The Shifting Nature of Health and Economic Evaluations of Disease Interventions
As the world recovers from the COVID-19 pandemic, the paradigm of multidimensional evidence generation is shifting [7]. Radical new techniques follow integrated evidence generation approaches rather than siloed methods, thereby connecting multiscale evidence that includes randomized clinical trials, patients’ medical and nonmedical histories, market penetration of interventions, population behaviors, health insurance claims, and public health surveillance. These emerging techniques follow a partnership model that comprises enhanced data engineering platforms, advanced analytical capabilities, modeling-based decision processes, and cross-functional collaborations [1].
What is Evidence and How Is It Used?
Data, information, evidence, and insights are key data science concepts that describe the process from initial data collection to ultimate knowledge. In general, data are raw symbols that are properties of observables, which then become information when described in context [2]. Evidence forms from information when one considers relevant standards of the context in order to derive insights.
The process of evidence generation involves gathering data from various sources, analyzing study design, and assessing the success of an application based on the collected data. It also entails collaborative approaches that build new information on health technologies, from the identification of data gaps to the execution and publication of scientific studies. Real-world evidence (RWE) provides valuable insight that supplements clinical trials (which are non-RWE) to help inform medical product decision-making. RWE consists of clinical evidence—collected from routine healthcare delivery—about the usage and potential risks or benefits of a medical product. It can be generated from multiple sources, such as electronic health records, medical claims, hospital billing data, insurance data, information from digital health technologies, and data from medical product and disease registries.
Studies often use multiple data sources from the literature to inform simulation models that economically evaluate a health technology. Systematic reviews and meta-analyses—the most powerful forms of scientific evidence—also help to rigorously capture reasonable uncertainty in model input. Researchers utilize statistical models that link information to combine available evidence and estimate the parameters of health economics models (see Figure 1).
Heterogeneity in Evidence Generation Across Regions
Stephen Jenkins’ 2004 book, How Science Works: Evaluating Evidence in Biology and Medicine, highlights limitations in health and ethical decision-making that stem from incomplete scientific understanding [4]. Despite updated biostatistical methods that analyze complex data with various degrees of reliability, scientists can often only achieve a limited level of consensus due to new and rapidly changing evidence from various sources. The COVID-19 pandemic intensified this problem of conflicting evidence. For example, many developing countries could not comprehensively track health burdens due to inadequate surveillance systems and inconsistent definitions of data across a variety of sources. Developed countries faced their own set of problems, including the inadequate transfer of evidence between health systems and difficulties in the generation of information about large populations. Historically, a trickle-down effect occurs wherein medical products and innovations that are created in developed countries take a long time to reach poorer nations [9].
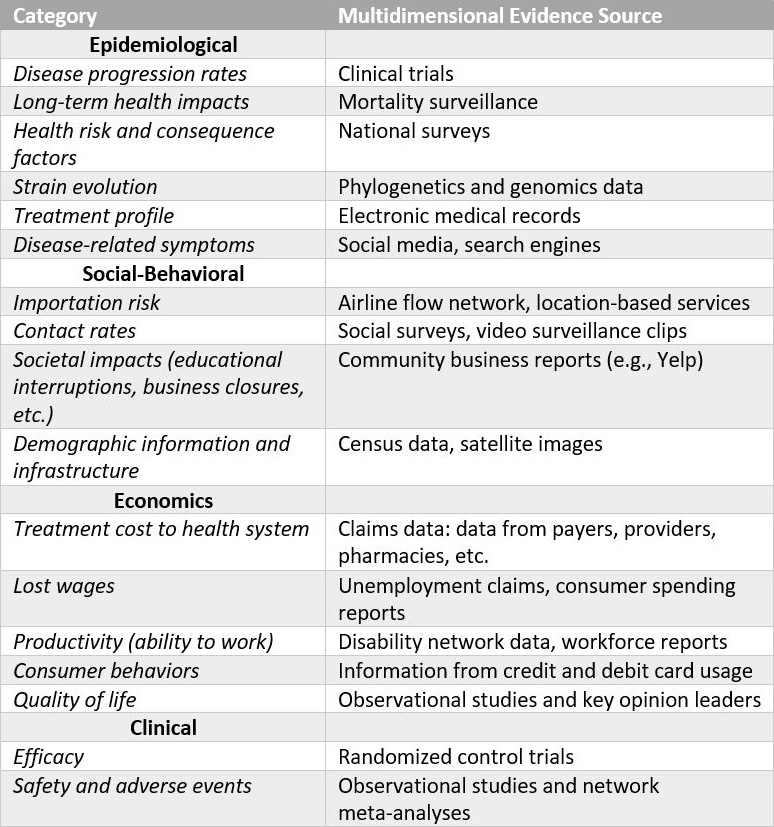
The pandemic-inspired shift in evidence generation and synthesis processes has varied drastically throughout the world. For example, the government in India conducted its COVID-19 healthcare policy design through the Kalam Institute of Health Technology, which supported the development of a complete health technology ecosystem so practitioners could conduct cutting-edge research, produce medical devices locally and on a large scale, and directly distribute these devices at the community level. These efforts helped researchers gather real-time, in-house evidence to quantify ground-level disease management.
