Wildebeest Self-organization via Active Matter Theory
From the motion of molecules over cell membranes to the movement of sheep during sheepdog trials, collective motion across a surface poses a fascinating and difficult set of problems. Unlike flocks of birds in three dimensions or herds on flat plains, groups that are confined to arbitrary, curved, two-dimensional surfaces bring a level of geometrical complexity to the mathematical simulation of flows. The orientation of an animal or molecule is also significant; sheep have heads and tails, for instance, and biological molecules have distinct physical and often electrical polarities.
For arbitrary surfaces, the topology and—more mundanely—the topography affect self-organization in subtle ways. A recent paper in Physical Review E approached this general problem in the context of wildebeest herding on various surfaces [1]. The authors had two different goals: (i) Understand the self-organization of actin molecules on the surface of the parasite Toxoplasma gondii and (ii) scientifically recreate a famous scene from Disney’s The Lion King.
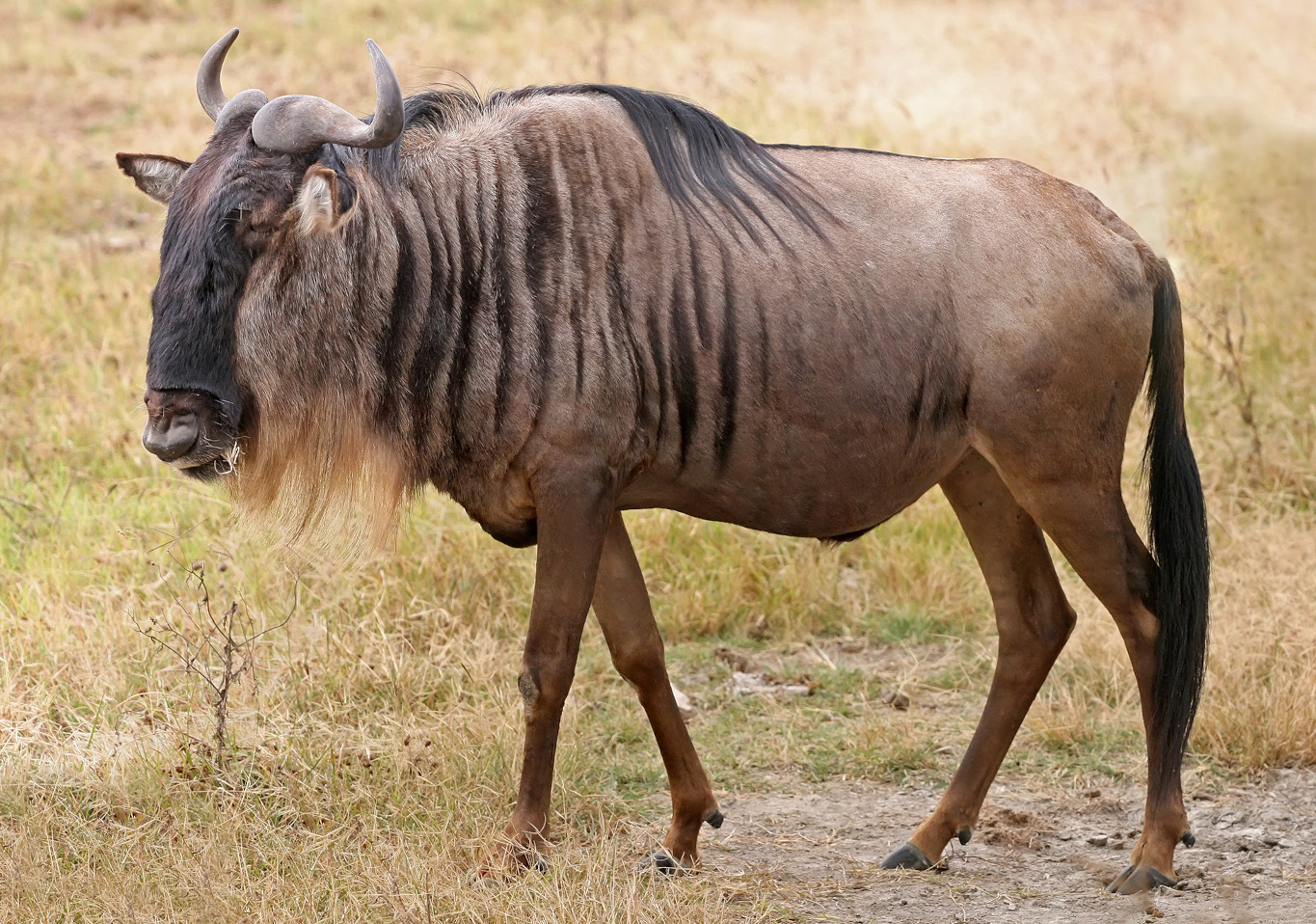
“We started with an analogous situation to pipe flow,” said Christina Hueschen, a biologist at Stanford University who authored the study with Alexander Dunn and Rob Phillips. “The channel was flat, like the canyon where Scar, the evil uncle [in The Lion King], drives the wildebeest down. We put in an obstacle and were excited to see how behaviors differ between this active system compared to a Newtonian fluid, like pipe flow past an obstacle.”
