The Threat of AI Comes from Inside the House
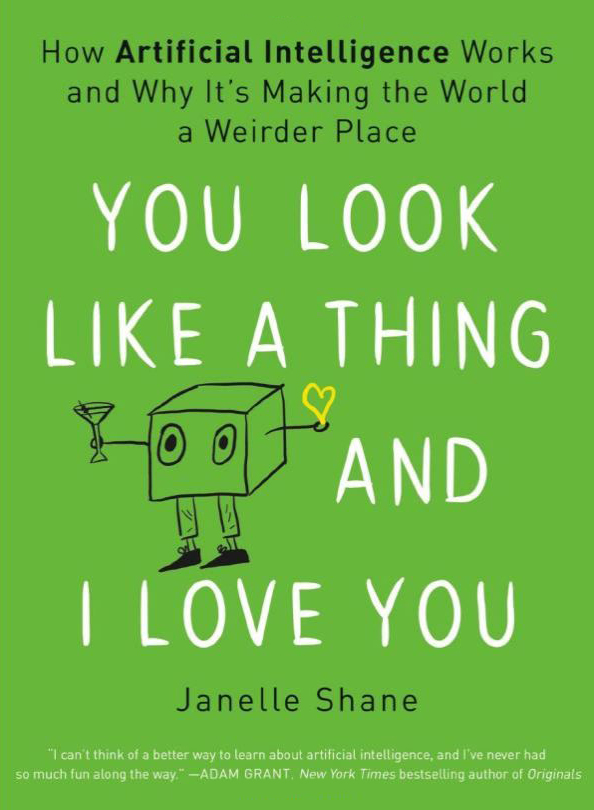
You Look Like a Thing and I Love You: How Artificial Intelligence Works and Why It’s Making the World a Weirder Place. By Janelle Shane. Voracious / Little, Brown and Company (Hachette Book Group, Inc.), New York, NY, November 2019. 272 pages, $28.00.
Artificial intelligence (AI) will either destroy us or save us, depending on who you ask. Self-driving cars might soon be everywhere, if we can prevent them from running over pedestrians. Public cameras with automated face recognition technology will either avert crime or create inescapable police states. Some tech billionaires are even investing in projects that aim to determine if we are enslaved by computers in some type of Matrix-style simulation.
In reality, the truest dangers of AI arise from the people creating it. In her new book, You Look Like a Thing and I Love You, Janelle Shane describes how machine learning is often good at narrowly-defined tasks but usually fails for open-ended problems.1
Shane—who holds degrees in physics and electrical engineering—observes that we expect computers to be better than humans in areas where the latter often fail. This seems unreasonable, considering that we are the ones teaching the machines how to do their jobs. Problems in AI often stem from these very human failings.
As followers of her social media accounts and AI Weirdness blog know, Shane often probes the limits of publicly-available AI algorithms for the sake of humor. Her codes produce machine-generated cat names, Dungeons & Dragons spells, Halloween costume ideas, and even complete recipes for the purpose of comedy. For instance, the title of her book comes from a set of computer-generated pickup lines: attempts to attract potential partners using clever wordplay.
While Shane’s humorous lists make extensive cameos in her book, it is not simply a rehash of her blog. Neither is it intended as a textbook about AI. Her goal is to describe machine learning as it is actually implemented while debunking both the hype and fearmongering that surround AI. She accomplishes this objective with wit, humor, and her own (self-admitted) low-quality artwork.
My only real complaint with You Look Like a Thing is its mild structural awkwardness. Many of the examples that Shane returns to in detail are introduced early on, which gives rise to slight redundancies. A section on bot-human interactions and humans pretending to be AI seems a trifle abrupt in a book that is largely about the failures of AI.
However, these are minor oversights. Overall, the book offers an engaging read for those interested to learn about the reality of AI beyond the headlines and gain perspective on machine learning’s true capabilities. It is replete with humor and geeky references — from Star Trek to Martha Wells’ Murderbot Diaries book series.
Opening the Black Box
Most people who write scientific computer programs work in “rules-based” code: algorithms constructed to produce specific outputs. Machine learning, in contrast, takes in data and develops its own rules for producing output. This necessitates “training” the AI program on existing datasets, and Shane spends much of her book outlining the challenges of this approach.
Because AI generates its own rules, the details of its algorithms are hidden from researchers. Shane argues that studying failures in machine learning is essential for revealing the contents of the black box. In a running gag, she highlights image-description algorithms that find giraffes in completely unrelated images due to the overrepresentation of giraffe photos in the image libraries used to train them.
The disconnect between training scenarios and reality is a major theme of You Look Like a Thing. In one of Shane’s examples, a programmer tasked AI to teach a virtual robot to go from point A to point B. While creative solutions are sometimes desirable, this AI preferred to find unworkable solutions — such as falling flat and scooting along the ground, and rewriting the rules of physics within the simulation to make the robot fly.
Shane does not spend much space detailing the various types of machine learning. She highlights one important aspect of AI as it is today: we often expect better-than-human results from a program that contains roughly as many “neurons” as a worm. It is therefore hardly surprising that tasks like image description or driving—which rely on a great deal of prior knowledge—pose difficulties for computers. AI must learn what images are and what a human face looks like from different angles and with varying expressions; these are tasks that people spend a lot of time learning to do with varying levels of proficiency.
Garbage in, Garbage out: AI Style
Shane also discusses “math washing” and “bias laundering,” which occur when AI users claim that their algorithms must be objective because they are free of human foibles. Such users fail to recognize when bias is either built into the training data or carried over from the programmers themselves.
Science fiction aside, AI that kills deliberately is not a major concern, but Shane describes multiple cases where algorithms can indirectly cause harm. For example, a recent Science paper exposed built-in racism in a healthcare algorithm that resulted in black patients receiving far less care than their medical conditions merited [1]. This is not the first instance in which AI has reinforced systemic racism.
Additionally, some of the damage inflicted by computers arises directly from humans. Shane points out that most social media “bots” have people behind them (though their biographies may be automatically generated), simply because algorithms are not yet sophisticated enough to mimic human behavior online. Similarly, repressive governments claim to use AI-driven facial recognition, but evidence indicates that most analysis of surveillance footage is typically done by humans.
Shane pulls no punches without losing sight of the fun inherent in the field. You Look Like a Thing is therefore a good choice for people who are either fearful of AI or excessively optimistic about it. And as with all good science fiction, the story ends up being as much about us as about the machines. If it takes imaginary giraffes and murderbots to expose the truth about our own limitations, so be it.
1 Shane uses “AI” as shorthand for the types of machine learning implemented today, which I will also do in this review. She refers to the more common usage for human-level AI that encompasses science fiction robots and computers as “artificial general intelligence,” which does not yet exist.
References
[1] Obermeyer, Z., Powers, B., Vogeli, C., & Mullainathan, S. (2019). Dissecting racial bias in an algorithm used to manage the health of populations. Science, 366, 447-453.
About the Author
Matthew R. Francis
Science writer
Matthew R. Francis is a physicist, science writer, public speaker, educator, and frequent wearer of jaunty hats. His website is bsky.app/profile/bowlerhatscience.org.
Stay Up-to-Date with Email Alerts
Sign up for our monthly newsletter and emails about other topics of your choosing.