In Who Shall Live? Health, Economics and Social Choice, author Victor Fuchs collects examples from U.S. healthcare systems to highlight the necessity of evidence-based research when striving for reliable patient-centric parameters and optimal policies in continuously evolving healthcare systems [3]. For instance, Fuchs explores state-level data from the 1960s and concludes that Nevada and Utah have vastly different life expectancies — despite their similar healthcare use patterns. Researchers later discovered that this difference in longevity is strongly related to population health behaviors and socioeconomic conditions. Through such examples, Fuchs inherently provides evidence-based reasoning that scientists must continuously replicate and reconsider their results in light of expanding data and evolving modeling methods.
Model Relevance with Available Evidence
A multitude of models simulate disease spread and evaluate the implications of health interventions (see Figure 2). These simulated outcomes play a key role in the decision-making processes for health technologies and policies. Yet while model trends are smooth, data from the field exhibits high irregularity. This disparity inspires the following questions: Is there sufficient evidence to support model assumptions, and do we have proper methods to infer information from the data? Clinicians and practitioners are sometimes skeptical of model results due to limited and/or complex unstructured evidence from both traditional and nontraditional sources. In fact, some researchers argue that better data are needed to justify estimates of risk and the subsequent measures to contain outbreaks like COVID-19.
Broader Health and Economic Impacts of Disease
In addition to patient health, the valuation of new health technologies should consider impacts such as return to livelihood and productivity level, as individual illnesses can cause ripple effects across fields and markets. This broader perspective aligns with an economic concept known as general equilibrium (as opposed to partial equilibrium, which focuses on a single market or economic sector). General equilibrium encompasses wages, costs, and benefits across multiple sectors and considers changing parameters over time as a function of an intervention’s supply and demand [5].
Novel evidence-gathering approaches that are multidimensional, integrated, and relevant to all stakeholders are pillars of the forthcoming paradigm shift. Siloed and fragmented data has hampered existing modeling in health economics and outcome research, which is primarily short term and only assesses value for select populations and markets. We hope that future health economic evaluation studies will identify evidence gaps and strategically develop cross-functional evidence-gathering processes.
Turning Evidence Challenges into Benefits
Because the state of public health and corresponding healthcare problems are continuously changing, scientists should prioritize appropriate pacing when developing modeling and data/evidence gathering tools. While immense progress has occurred in state-of-the-art surveillance and detection for many diseases (e.g., a blood test called Galleri can reportedly detect 50 different types of cancer in early stages), novel diagnosis technologies come with collateral social consequences. One must rely on multidimensional evidence—including dimensions that were traditionally overlooked—to find an effective balance and maintain healthy communities.
Significant future areas of study in the healthcare sector include strategies to reduce drug resistance, comprehensive genomic profiling for faster personalized cancer treatment, and scalable cell and gene therapies for difficult-to-treat disorders. Research in these areas progresses slowly due to the lack of evidence on drug mechanisms and healthcare resource usage, as well as the associated costs of completely redesigned approaches [6]. Despite advancements in health technology, traditional data modeling projects still involve time-consuming and repetitive manual processes. Automated machine learning (AutoML) automates the end-to-end process of model creation—including feature selection—but may not consistently outperform humans who develop models and analyze processes. By combining an analyst’s expertise with AutoML’s capabilities for model and feature selection, preprocessing, and hyper-parameter tuning, we can create a powerful solution that derives validated, valuable insights and achieves strong predictive results.
