Where are All the Guns in America? A Spatiotemporal Model of Firearm Ownership Rates
Firearm violence poses a significant threat to public health in the U.S; every day, more than 200 people sustain a nonfatal firearm injury and over 100 people are fatally injured. Despite these grim figures, surveys by the Pew Research Center reveal that 49 percent of Americans believe that gun ownership increases overall safety by allowing law-abiding citizens to protect themselves, and 67 percent of firearm owners purchased their guns for protection [4, 9]. Policymakers therefore face an exceptional challenge: how to reduce firearm-induced harm while maintaining citizens' right to bear arms.
To formulate policies that minimize firearm-related harms, legislators must understand the connection between firearm access and corresponding injuries in both their own state and other states. However, the absence of a national firearm ownership registry means that firearm prevalence data is virtually unavailable in the U.S. Although firearm research is severely underfunded and underdeveloped relative to other public health threats of comparable size, several studies have explored a few proxies that could estimate firearm prevalence. The monthly number of background checks reported by the Federal Bureau of Investigation's National Instant Criminal Background Check System is one such measure, though background checks do not always lead to gun purchases and also fail to capture illegal firearm sales. Another common measure of firearm prevalence is the Centers for Disease Control and Prevention’s report of the number of suicides that are committed with firearms each month, although the path to suicide is not always driven by firearm access.
Spatial econometric models can estimate firearm ownership in a more reliable manner. Historically, spatial econometrics emerged in the 1970s to model the urbanization of European cities [6]; researchers have since used the concept to study a wide range of socially and economically relevant topics. These types of models identify multiple geographical units (e.g., U.S. states in the case of firearm prevalence) that interact over both space and time. While temporal interactions evolve from past to future along a single axis, spatial units can evolve in multiple directions via spillover effects in a process called spatial autocorrelation.
An econometric model of firearm prevalence can incorporate proxies into a spatial Durbin model. In its simplest form, this model is expressed as follows:
\[Y=\rho WY+\beta X +\theta WX+\alpha i+\epsilon,\]
where \(Y\) is a column vector that contains the predicted firearm prevalence for each state and \(X\) is a proxy that we use to measure it. \(W\) is a spatial weight matrix that quantifies the extent to which one state interacts with another; \(i\) represents a column vector of ones; and the scalars \(\rho,\) \(\beta,\) \(\theta,\) and \(\alpha\) respectively reflect autoregressive processes, linear correlations, interactions between states, and some weight. In the absence of spatial interactions (i.e., terms that contain \(W\)), the equation reduces to an ordinary linear equation.
We can introduce a time lag into the model to account for temporal variations, as J. Paul Elhorst previously explained [5]:
\[Y_t=\tau Y_{t-1}+\rho WY_t+\eta WY_{t-1} + \beta X_t + \phi X_{t-1} + \theta WX_t + \psi WX_{t-1} + \alpha i+\epsilon.\]
In this formula, elements with subscript \(t-1\) contain observations at a time period that precedes elements with subscript \(t\). To create a causal model, we can remove nonpredictive elements (those that contain \(X_t\)) from Elhorst’s spatiotemporal econometric model and further expand it to account for multiple proxies and time resolutions:
\[Y_m=\tau Y_{m-12} + \rho WY_m + \eta WY_{m-12}+\phi^{(1)}X^{(1)}_{m-1}+\phi^{(2)}X^{(2)}_{m-1}+ \psi^{(1)}WX^{(1)}_{m-1}+\psi^{(2)}WX^{(2)}_{m-1}+\gamma d +\alpha i + \epsilon.\]
Here, superscripts of \((1)\) correspond to background checks per capita, and superscripts of \((2)\) correspond to the fraction of suicides that are committed with firearms. Subscript \(m\) denotes the month in which a measurement was made, which means that \(m-1\) denotes a measurement from the previous month and \(m-12\) denotes a measurement from the same month in the previous year. The term \(\gamma\) captures a linear time trend that might take place over multiple years.
We can calibrate the causal model with data from surveys that inquire about firearm ownership. One such survey is the Gallup Poll Social Series (GPSS), which explicitly asks respondents questions such as “Do you have a gun in your home?” and “Do you have a gun anywhere else on your property, such as in your garage, barn, shed, or in your car or truck?”. GPSS provides the highest annual resolution and has been administered more continuously than any other review, with some surveys dating back to the 1930s. Calibration with these data provides information about the values of parameters \(\tau,\) \(\rho,\) \(\eta,\) \(\phi^{(1)},\) \(\phi^{(2)},\) \(\psi^{(1)},\) \(\psi^{(2)},\) \(\gamma,\) and \(\alpha\).