To explore this problem, Hueschen and her colleagues treated a single herd of wildebeest—also known as gnu (see Figure 1)—with a nonlinear continuum model for active matter. They drew upon a type of differential geometry and a common numerical method in engineering to extend the theory onto arbitrary curved surfaces for the first time. “We tried to make these different toolkits accessible across fields,” Hueschen said. The use of wildebeest as the primary example made the methodology both concrete and eye-catching for other researchers who might not otherwise be inclined to read an article on either subspecialty.
A Gnu Approach to Active Matter
Flocking birds and herding animals have inspired artists and naturalists since antiquity; in fact, some cave paintings might even depict herding behaviors. The theory of active matter, however, is much more recent — in the 1990s, John Toner and Yuhai Tu adapted the widely-used XY model from condensed matter physics to understand flocking and other forms of self-organization [2]. Their approach, which treats polar matter as a nonequilibrium fluid that is dominated by friction and minimal self-interaction, turned out to be extremely applicable to a wide range of phenomena in physics and biology.
The Toner-Tu active matter equations—which govern the density \(\rho(\mathbf{r},t)\) and velocity \(\mathbf{v}(\mathbf{r},t)\) fields for the wildebeest—are written as
\[\frac{\partial \mathbf{v}}{\partial t}=\beta(v^2_\textrm{pref}-v^2)\mathbf{v}-\sigma\nabla\rho+D\nabla^2\mathbf{v}-\lambda \mathbf{v}\cdot\nabla \mathbf{v},\]
with parameters \(\{\beta, \rho, D, \lambda\}\). The first term ensures that the wildebeest velocities remain close to a preferred speed \(v_\textrm{pref}\), which is set by the preferred density \(\rho_c\) and an additional parameter \(\alpha\):
\[v^2_\textrm{pref}=\alpha(\rho-\rho_c)\beta.\]
The \(\sigma\) term contains the density’s gradient and is an effective pressure that prevents wildebeest clumping, while the Laplacian term with \(D\) controls diffusion. The last piece, which is parameterized with \(\lambda\), is a Navier-Stokes advection term; it guarantees that the wildebeest carry the information about their orientation and velocity through the “fluid.” Finally, this model assumes wildebeest conservation (i.e., no animals are born or die for the duration of the simulation):
\[\frac{\partial\rho}{\partial t}+\nabla \cdot (\rho \mathbf{v})=0.\]
Real-world data from wildlife tracking efforts or microscopy inform both the choice of parameters and the demands of the simulation.
However, analytical solutions of the active matter equations are only possible for certain systems, such as flocking in an empty, geometrically flat space or on highly symmetrical curved surfaces like spheres or cylinders. Real-world surfaces of Toxoplasma cells or the Serengeti ecosystem are neither flat nor symmetrical, which limits the application of the Toner-Tu model in its original form.
Hueschen and her colleagues decided to utilize extrinsic differential geometry1 because their research involves surfaces that are embedded in three-dimensional (3D) space. This method allowed them to project the fully 3D Toner-Tu equations onto the surface of interest with a standard geometrical operator:
\[\hat{P} = I - \mathbf{n} \otimes \mathbf{n},\]
where \(I\) is the identity operator and \(\mathbf{n}\) is the normal vector at each point on the surface. When the projection operator acts on the velocity vector, it only selects the components that are tangent to the surface:
\[\hat{P}(\mathbf{v})=\mathbf{v}-\mathbf{n}(\mathbf{n}\cdot \mathbf{v})=\mathbf{v}^{\|}.\]
Extrinsic differential geometry also defines the curved-surface versions of the Toner-Tu model’s gradient, Laplacian, and advection terms in a standard way. The projected gradient of the density is relatively simple:
\[\nabla_{\Gamma} \rho=\hat{P}(\nabla \rho)=\nabla \rho-\mathbf{n}(\mathbf{n} \cdot \nabla\rho),\]
where \(\nabla_\Gamma\) denotes the gradient operator that is projected onto the surface. The advection and Laplacian terms are more complicated because they require projection of the velocity vectors and differential operators. For instance, the advection term is most easily expressed in matrix form—using the Einstein summation convention over repeated indices—with Cartesian coordinates:
\[[\nabla_\Gamma \mathbf{v}^{\|}]_{ij}=[\hat{P}(\nabla \mathbf{v}^{\|})\hat{P}]_{ij}=(\delta_{ik}-n_i n_k)\frac{\partial v_k^{\|}}{\partial x_m}(\delta_{mj}-n_mn_j).\]
To further simplify the simulation, the authors employed the finite element method (FEM) to divide the surface into a set of (flat) triangles with a normal vector at each vertex — a common technique in a wide range of engineering and computer graphics applications. “We wanted to use commonly available numerical tools, and the finite element approach allows one to solve any [partial differential equation] at the nodes and then interpolate between,” Hueschen said. In other words, formulating the Toner-Tu model with extrinsic differential geometry and FEM turns an analytically intractable problem into one that everyday commercial software can feasibly handle.