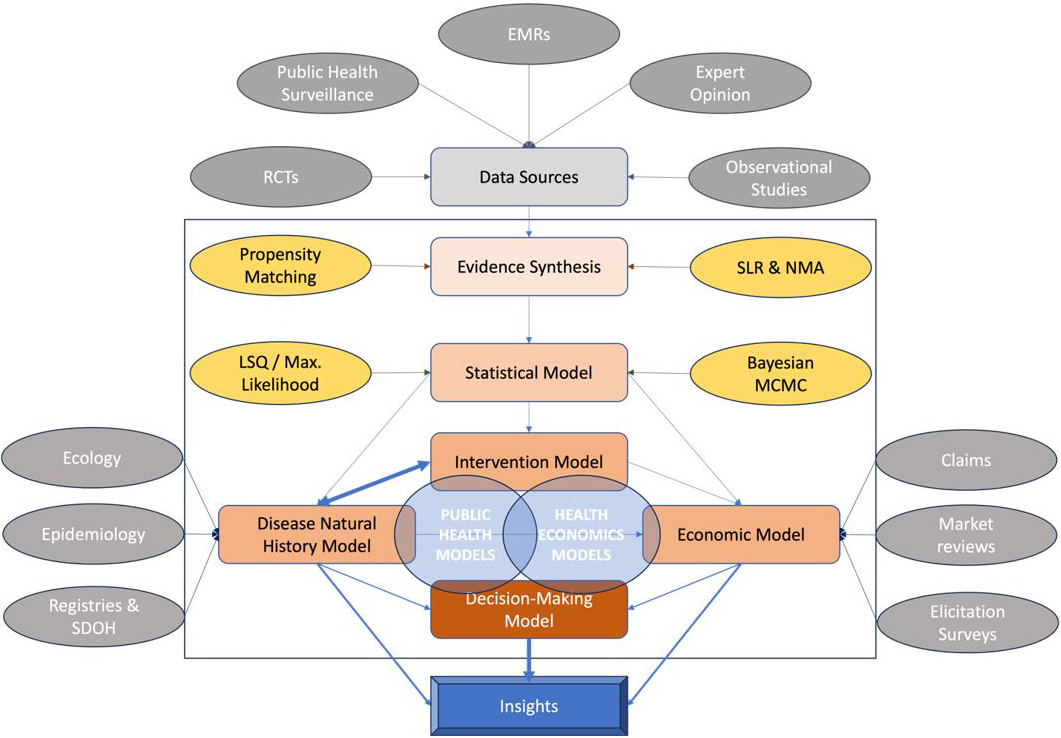
Digital traces may be useful in early warning systems for infectious disease outbreaks and studies with inadequate clinical evidence [10]. Digital traces come from internet searches on health characteristics, practitioner queries about new medical information from databases or social media, and other electronic signatures that pertain to the socioeconomic conditions of patients and caregivers. Evidence from nontraditional data streams—such as public webcam videos and location-based services—can inform dynamic models and drive real-time decision-making processes. However, the innovation of new digital technologies requires a proper data governance for the protection and dissemination of data, as well as a set of ethical principles that govern appropriate conduct for health-related activities and reduce risks of fraud, waste, and abuse.
Discussion
COVID-19 has underscored the importance of the aforementioned partnership model and continuous domain expertise when analyzing key problems in future healthcare technologies. In countries such as India which has a limited health system capacity, the delivery of health technology via digital data streams (like telemedicine and software as a service) could swiftly address problems within remote and targeted populations. Some relevant data-related challenges include the implementation of best practices for evidence collection with complete transparency and accountability, quick scaling operations despite inconsistent policies across regional boundaries, and easy importability of data from different sources.
Practitioners and policymakers should investigate additional multidimensional data from claims and reimbursements, evidence on societal disease burden, and secondary costs related to mental health [11]. Analysts can use mathematical and computational methods to enhance estimates of parameters of interest by integrating nontraditional and conventional data. It is also important to note that epidemics aggravate social issues that in turn impact disease spread [8]; for example, patients’ health conditions directly impact caregivers’ time, health, and productivity. As such, an accurate estimate of societal burden can comprehensively capture the true value of an intervention.
Integrated modeling techniques that better incorporate AutoML and social and behavioral dynamics will advance predictive accuracy and more effectively guide policy and response measures. To inform timely healthcare decision-making, we need a dynamic approach to value assessment that is characterized by living evidence synthesis and health economic evaluations — especially when the evidence base is likely to develop rapidly. Evidence synthesis should also include studies that better represent vulnerable populations and account for modeling uncertainty to fully characterize the implications of decision-making. Finally, transparency and clear communication are essential to ensuring trust in value assessment among all stakeholders. Reproducibility and reliability require the creation and maintenance of easily accessible evidence and model repositories, and we should substantially invest in data engineering infrastructures that address computational challenges. Through the support and collaboration of multiple scientific domains, healthcare systems could adopt a smooth, easily accessible pipeline of centralized multidimensional evidence that is readily integrable with multiscale dynamic modeling methods to optimize both patient and societal outcomes.
References
[1] Akman, O., Chauhan, S., Ghosh, A., Liesman, S., Michael, E., Mubayi, A., ... Tripathi, J.P. (2022). The hard lessons and shifting modeling trends of COVID-19 dynamics: Multiresolution modeling approach. Bull. Math. Biol., 84(1), 1-30.
[2] Dammann, O. (2018). Data, information, evidence, and knowledge: A proposal for health informatics and data science. Online J. Public Health Inform., 10(3), e224.
[3] Fuchs, V.R. (2011). Who shall live? Health, economics and social choice (2nd expanded ed.). Singapore: World Scientific.