Two important lessons emerge from this calibration. First, the fact that parameters \(\phi^{(1)},\) \(\phi^{(2)},\) \(\psi^{(1)},\) and \(\psi^{(2)}\) are different from zero indicates that both background checks and firearm suicides are predictive of firearm ownership — an understandable finding, since both proxies reflect different aspects of gun ownership. In fact, we could potentially improve the model’s predictions by introducing additional proxies, such as the number of hunting licenses and subscriptions to gun-related magazines. Second, the model’s spatial components—namely \(\rho,\) \(\psi^{(1)},\) and \(\psi^{(2)}\)—are also different from zero, which suggests that spillover effects take place between states when firearms are moved across borders.
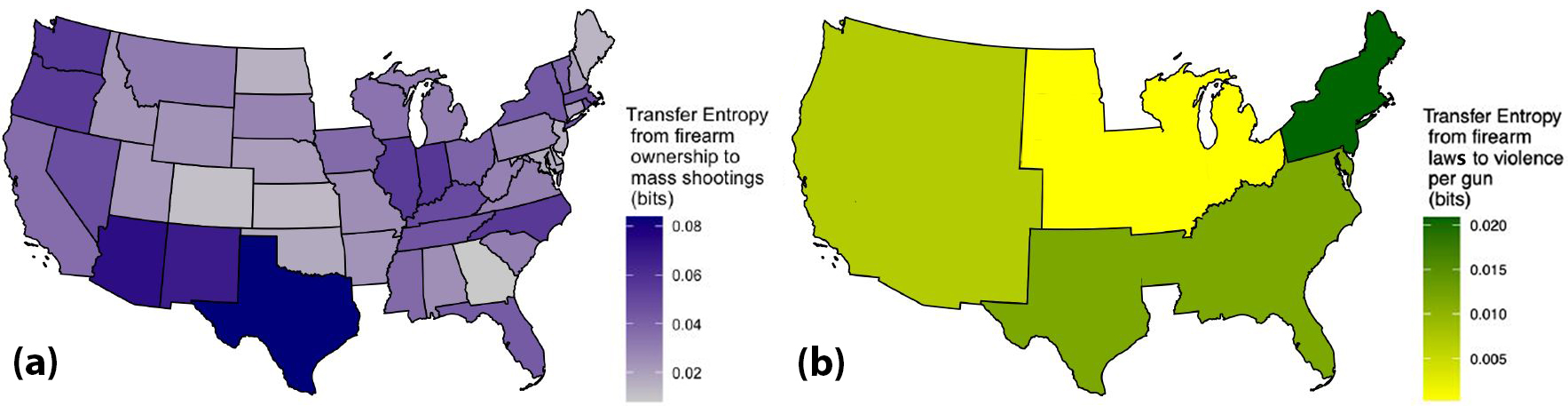
After calibrating the model’s parameters, we can utilize its output for analyses that inform firearm policy. For example, transfer entropy analysis could identify a possible causal relationship between firearm ownership and variables of firearm harm. Transfer entropy is a model-agnostic approach that infers causal relationships between pairs of variables in a Wiener-Granger sense. Mathematically, it quantifies the reduction of uncertainty when predicting the future state of one variable, given knowledge about its present state and the present state of another variable. Researchers have successfully implemented transfer entropy in a wide range of applications, including neuroscience, economics, and the study of human behavior.
Following established procedures, we can compute transfer entropy on a national level in multiple settings — from firearm ownership to mass shootings [7, 8]. For the first time, our analyses demonstrate that greater firearm ownership is causally linked to higher rates of mass shootings [2]. This result supports the notion that mass shooters can commit their crimes in part because they have access to firearms. When inspecting transfer entropy values on a state-by-state basis, it appears that this causal relationship is stronger in the western and southwestern parts of the U.S. (see Figure 1a). This outcome is unsurprising, considering that 37.5 percent of mass shootings take place in these parts of the country.
