Virtual Panel Dives Into Data Science Careers in Industry and Government
As the wide-reaching relevance of data science, artificial intelligence (AI), and machine learning (ML) continues to grow, exciting new opportunities are unfolding for applied mathematicians and computational scientists across a multitude of industries. In response to the heightened demand for mathematical expertise from organizations that are actively adopting these technologies, the SIAM Industry Committee organized a virtual panel—which took place last October, just before the 2024 SIAM Conference on Mathematics of Data Science—that addressed data science careers outside of academia. Moderators William Pack of Leonardo DRS and Nessy Tania of Pfizer guided an hourlong conversation between panelists Jiahao Chen of the New York City Office of Technology and Innovation, Alyssa Cuyjet of Google, Marta D’Elia of Atomic Machines, Justina Ivanauskaite of MSD Czech Republic, and Raymond Perkins of Intuit, all of whom offered an inside view of their experiences as data scientists within various industry and government settings.
As the discussion commenced, several panelists acknowledged the importance of practical research opportunities as motivating factors in their pursuit of industry or government careers. “I really appreciated that I could use mathematics to solve real problems,” Perkins, a senior data scientist at Intuit, said. “It was more a question of finding problems that I found interesting, then using that mathematical toolset.” D’Elia explored several different avenues before landing her current role as Director of AI and Modeling and Simulation at Atomic Machines. “I tried all types of jobs,” she said. “I started in academia, spent a lot of time at the labs, and then landed in industry. Throughout this path, I got closer and closer to solving actual problems; that’s when I realized that I wanted to stay in industry.”
Certain competencies from academic training become particularly relevant in the workforce. For example, a solid background in applied mathematics and statistics can help data scientists develop creative approaches to a variety of scenarios. “Strong mathematics and scientific computing skills are so important,” D’Elia said. “Students now are so lucky because they have training in ML and data science.”
A rigorous mathematical foundation also engenders a pragmatic understanding of the most effective ways to make suitable approximations according to a project’s demands and timelines. “Applied mathematics really prepares you to tread that ground,” Chen said, noting that realistic problems generally have ill-defined domains. As Director of AI and ML at the New York City Office of Technology and Innovation, Chen collaborates with his social scientist colleagues when addressing ethical questions that cannot be solved with mathematical techniques alone.
Regardless of educational track, there are some aspects of real-world careers that researchers do not typically encounter in the classroom. Ivanauskaite, who oversees a 15-person team as a data science lead at MSD, found that her own training did not prepare her to solve distinctly human problems. “When you study math, it’s very individual,” she said. “Then you come to industry and need to constantly adjust to other people.”
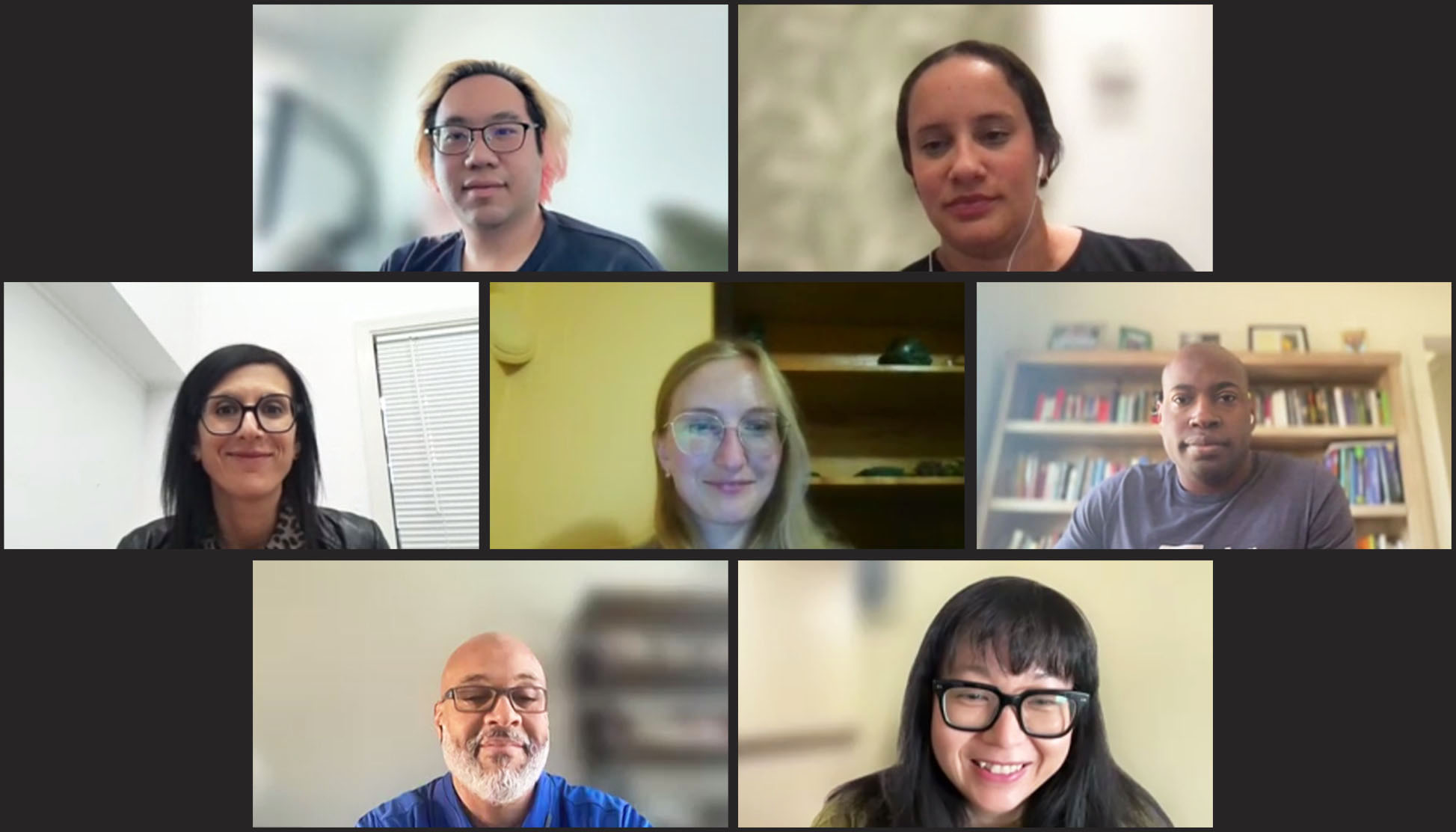
Early-career scientists will also find that they transition away from the clear-cut problems that are typically associated with academic coursework. “One of the big culture shocks for me was coming from a world where the problems were well defined,” Chen said. “In industry, I feel like 70 percent of data science is trying to find out what data I have and what I can do with it.” The panelists thus encouraged attendees to embrace a self-starter mindset; learn to evaluate the available data; and determine possible, practical courses of action. “When I started working, I realized that I could define the problem myself,” Ivanauskaite said. “I can create problems that are relevant for the business to solve.”
