided to personally collect the images and tag them herself. She assigned each face a numerical measure of skin color and gender, acknowledging that neither measure is fully objective and both are sometimes difficult to judge from a photograph.
Image collection presented its own set of complications. Buolamwini used photographs of global parliamentarians from official websites to avoid copyright issues and ensure that the subjects had consented to publication. Nevertheless, some concerns remained. For instance, there was no reason to suppose that, when agreeing to circulate their likenesses, the image subjects had also intended to give permission for the use of their photos in this context. In addition, a collection of parliamentarians is obviously not a representative sample in areas like age, social status, and image quality.
Finally, Buolamwini wondered about the overall impact of her own work; to what extent would it make the world better, fairer, and more equitable, and to what extent was it merely improving the technology that served as a tool for surveillance capitalism?
Although Buolamwini’s research—and that of the scientists who have followed in her footsteps—has led to significant reductions in gender and racial bias in facial recognition programs, the problem still persists. A recent New Yorker article discussed the wrongful arrest of Alonzo Sawyer in 2022 based purely on a match by AI software, despite a wealth of contrary evidence [3]. Racial and gender bias also infects other kinds of AI software, such as image generation programs. For example, when the authors of a Washington Post article entered the prompt “attractive people” into the popular Stable Diffusion model, it produced images of young, light-skinned individuals [4].
§
On January 18, 2020, the front page of The New York Times featured an extraordinary story about a completely unknown company called Clearview AI [2]. Clearview had downloaded billions of photos from the web and built an app that matched an input image against its collection with startling scope and accuracy. When Kashmir Hill, the author of the article, submitted her own photograph, the app returned “numerous results, dating back a decade, including photos of myself that I had never seen before.” The Clearview app creators sold their product—without any public notification, scrutiny, or independent evaluation of its error rate or biases—to more than 600 different law enforcement agencies and a handful of companies. The police departments that purchased it were very enthusiastic and had already used the technology to identify perpetrators and victims in cases of murder, assault, sexual abuse, and theft. Hill’s 2023 book, Your Face Belongs to Us, expands upon her original article, details her investigation, offers additional information about the history of Clearview and its founder Hoan Ton-That, and provides readers with updates to the narrative.
The prologue of the book, which recounts the first stages of Hill’s investigation, is particularly fascinating. When Hill learned about the existence of Clearview, the organization was wrapped in secrecy — despite the fact that it was already aggressively promoting the app to police departments. The scant company website listed a nonexistent New York address. When Hill called or emailed police departments, they subsequently avoided all communication. She hired a private investigator who contacted Clearview while posing as a potential customer; when he tried to test their product with Hill’s photo, they immediately severed the connection.
One particularly notable characteristic of Clearview is the comparatively fly-by-night way in which it came about. In recent years, impactful AI products have mostly originated in large corporate labs with huge teams of top-notch scientists, enormous budgets, and a plethora of computing resources. By contrast, Ton-That seemingly built, deployed, marketed, and maintained Clearview largely by himself (though he was joined by then-computational physicist Terence Liu for a few months). The product’s code used a combination of open-source software and techniques from the published literature; there is no indication that its construction involved any particular technical innovation.
When I first read Hill’s New York Times article, I assumed that it would mark the end of Clearview, much like how John Carreyou’s exposé of Theranos in The Wall Street Journal ultimately led to that company’s downfall [1]. In fact, the outcome was quite the contrary. The article’s publicity brought Clearview even more customers, though it did generate a certain amount of legal trouble for the organization. The American Civil Liberties Union (ACLU) filed a lawsuit against Clearview, but their argument was based on the narrow legal grounds that Clearview’s use of biometric measurements was illegal; the ACLU agreed with Clearview’s lawyer that simply scraping images from the web, matching them, and distributing them was protected free speech. The two groups eventually settled on the compromise that Clearview would not sell its app to private individuals or companies within the U.S., but it could continue selling to U.S. government agencies — including police departments.
§
What will the future bring? Buolamwini’s book is not very hopeful in that regard, and Hill’s text is downright depressing. Buolamwini and her colleagues at the Algorithmic Justice League (an association that she founded and runs) have an admirable mission in trying to make AI a realistic tool for human prosperity, dignity, and equity, but they face formidable headwinds as many large corporations and nations continue to develop and deploy AI systems in a seemingly reckless manner. The outlook for privacy is even worse. Facial recognition systems like Clearview are powerful and easy to use; cameras are ubiquitously deployed by the police, carried by citizens in cell phones, and hidden in household devices; and the public’s fascination with social media is apparently inexhaustible. We may soon arrive at a dystopia in which anyone can examine practically everything about someone’s life and publish it to the world whenever they choose.
Of course, unlike with climate change or pandemics, society as a whole has complete collective agency over computer technology. Given the will, nothing would stop us from eliminating all facial recognition software from our lives; doing so would not even cost much. We are in charge, not the AIs. But we must jointly identify our most important values and figure out how to protect them. Doing so is not an easy task, and we may not have much time before the situation becomes relatively dire.
References
[1] Carreyou, J. (2015, October 16). Hot startup Theranos has struggled with its blood-test technology. The Wall Street Journal. Retrieved from https://www.wsj.com/articles/theranos-has-struggled-with-blood-tests-1444881901.
[2] Hill, K. (2020, January 18). The secretive company that might end privacy as we know it. The New York Times. Retrieved from https://www.nytimes.com/2020/01/18/technology/clearview-privacy-facial-recognition.html.
[3] Press, E. (2023, November 13). Does A.I. lead police to ignore contradictory evidence? The New Yorker. Retrieved from https://www.newyorker.com/magazine/2023/11/20/does-a-i-lead-police-to-ignore-contradictory-evidence.
[4] Tiku, N., Schaul, K., & Chen, S.Y. (2023, November 1). These fake images reveal how AI amplifies our worst stereotypes. The Washington Post. Retrieved from https://www.washingtonpost.com/technology/interactive/2023/ai-generated-images-bias-racism-sexism-stereotypes.
About the Author
Ernest Davis
Professor, New York University
Ernest Davis is a professor of computer science at New York University's Courant Institute of Mathematical Sciences.
Stay Up-to-Date with Email Alerts
Sign up for our monthly newsletter and emails about other topics of your choosing.