Another type of transfer entropy analysis can quantify the influence of restrictive and permissive laws on firearm safety. To do so, we create a measure of “deaths per firearm” by dividing the monthly number of firearm deaths by the estimated monthly number of firearms from the spatiotemporal econometric model [1]. We then generate a time series that records the number of restrictive and permissive laws in effect each month from the RAND State Firearm Law Database [3]. Transfer entropy between the two different time series reveals a causal link wherein an increasingly restrictive legal environment yields lower rates of “deaths per firearm.” When we compute values on a regional level, the causal link appears to be strongest in the northeastern parts of the U.S. and moderate in the midwestern and western regions (see Figure 1b). This finding is the first result to demonstrate the causal influence of firearm policies on firearm safety; it also suggests that restrictive laws allow firearm owners to bear arms while simultaneously minimizing firearm harms.
The absence of data on firearm ownership has gravely hindered firearm research in the U.S., preventing quantitative research from going beyond simple correlational analyses and thereby limiting the formulation of effective firearm policies. The field of spatial econometrics is an avenue for researchers to generate further knowledge about firearm ownership and advance this area of study. Unlike previous efforts to estimate firearm ownership, spatial econometric models account for spatially and temporally autoregressive processes. In fact, the unique federal structure of the U.S. is ideal for the study of states’ behaviors through the lens of spatial econometrics, wherein states act as individual spatial units but share language, history, politics, and culture. Depending on the availability of data, researchers could generate alternative spatial econometric models and apply them to cities, regions, divisions, or even counties. Here, we presented two analyses of the American firearm ecosystem with unprecedented results; similar analyses could inform policymakers about the determinants and consequences of firearm ownership in every state, thereby promoting the design of effective firearm legislation in the U.S.
Roni Barak Ventura delivered a minisymposium presentation on this research at the 2023 SIAM Conference on Applications of Dynamical Systems, which took place in Portland, Ore., last year.
Acknowledgments: The authors thank the National Science Foundation (award CMMI-1953135), Ministerio de Ciencia e Innovación (grant PID2019-107800GB-I00/AEI/10.13039/501100011033) and the National Collaborative on Gun Violence Research for their support. This study was also part of collaborative activities under the following programs of the region of Murcia in Spain: Groups of Excellence of the region of Murcia, Fundación Séneca, and the Science and Technology Agency (project 19884/GERM/15). The views expressed in this article are the authors’ own and do not necessarily reflect the opinions of the funding organizations.
References
[1] Barak Ventura, R., Macinko, J., Ruiz Marín, M., & Porfiri, M. (2024). Association of state firearm laws with firearm ownership and mortality. AJMP Focus, 3(4), 100250.
[2] Barak Ventura, R., Ruiz Marín, M., & Porfiri, M. (2022). A spatiotemporal model of firearm ownership in the United States. Patterns, 3(8), 100546.
[3] Cherney, S., Morral, A.R., Schell, T.L., Smucker, S., & Hoch, E. (2018). Development of the Rand State Firearm Law Database and supporting materials. Santa Monica, CA: RAND Corporation. Retrieved from https://www.rand.org/pubs/tools/TLA243-2-v2.html.
[4] Doherty, C., Kiley, J., Oliphant, B., Borelli, G., Daniller, A., Hartig, H., … Lin, K. (2023). Gun violence widely viewed as a major – and growing – national problem. Washington, D.C.: Pew Research Center. Retrieved from https://www.pewresearch.org/politics/2023/06/28/gun-violence-widely-viewed-as-a-major-and-growing-national-problem.
[5] Elhorst, J.P. (2001). Dynamic models in space and time. Geogr. Anal., 33(2), 119-140.
[6] Klaassen, L.H., & Paelinck, J.H.P. (1979). The future of large towns. Environ. Plan. A: Econ. Space, 11(10), 1095-1104.
[7] Porfiri, M., & Ruiz Marín, M. (2017). Symbolic dynamics of animal interaction. J. Theor. Biol., 435, 145-156.
[8] Porfiri, M., Sattanapalle, R.R., Nakayama, S., Macinko, J., & Sipahi, R. (2019). Media coverage and firearm acquisition in the aftermath of a mass shooting. Nat. Hum. Behav., 3, 913-921.
[9] Schaeffer, K. (2021). Key facts about Americans and guns. Washington, D.C.: Pew Research Center. Retrieved from https://www.pewresearch.org/short-reads/2021/09/13/key-facts-about-americans-and-guns.
About the Authors
Roni Barak Ventura
Postdoctoral associate, New York University
Roni Barak Ventura is a postdoctoral associate at the Center for Urban Science and Progress within the New York University (NYU) Tandon School of Engineering. She received a B.Sc. degree in biology from the University of Rochester in 2014 before joining NYU for her graduate training, where she received M.Sc. and Ph.D. degrees in biomedical engineering and mechanical engineering in 2017 and 2021, respectively. From 2021 to 2023, she served as a science analyst in the Directorate for Engineering at the National Science Foundation. Her research interests include causal inference, time series analysis, quantitative policy evaluation, and human behavior.