Maintaining focus on this practical applicability to business is crucial, as fancy mathematical techniques that do not produce useful results are irrelevant in industry. “You can have the technical solution and build something very complex and nuanced,” Cuyjet, a technical program manager at Google, said. “But if it’s not dealing with the problem at hand, it will only help you so far in the job.”
Most data science job candidates are technically proficient, so what can applied mathematicians do to set themselves apart during the application process? First, they should demonstrate an ability to clearly explain an approach, articulate the importance of different metrics, and describe a model’s risks to nontechnical colleagues. “How do you think about the problems that you’re going to solve?” Perkins asked. “If you can answer that, it will really distinguish you.” Understanding the drivers of profit, potential consumer responses, and the cascading impacts of decisions can likewise differentiate an applicant, but gaining this kind of knowledge often requires some amount of industry experience. “Where I see people struggle the most is actually having the business sense, specifically around products,” Perkins said. “Yes, you can build this type of model, but the question is, why would we want you to?”
Internships provide unique opportunities for students to familiarize themselves with an industry and gain experience with real-world problems. “If you are still in undergraduate or graduate school, I would recommend reaching out to alumni from your program,” Cuyjet said. “Ask about their experience getting into industry.” When students graduate and enter the job market, this network becomes even more crucial. Because most job postings attract a high volume of applications, a referral from a connection within the company can increase one’s chances of securing an interview.
For entry-level roles that target recent graduates, academic background and demonstrated interest in the field are especially relevant. Applicants must show that they are motivated and able to deliver what the company asks; for instance, candidates can detail their contributions to a useful project that addressed a practical issue in order to demonstrate a broader perspective beyond their technical expertise. “Be able to clearly describe the value of your work in these small projects,” Ivanauskaite said.
Educational requirements for data science jobs depend heavily on the company, location, and specific position in question. In the U.S., researchers with Ph.D.s are often offered higher salaries right out of school, though the tradeoff is that they must first spend several years in low-paying graduate programs. But this situation is not universal, and Ivanauskaite noted that a Ph.D. does not significantly accelerate one’s industry career advancement in many European countries.
Some data science roles are heavily research-driven, meaning that a Ph.D. is very beneficial (though not always strictly required) to remain competitive. However, many master’s programs now focus specifically on data science and ML and prepare students for tech-oriented positions. Perkins has observed that some Ph.D.-level scientists who enter the tech industry still need to attend bootcamps to gain certain technical skills, while other individuals with only master’s degrees possess the necessary competencies because of their programs’ concentrations. “I would begin by asking, ‘Where do you see yourself and want to be in industry?’” Perkins said. “Then work backwards to figure out what education you need.”
Overall, applied mathematicians and computational scientists have much to be excited about in the data science space. In the coming years, many opportunities will likely continue to emerge for lucrative careers across a wide range of organizations. “It’s very heartening that the field of AI and ML is a place where technically-minded people can find no shortage of interesting problems to work on,” Chen said.
About the Author
Jillian Kunze
Associate editor, SIAM News
Jillian Kunze is the associate editor of SIAM News.
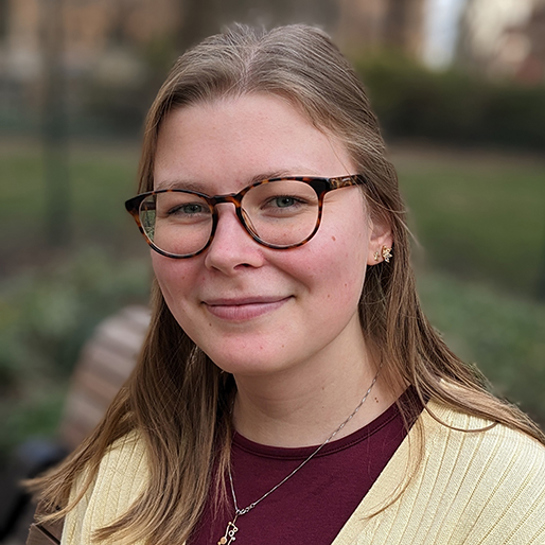
Stay Up-to-Date with Email Alerts
Sign up for our monthly newsletter and emails about other topics of your choosing.