[4] Jenkins, S.H. (2004). How science works: Evaluating evidence in biology and medicine. Oxford, U.K.: Oxford University Press.
[5] Keogh-Brown, M.R., Jensen, H.T., Edmunds, W.J., & Smith, R.D. (2020). The impact of Covid-19, associated behaviours and policies on the UK economy: A computable general equilibrium model. SSM Popul. Health, 12, 100651.
[6] Lee, J.H., Park, K.S., Karim, A.M., Lee, C.-R., & Lee, S.H. (2016). How to minimise antibiotic resistance. Lancet Infect. Dis., 16(1), 17-18.
[7] Mubayi, A., & Mubayi, A. (2022). Multidimensional evidence generation: A paradigm shift post-pandemic enhancing policy implementation for India’s health system. Int. J. Health Technol. Innov., 1(2), 3-6.
[8] Mubayi, A., Sullivan, J., Shafrin, J., Diaz, O., Ghosh, A., Mubayi, A., ... Veeranki, P. (2020). Battling epidemics and disparity with modeling: The coupled dynamics of the COVID-19 pandemic with social epidemics. Lett. Biomath., 7(1), 105-110.
[9] Pai, M. (2020, February 17). Global health technologies: Time to re-think the ‘trickle down’ model. Forbes. Retrieved from https://www.forbes.com/sites/madhukarpai/2020/02/17/global-health-technologies-time-to-re-think-the-trickle-down-model.
[10] Stolerman, L.M., Clemente, L., Poirier, C., Parag, K.V., Majumder, A., Masyn, S., ... & Santillana, M. (2023). Using digital traces to build prospective and real-time county-level early warning systems to anticipate COVID-19 outbreaks in the United States. Sci. Adv., 9(3), eabq0199.
[11] Yerushalmi, E., & Ziv, S. (2020). Imputing the social value of public health care: A new method with application to Israel (CAFE Working Papers 4). Birmingham, UK: Centre for Applied Finance and Economics, Birmingham City Business School, Birmingham City University. Retrieved from https://ideas.repec.org/p/akf/cafewp/4.html.
About the Authors
Anuj Mubayi
Fellow in Residence, Intercollegiate Biomathematics Alliance
Anuj Mubayi is a Fellow in Residence for the Intercollegiate Biomathematics Alliance, which is housed at Illinois State University; an honorary fellow at the Kalam Institute of Health Technology in India; an adjunct faculty member in the Department of Mathematics and Computer Science at the Sri Sathya Sai Institute of Higher Learning in India; and a scientific advisor for Kalam Experts, a healthcare consulting company. Mubayi previously served as director of the Mathematical and Theoretical Biology Institute’s Research Experiences for Undergraduates program. His expertise lies in health decision science and encompasses health economics, data-driven disease modeling, and real-world evidence generation.
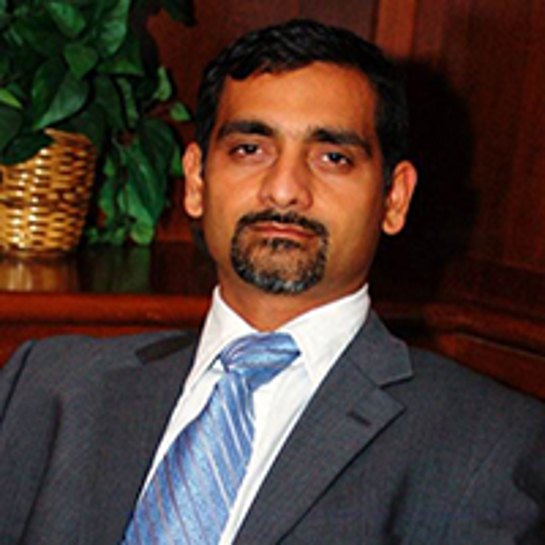
Anamika Mubayi
Associate Professor, Marwadi University
Anamika Mubayi is an associate professor in Department of Chemistry at Marwadi University in India. Her research interests are in the design, synthesis, formulation, and characterization of metal nanoparticles and nano zeolites, as well as testing and risk assessment of nanomaterials for applications in medicinal chemistry, environmental pollution, and conductive inks for printed electronics.
Olcay Akman
Professor, Illinois State University
Olcay Akman is a professor of mathematics at Illinois State University. He is director of the Intercollegiate Biomathematics Alliance: a consortium of universities that collaborate and share resources in the pursuit of scholarships, teaching, and advanced research development. Akman is currently the editor-in-chief of Letters in Biomathematics and Spora: A Journal of Biomathematics. His research interests include computing-intensive modeling, evolutionary computing, and machine-learning-based methods.
Stay Up-to-Date with Email Alerts
Sign up for our monthly newsletter and emails about other topics of your choosing.