All This and Toner-Tu
Hueschen and her collaborators compared their FEM-based numerical algorithms with other scientists’ analytical solutions to the Toner-Tu equations for specific geometries, including flat surfaces, cylinders, and spheres. For their Lion King simulation, the authors utilized a “racetrack”: a flat, rectangular surface with rigid sides and periodic boundary conditions at the ends (analogous to a two-dimensional “pipe”), which matches known active matter solutions. They also demonstrated that their numerical simulations match the analytical results for symmetrical surfaces, including spheres.
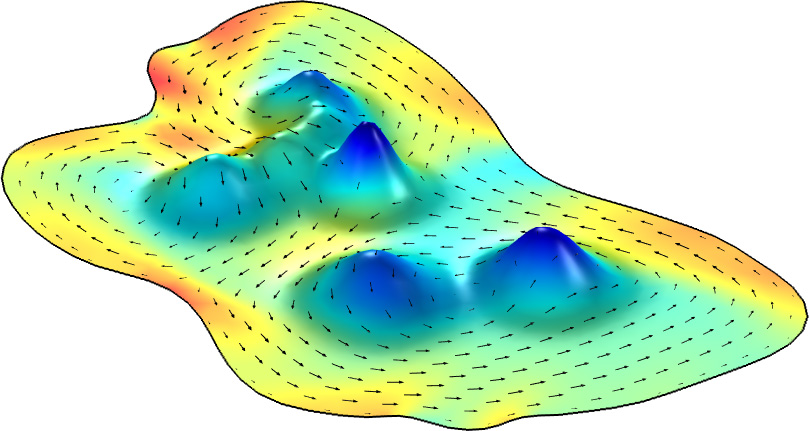
With the comparison to established solutions in hand, the researchers then added a single hill to the racetrack to identify potential changes in wildebeest herding behavior. Real herds “scatter” off obstacles by increasing their density on either side of the obstruction and thinning out over hills, or avoiding overly steep inclines entirely (see Figure 2). Although the basic Toner-Tu model does not include gravity—since gravity is not relevant to microscopic examples like molecular self-organization on cell surfaces—the team created a modified version of the equations to represent the way in which herds might account for gravity when encountering hills in the terrain.
But the real power of the advanced Toner-Tu model lies in its versatility. “We reformulated these Toner-Tu flocking equations into a general curved surface form that is solvable as long as one has any surface where the normal vectors are defined,” Hueschen said. “You don’t need a parameterization or coordinate system. In two minutes, you can plug in a new geometry and resolve the equations on the hilly Serengeti or a weird banana shape.”
The need to define normal vectors does somewhat limit the methodology, as it cannot handle especially complicated surfaces without some initial smoothing. Yet despite the current focus on the wildebeest example, the toolkit is still quite powerful and general. This flexibility is important because the researchers were primarily interested in actin molecules’ ability to self-organize on the very complex surfaces of Toxoplasma cells — knowledge that is potentially useful when modeling the way in which these parasites infect mammal brains. Other examples of biological self-organization include cell motion on the surface of embryos and cytoskeleton patterns that give cells their shapes, neither of which exhibit strong geometrical symmetries.
Despite the complicated mathematics, Hueschen emphasized how much fun she and her colleagues had while working on this problem. “There was sort of an element of joy the whole time,” she said. Delight is never a bad thing when exploring new (or gnu) methodologies.
1 As opposed to the intrinsic differential geometry of manifolds, which defines arbitrary curved surfaces or other spaces without embedding them in a higher-dimensional space.
References
[1] Hueschen, C.L., Dunn, A.R., & Phillips, R. (2023). Wildebeest herds on rolling hills: Flocking on arbitrary curved surfaces. Phys. Rev. E, 108, 024610.
[2] Toner, J., & Tu, Y. (1995). Long-range order in a two-dimensional dynamical XY model: How birds fly together. Phys. Rev. Lett., 75(23), 4326.
About the Author
Matthew R. Francis
Freelance science writer
Matthew R. Francis is a physicist, science writer, public speaker, educator, and frequent wearer of jaunty hats. His website is BowlerHatScience.org.
Stay Up-to-Date with Email Alerts
Sign up for our monthly newsletter and emails about other topics of your choosing.