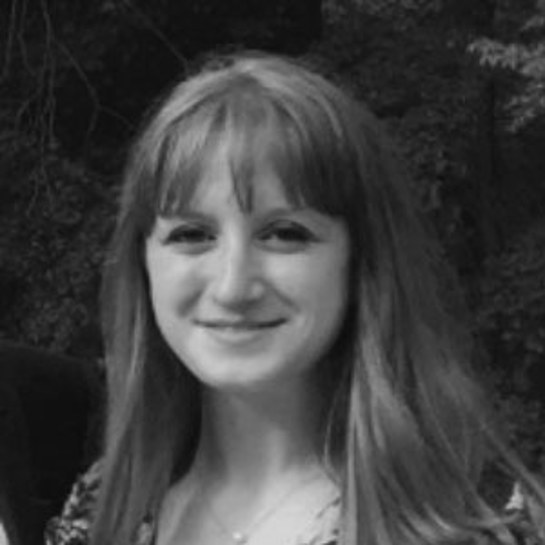
Manuel Ruíz Marín
Professor, Technical University of Cartagena
Manuel Ruíz Marín is a professor at the Technical University of Cartagena in Spain. He received his Ph.D. in mathematics in 2002 from the University of Murcia in Spain and then became a postdoctoral fellow at the Free University of Brussels. His main research interests are in spatial and time series analysis, including the study of spatial statistics/econometrics, nonparametric statistics, symbolic analysis, and complex dynamics. He has advised six Ph.D. students, contributed more than 100 scientific publications, and participated in more than 20 research projects/contracts (several as principal investigator) with national and international teams.
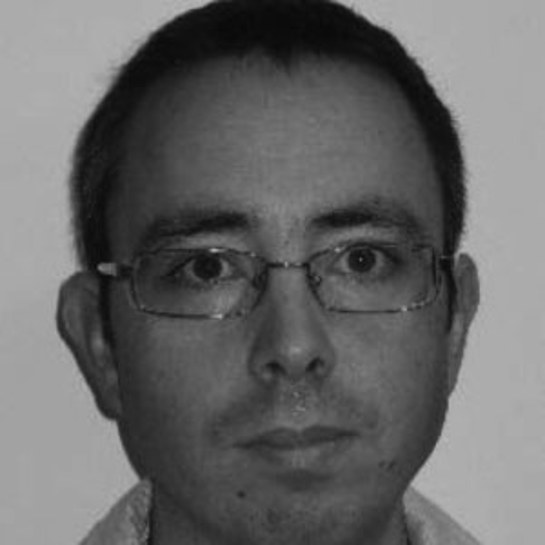
Maurizio Porfiri
Institute Professor, New York University
Maurizio Porfiri is an Institute Professor at the New York University (NYU) Tandon School of Engineering, with appointments in the Center for Urban Science and Progress, the Department of Mechanical and Aerospace Engineering, and the Department of Biomedical Engineering. He is founder and director of the Dynamical Systems Laboratory at NYU, where he conducts and supervises research on theory and interdisciplinary applications of dynamical systems.
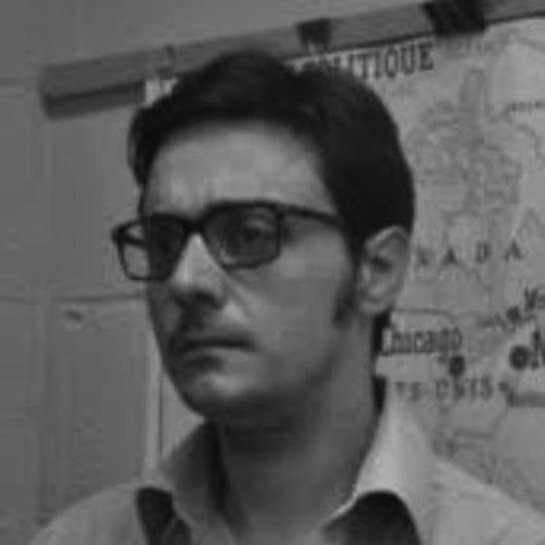
Related Reading
Stay Up-to-Date with Email Alerts
Sign up for our monthly newsletter and emails about other topics of your choosing.